Generative machine learning-based multi-objective process parameter optimization towards energy and quality of injection molding
Environmental science and pollution research international(2023)
摘要
The high energy intensity and rigorous quality demand of injection molding have received significant interest under the background of the soaring production of global plastic industry. As multiple parts can be produced in a multi-cavity mold during one operation cycle, the weight differences of these parts have been demonstrated to reflect their quality performance. In this regard, this study incorporated this fact and developed a generative machine learning-based multi-objective optimization model. Such model can predict the qualification of parts produced under different processing variables and further optimize processing variables of injection molding for minimal energy consumption and weight difference amongst parts in one cycle. Statistical assessment via F 1 -score and R 2 was performed to evaluate the performance of the algorithm. In addition, to validate the effectiveness of our model, we conducted physical experiments to measure the energy profile and weight difference under varying parameter settings. Permutation-based mean square error reduction was adopted to specify the importance of parameters affecting energy consumption and quality of injection molded parts. Optimization results indicated that the processing parameters optimization could reduce ~ 8% energy consumption and ~ 2% weight difference compared with the average operation practices. Maximum speed and first-stage speed were identified as the dominating factors affecting quality performance and energy consumption, respectively. This study could contribute to the quality assurance of injection molded parts and facilitate energy efficient and sustainable plastic manufacturing.
更多查看译文
关键词
Energy consumption,Injection molding,Multi-objective optimization,Product quality,Random forest,Sustainable manufacturing
AI 理解论文
溯源树
样例
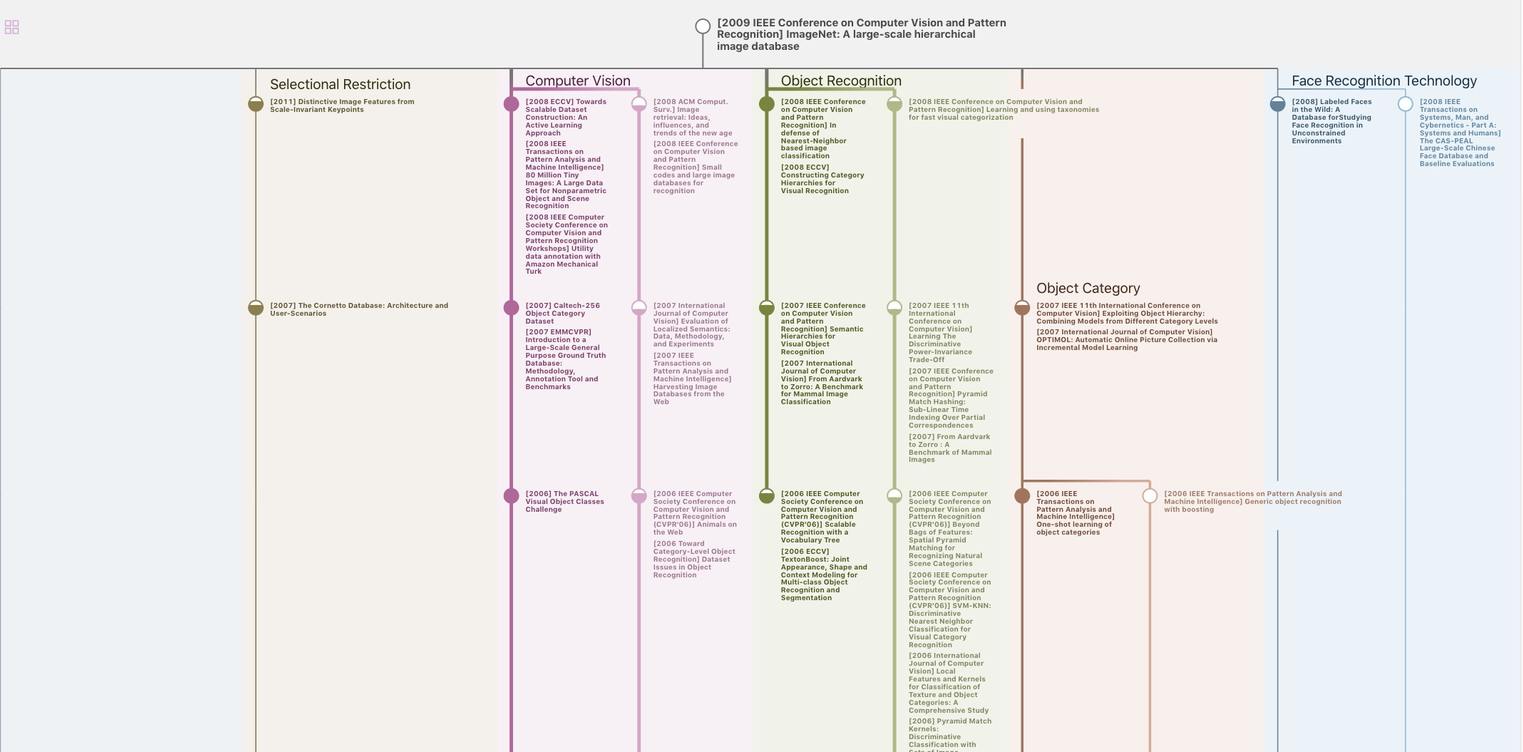
生成溯源树,研究论文发展脉络
Chat Paper
正在生成论文摘要