Graph embedding and Gaussian mixture variational autoencoder network for end-to-end analysis of single-cell RNA sequencing data
CELL REPORTS METHODS(2023)
摘要
Single-cell RNA sequencing (scRNA-seq) is a revolutionary technology to determine the precise gene expression of individual cells and identify cell heterogeneity and subpopulations. However, technical limitations of scRNA-seq lead to heterogeneous and sparse data. Here, we present autoCell, a deep-learning approach for scRNA-seq dropout imputation and feature extraction. autoCell is a variational autoencoding network that combines graph embedding and a probabilistic depth Gaussian mixture model to infer the distribution of high-dimensional, sparse scRNA-seq data. We validate autoCell on simulated datasets and biologically relevant scRNA-seq. We show that interpolation of autoCell improves the performance of existing tools in identifying cell developmental trajectories of human preimplantation embryos. We identify disease-associated astrocytes (DAAs) and reconstruct DAA-specific molecular networks and ligand-receptor interactions involved in cell-cell communications using Alzheimer's disease as a prototypical example. autoCell provides a toolbox for end-to-end analysis of scRNA-seq data, including visualization, clustering, imputation, and disease-specific gene network identification.
更多查看译文
关键词
Alzheimer’s disease,deep learning,disease-associated astrocyte,scRNA-seq,single cell/nuclei,variational autoencoding network
AI 理解论文
溯源树
样例
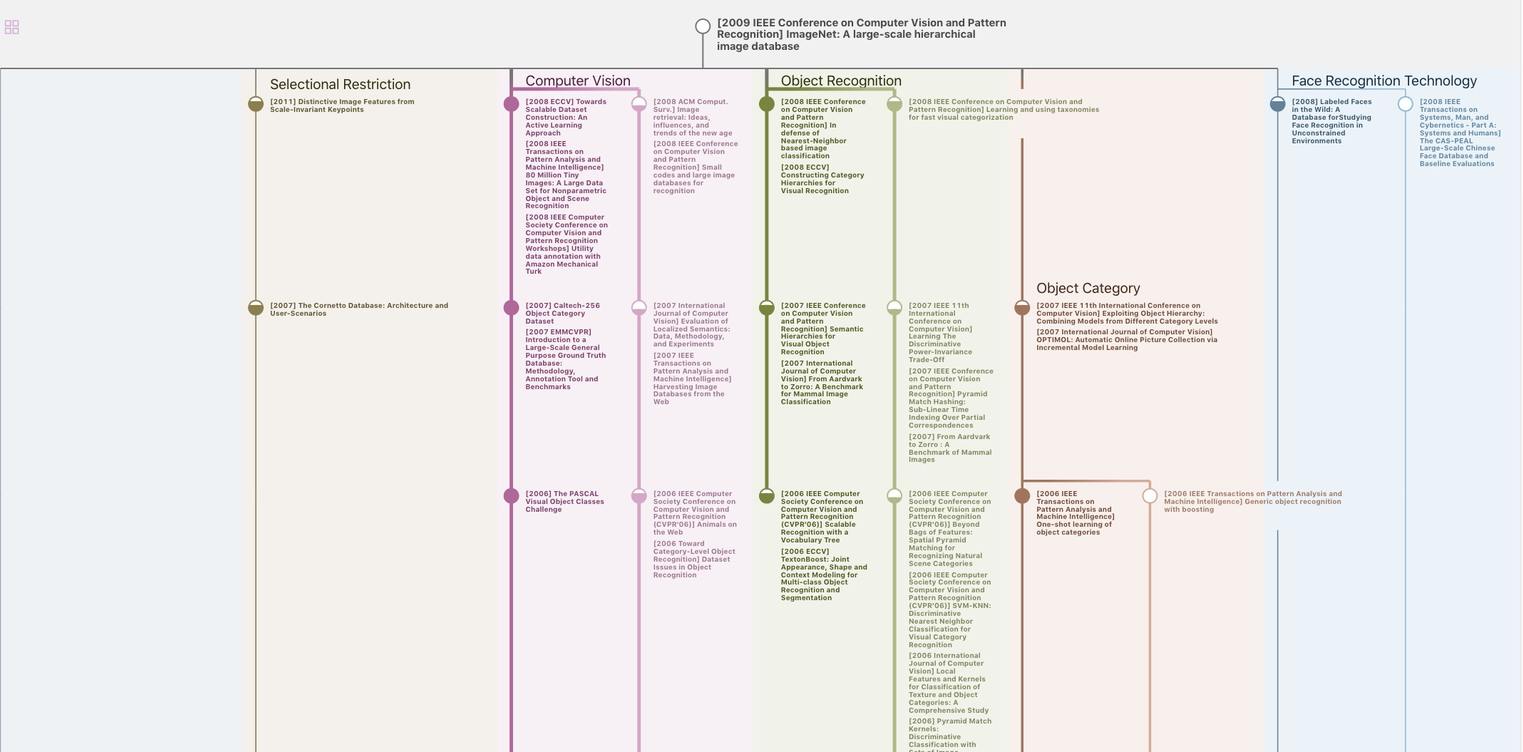
生成溯源树,研究论文发展脉络
Chat Paper
正在生成论文摘要