Sparse Component Analysis (SCA) Based on Adaptive Time-Frequency Thresholding for Underdetermined Blind Source Separation (UBSS).
Sensors(2023)
摘要
Blind source separation (BSS) recovers source signals from observations without knowing the mixing process or source signals. Underdetermined blind source separation (UBSS) occurs when there are fewer mixes than source signals. Sparse component analysis (SCA) is a general UBSS solution that benefits from sparse source signals which consists of (1) mixing matrix estimation and (2) source recovery estimation. The first stage of SCA is crucial, as it will have an impact on the recovery of the source. Single-source points (SSPs) were detected and clustered during the process of mixing matrix estimation. Adaptive time-frequency thresholding (ATFT) was introduced to increase the accuracy of the mixing matrix estimations. ATFT only used significant TF coefficients to detect the SSPs. After identifying the SSPs, hierarchical clustering approximates the mixing matrix. The second stage of SCA estimated the source recovery using least squares methods. The mixing matrix and source recovery estimations were evaluated using the error rate and mean squared error (MSE) metrics. The experimental results on four bioacoustics signals using ATFT demonstrated that the proposed technique outperformed the baseline method, Zhen's method, and three state-of-the-art methods over a wide range of signal-to-noise ratio (SNR) ranges while consuming less time.
更多查看译文
关键词
mixing matrix estimation,sparse component analysis,underdetermined blind source separation
AI 理解论文
溯源树
样例
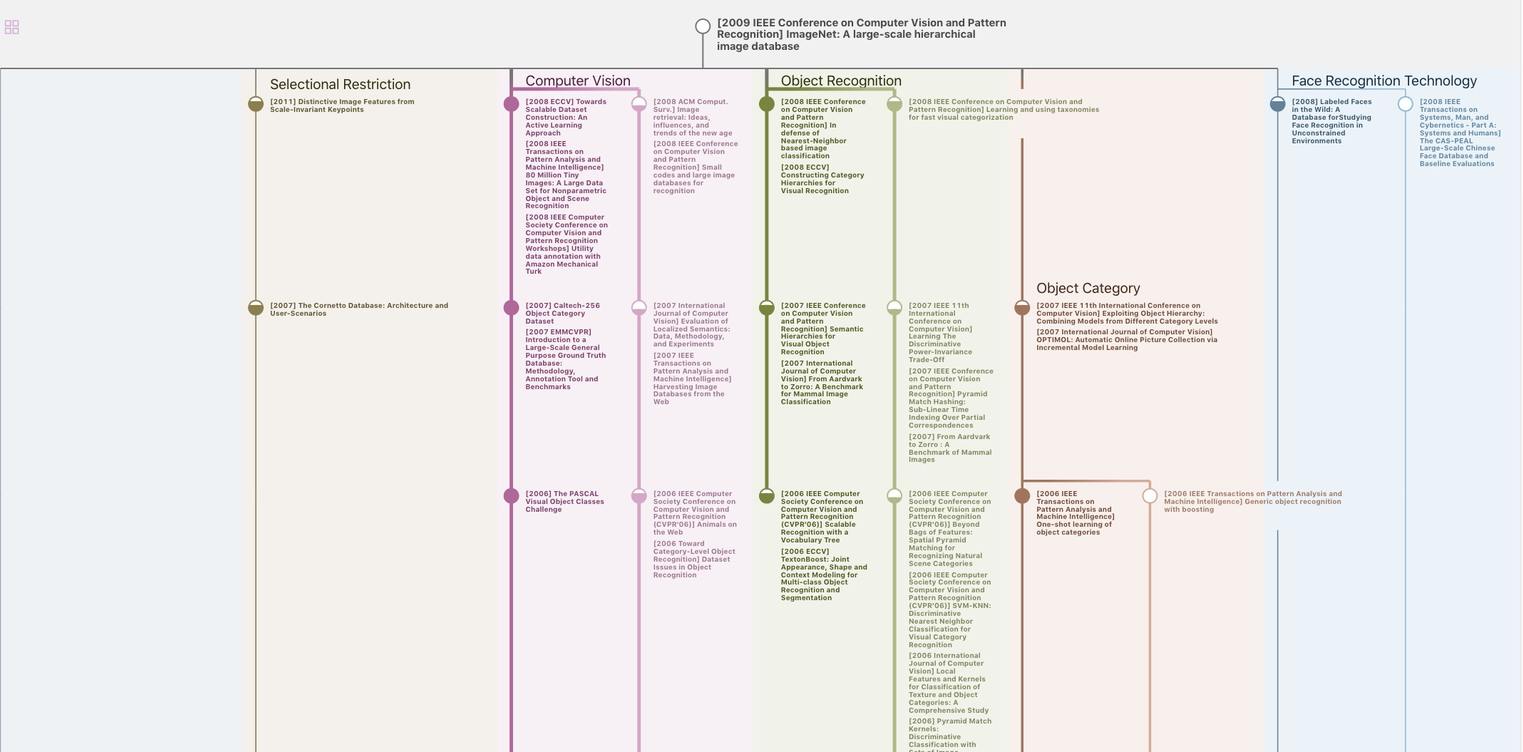
生成溯源树,研究论文发展脉络
Chat Paper
正在生成论文摘要