Robust and Secure Quality Monitoring for Welding Through Platform-as-a-Service: A Resistance and Submerged Arc Welding Study
Machines(2023)
摘要
For smart manufacturing systems, quality monitoring of welding has already started to shift from empirical modeling to knowledge integration directly from the captured data by utilizing one of the most promising Industry 4.0’s key enabling technologies, artificial intelligence (AI)/machine learning (ML). However, beyond the advantages that they bring, AI/ML introduces new types of security threats, which are related to their very nature and eventually, they will pose real threats to the production cost and quality of products. These types of security threats, such as adversarial attacks, are causing the targeted AI system to produce incorrect or malicious outputs. This may undermine the performance (and the efficiency) of the quality monitoring systems. Herein, a software platform servicing quality monitoring for welding is presented in the context of resistance and submerged arc welding. The hosted ML classification models that are trained to perform quality monitoring are subjected to two different types of untargeted, black-box, adversarial attacks. The first one is based on a purely statistical approach and the second one is based on process knowledge for crafting these adversarial inputs that can compromise the models’ accuracy. Finally, the mechanisms upon which these adversarial attacks are inflicting damage are discussed to identify which process features or defects are replicated. This way, a roadmap is sketched toward testing the vulnerability and robustness of an AI-based quality monitoring system.
更多查看译文
关键词
smart manufacturing,welding,quality monitoring,adversarial attacks,machine learning
AI 理解论文
溯源树
样例
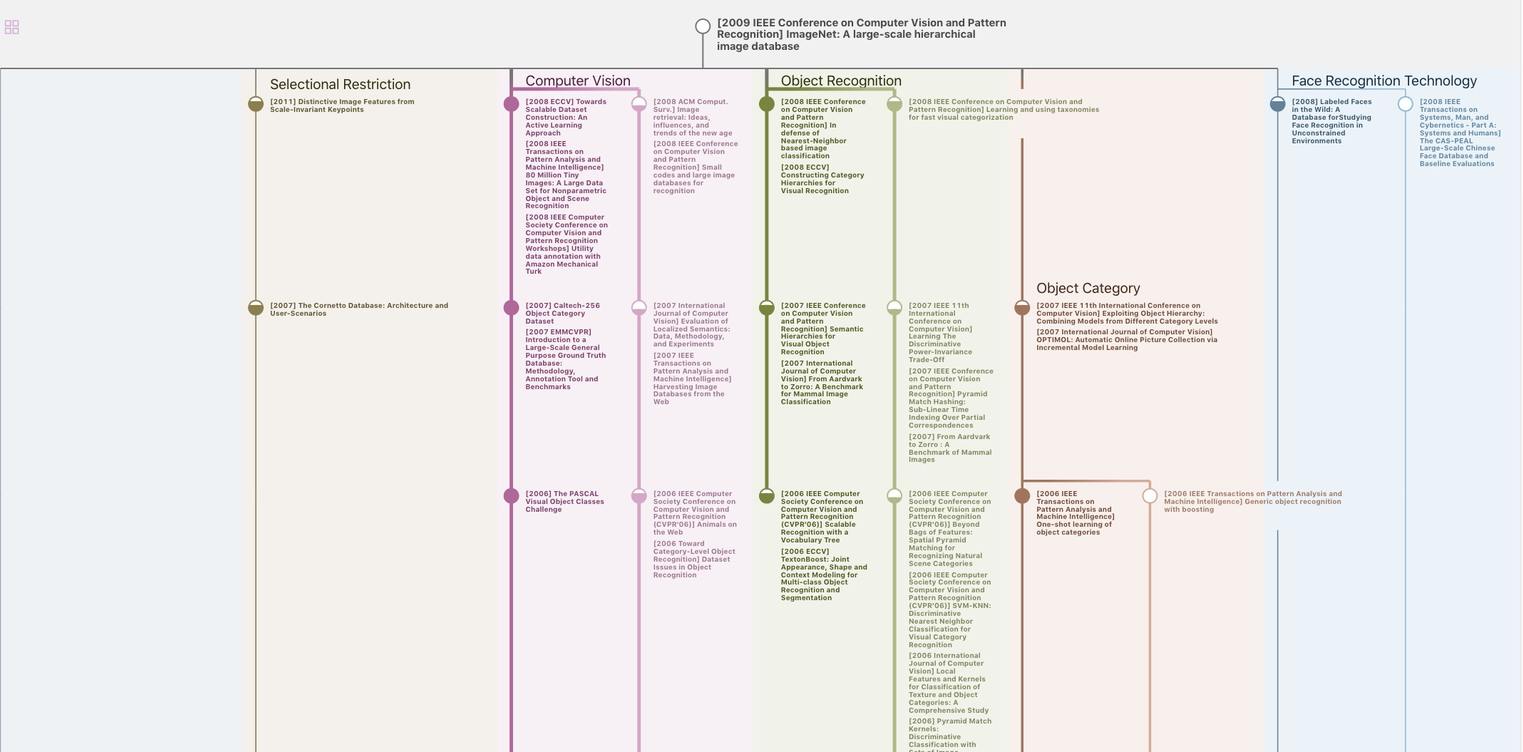
生成溯源树,研究论文发展脉络
Chat Paper
正在生成论文摘要