Learning Based Spatial Power Characterization and Full-Chip Power Estimation for Commercial TPUs
2023 28th Asia and South Pacific Design Automation Conference (ASP-DAC)(2023)
摘要
In this paper, we propose a novel approach for the real-time estimation of chip-level spatial power maps for commercial Google Coral M.2 TPU chips based on a machine-learning technique for the first time. The new method can enable the development of more robust runtime power and thermal control schemes to take advantage of spatial power information such as hot spots that are otherwise not available. Different from the existing commercial multi-core processors in which real-time performance-related utilization information is available, the TPU from Google does not have such information. To mitigate this problem, we propose to use features that are related to the workloads of running different deep neural networks (DNN) such as the hyperparameters of DNN and TPU resource information generated by the TPU compiler. The new approach involves the offline acquisition of accurate spatial and temporal temperature maps captured from an external infrared thermal imaging camera under nominal working conditions of a chip. To build the dynamic power density map model, we apply generative adversarial networks (GAN) based on the workload-related features. Our study shows that the estimated total powers match the manufacturer's total power measurements extremely well. Experimental results further show that the predictions of power maps are quite accurate, with the RMSE of only 4.98mW/mm
2
, or 2.6% of the full-scale error. The speed of deploying the proposed approach on an Intel Core i7-10710U is as fast as 6.9ms, which is suitable for real-time estimation.
更多查看译文
关键词
based spatial power characterization,commercial tpus,full-chip
AI 理解论文
溯源树
样例
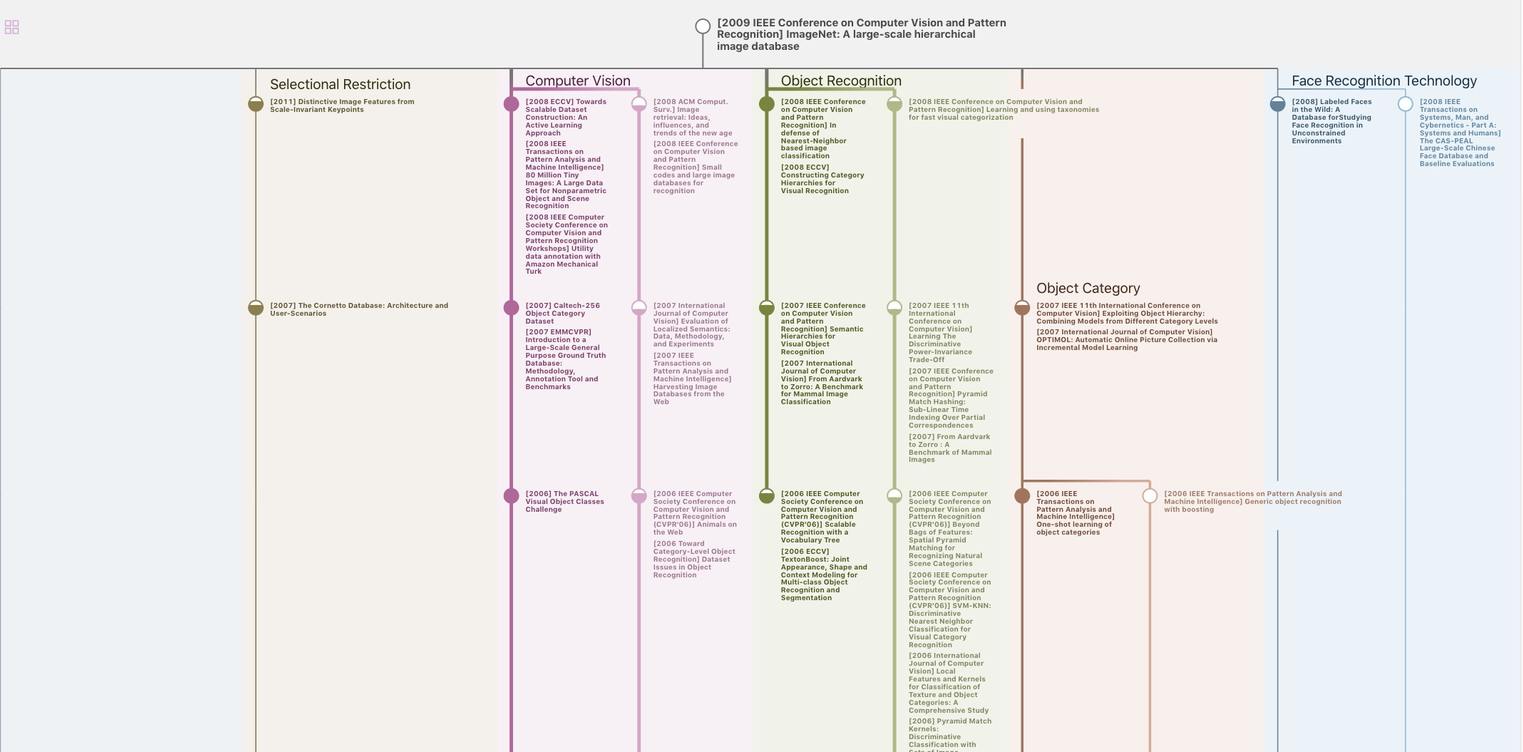
生成溯源树,研究论文发展脉络
Chat Paper
正在生成论文摘要