Natural Language Processing of Learners' Evaluations of Attendings to Identify Professionalism Lapses.
Evaluation & the health professions(2023)
摘要
Unprofessional faculty behaviors negatively impact the well-being of trainees yet are infrequently reported through established reporting systems. Manual review of narrative faculty evaluations provides an additional avenue for identifying unprofessional behavior but is time- and resource-intensive, and therefore of limited value for identifying and remediating faculty with professionalism concerns. Natural language processing (NLP) techniques may provide a mechanism for streamlining manual review processes to identify faculty professionalism lapses. In this retrospective cohort study of 15,432 narrative evaluations of medical faculty by medical trainees, we identified professionalism lapses using automated analysis of the text of faculty evaluations. We used multiple NLP approaches to develop and validate several classification models, which were evaluated primarily based on the positive predictive value (PPV) and secondarily by their calibration. A NLP-model using sentiment analysis (quantifying subjectivity of the text) in combination with key words (using the ensemble technique) had the best performance overall with a PPV of 49% (CI 38%-59%). These findings highlight how NLP can be used to screen narrative evaluations of faculty to identify unprofessional faculty behaviors. Incorporation of NLP into faculty review workflows enables a more focused manual review of comments, providing a supplemental mechanism to identify faculty professionalism lapses.
更多查看译文
关键词
faculty professionalism,identification of professionalism lapses,medical education,natural language processing,professionalism
AI 理解论文
溯源树
样例
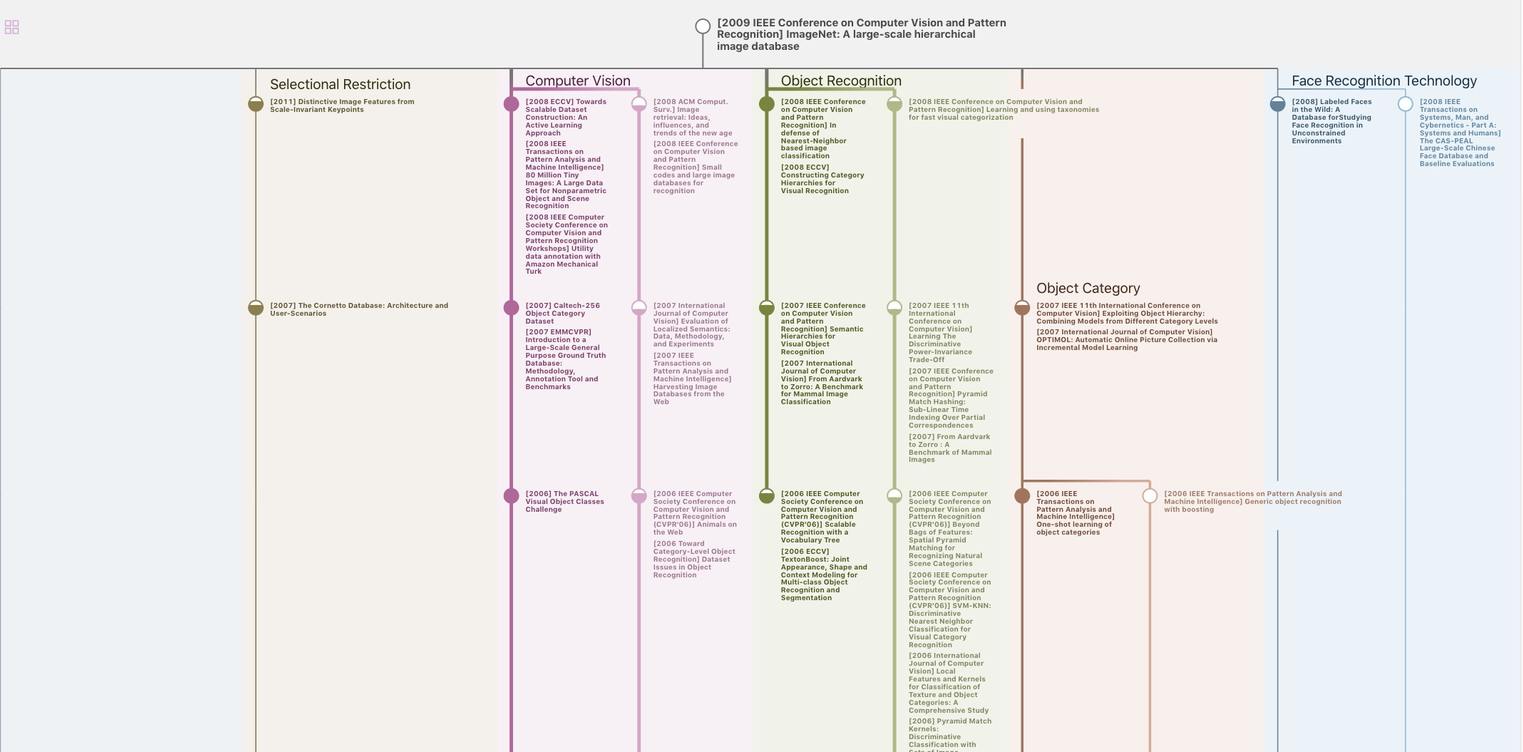
生成溯源树,研究论文发展脉络
Chat Paper
正在生成论文摘要