Clustering Geodata Cubes (CGC) and Its Application to Phenological Datasets
crossref(2023)
摘要
<p>Unsupervised classification techniques are becoming essential to extract information from the wealth of data that Earth observation satellites and other sensors currently provide. These datasets are inherently complex to analyze due to the extent across multiple dimensions - spatial, temporal, and often spectral or band dimension – their size, and the high resolution of current sensors. Traditional one-dimensional cluster analysis approaches, which are designed to find groups of similar elements in datasets such as rasters or time series, may come short of identifying patterns in these higher-dimensional datasets, often referred to as data cubes. In this context, we present our Clustering Geodata Cubes (CGC) software, an open-source Python package that implements a set of co- and tri-clustering algorithms to simultaneously group elements across two and three dimensions, respectively. The package includes different implementations to most efficiently tackle datasets that fit into the memory of a single machine as well as very large datasets that require cluster computing. A refining strategy to facilitate data pattern identification is also provided. We apply CGC to investigate gridded datasets representing the predicted day of the year when spring onset events (first leaf, first bloom) occur according to a well-established phenological model. Specifically, we consider spring indices computed at high spatial resolution (1 km) and continental scale (conterminous United States) for the last 40+ years and extract the main spatiotemporal patterns present in the data via CGC co-clustering functionality.  </p>
更多查看译文
AI 理解论文
溯源树
样例
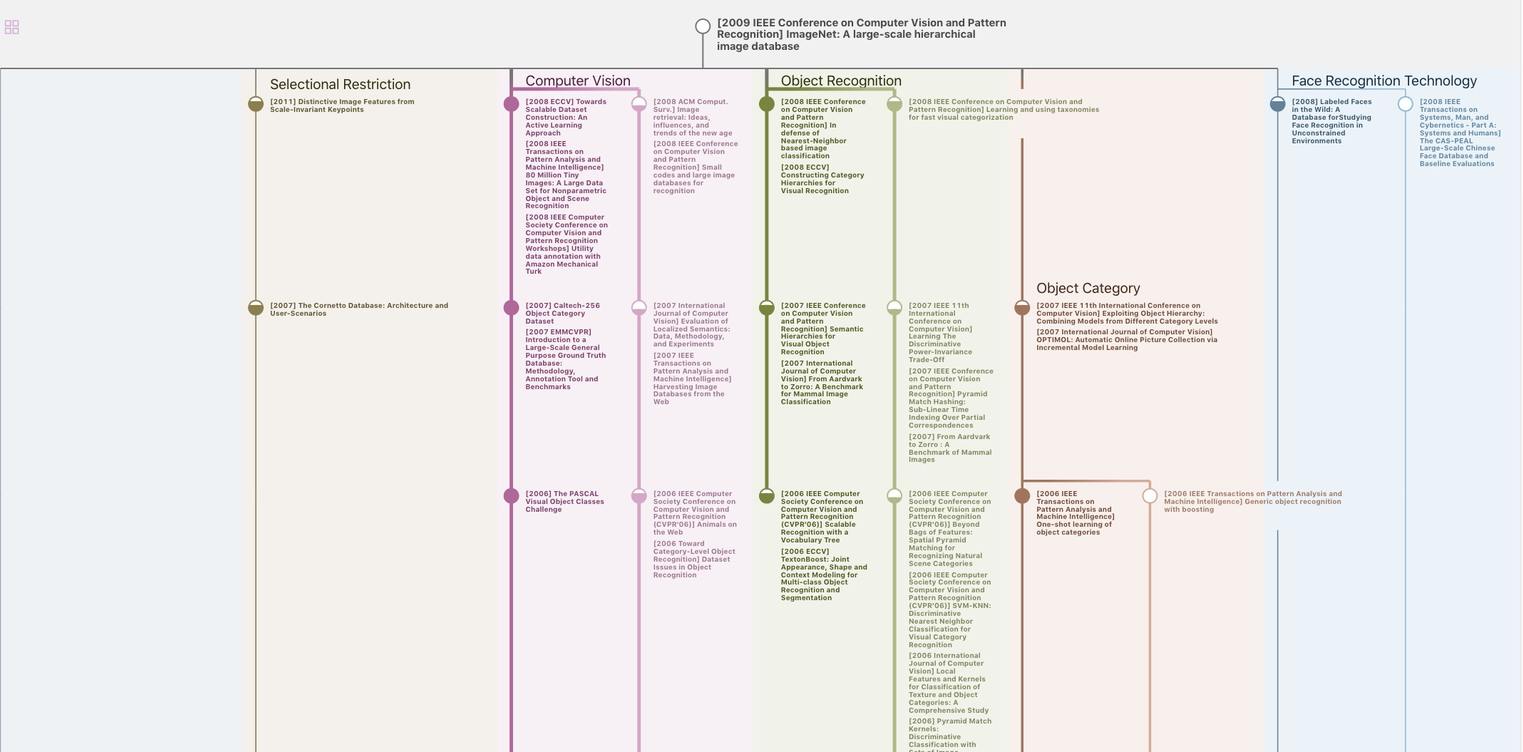
生成溯源树,研究论文发展脉络
Chat Paper
正在生成论文摘要