New trends in urban change detection: detecting 3D changes from bitemporal optical images 
crossref
摘要
<p><strong>Keywords</strong>: Urban sustainability, Earth observation, 3D change detection, Deep Learning, Dataset</p> <p>Nowadays, remote sensing products can provide useful and consistent information about urban areas and their morphological structures with different spatial and temporal resolutions, making it possible to perform long term spatiotemporal analyses of the historic development of the cities and in this way to monitor the evolution of their urbanization patterns, a goal strictly related to the United Nations (UN) Sustainable Development Goals (SDGs) concerning the sustainability of the cities (SDG 11 - Sustainable Cities and Communities).</p> <p>In this context, Change Detection (CD) algorithms estimate the changes occurred at ground level and are employed in a wide range of applications, including the identification of urban changes. Most of the recently developed CD methodologies rely on deep learning architectures. Nevertheless, the CD algorithms currently available are mainly focused on generating two-dimensional (2D) change maps, where the planimetric extent of the areas affected by changes is identified without providing any information on the corresponding elevation (3D) variations. These algorithms can thus only identify planimetric changes such as appearing/disappearing buildings/trees, shrinking/expanding structures and are not able to satisfy the requirements of applications which need to detect and, most of all, to quantify the elevation variations occurred in the area of interest (AOI), such as the estimation of volumetric changes in urban areas.</p> <p>It is therefore essential to develop CD algorithms capable of automatically generating an elevation (3D) CD map (a map containing the quantitative changes in elevation for the AOI) together with a standard 2D CD map, from the smallest possible amount of information. In this contribution, we will present the MultiTask Bitemporal Images Transformer (MTBIT) [1], a recently developed network, belonging to the family of vision Transformers and based on a semantic tokenizer, explicitly designed to solve the 2D and 3D CD tasks simultaneously from bitemporal optical images, and thus without the need to rely directly on elevation data during the inference phase. </p> <p>The MTBIT performances were evaluated in the urban area of Valladolid on the modified version of the 3DCD dataset [2], comparing this architecture with other networks designed to solve the 2D CD task. In particular, MTBIT reaches a metric accuracy equal to 6.46 m – the best performance among the compared architectures – with a limited number of parameters (13,1 M) [1].</p> <p>The code and the 3DCD dataset are available at https://sites.google.com/uniroma1.it/3dchangedetection/home-page.</p> <p> </p> <p><strong>References</strong></p> <p>[1] Marsocci, V., Coletta, V., Ravanelli, R., Scardapane, S., and Crespi, M.: Inferring 3D change detection from bitemporal optical images. <em>ISPRS Journal of Photogrammetry and Remote Sensing</em>. 2023 - in press.</p> <p>[2] Coletta, V., Marsocci, V., and Ravanelli, R.: 3DCD: A NEW DATASET FOR 2D AND 3D CHANGE DETECTION USING DEEP LEARNING TECHNIQUES,<em> The International Archives of the Photogrammetry, Remote Sensing and Spatial Information Sciences</em>, XLIII-B3-2022, 1349–1354, 2022.</p>
更多查看译文
AI 理解论文
溯源树
样例
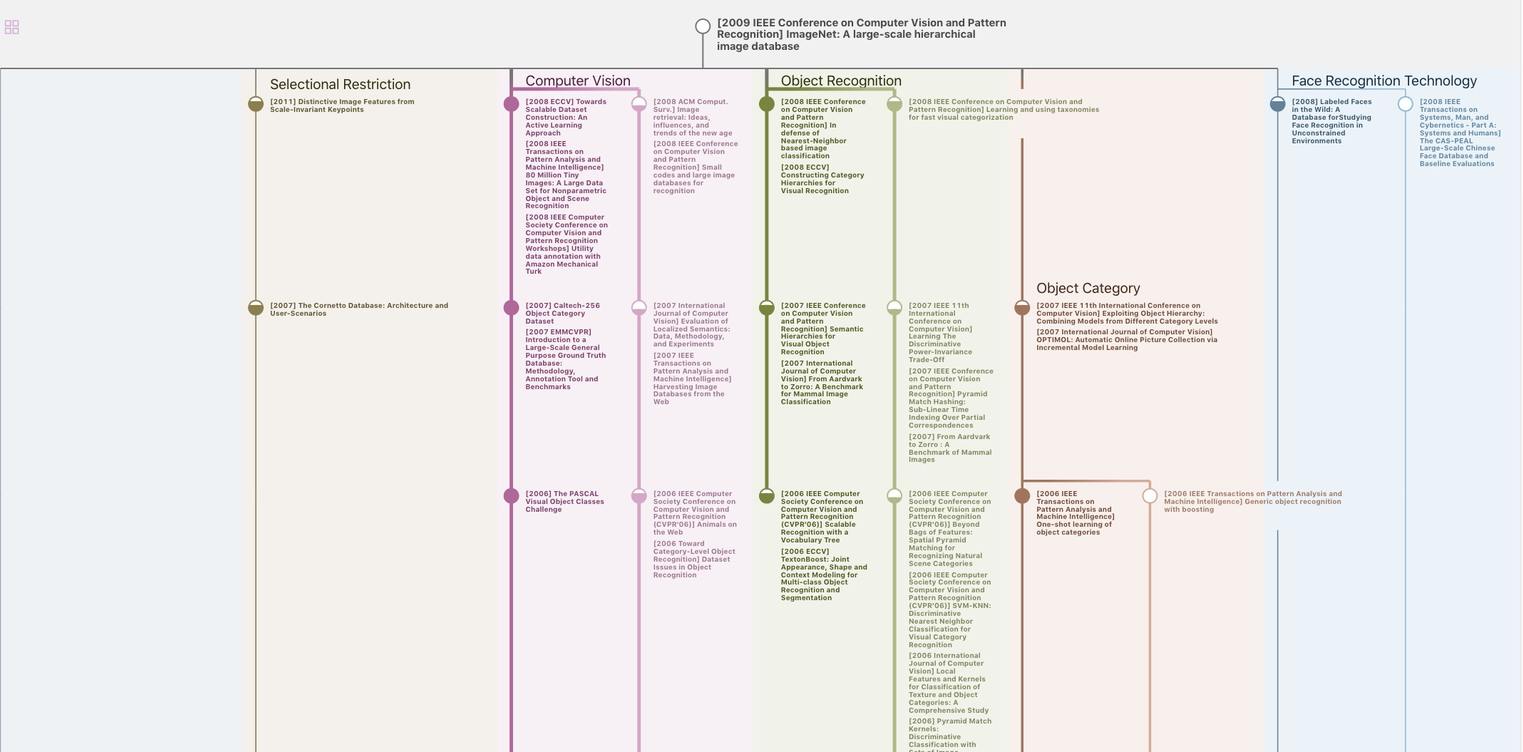
生成溯源树,研究论文发展脉络
Chat Paper
正在生成论文摘要