Learning stiff chemical kinetics using extended deep neural operators
COMPUTER METHODS IN APPLIED MECHANICS AND ENGINEERING(2024)
摘要
We utilize neural operators to learn the solution propagator for challenging systems of differential equations that are representative of stiff chemical kinetics. Specifically, we apply the deep operator network (DeepONet) along with its extensions, such as the autoencoder-based DeepONet and the newly proposed Partition-of-Unity (PoU-) DeepONet to study a range of examples, including the ROBERS problem with three species, the POLLU problem with 25 species, pure kinetics of a skeletal model for CO/H2 burning of syngas, which contains 11 species and 21 reactions and finally, a temporally developing planar CO/H2 jet flame (turbulent flame) using the same syngas mechanism. We have demonstrated the advantages of the proposed approach through these numerical examples. Specifically, to train the DeepONet for the syngas model, we solve the skeletal kinetic model for different initial conditions. In the first case, we parameterize the initial conditions based on equivalence ratios and initial temperature values. In the second case, we perform a direct numerical simulation of a two-dimensional temporally developing CO/H2 jet flame with integrated chemistry modeling as a source of training data for the solution propagator. Then, we initialize the kinetic model by the thermochemical states visited by a subset of grid points at different time snapshots. Stiff chemical kinetics are computationally expensive to solve, thus, this work aims to develop a neural operator-based surrogate model to efficiently solve stiff chemical kinetics. The operator, once trained offline, can accurately integrate the thermochemical state for arbitrarily large time advancements, leading to significant computational gains compared to stiff integration schemes.
更多查看译文
关键词
Stiff problems,Scientific machine learning,Neural operators,Combustion
AI 理解论文
溯源树
样例
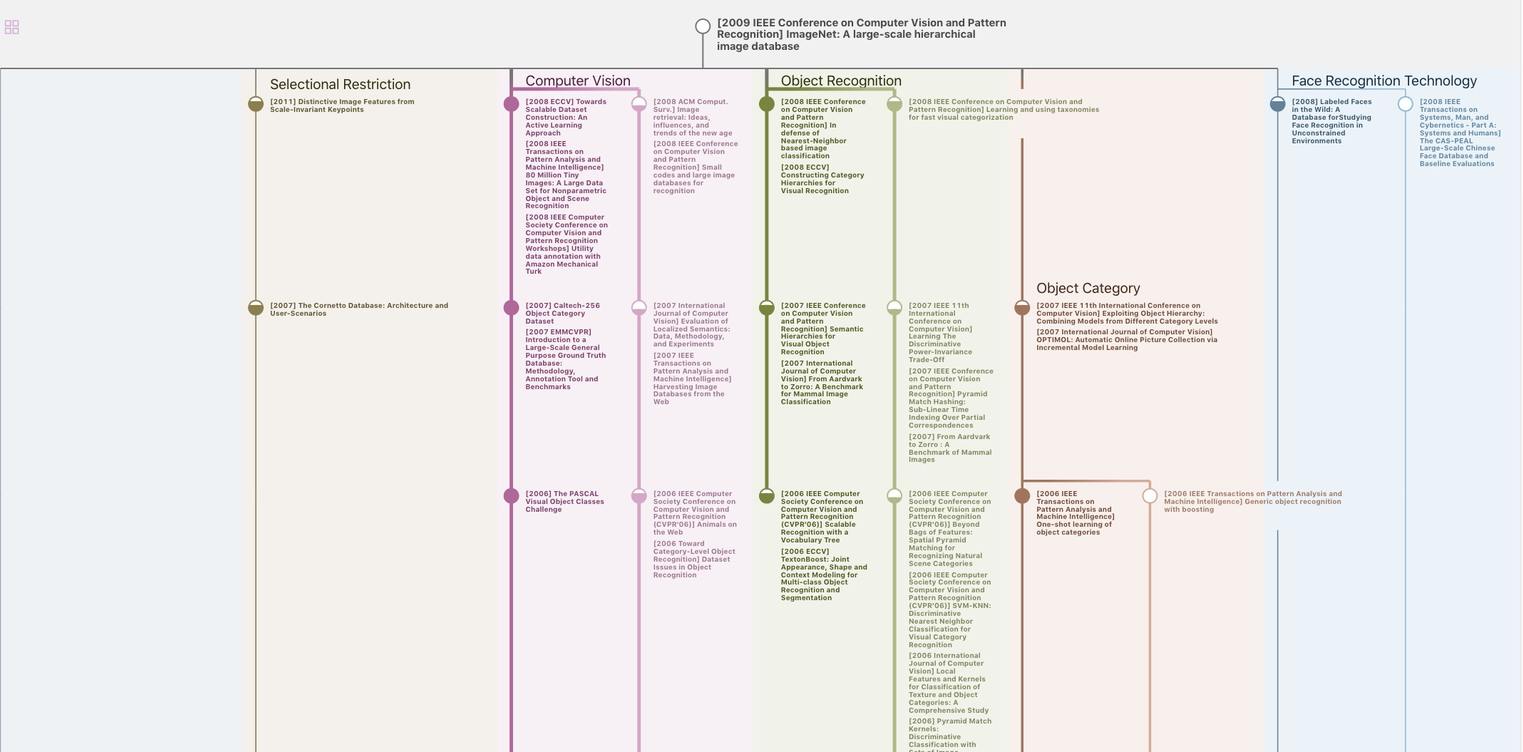
生成溯源树,研究论文发展脉络
Chat Paper
正在生成论文摘要