Evaluation of Athlete Monitoring Tools across 10 Weeks of Elite Youth Basketball Training: An Explorative Study.
Sports (Basel, Switzerland)(2023)
摘要
The growth of sport science technology is enabling more sporting teams to implement athlete monitoring practices related to performance testing and load monitoring. Despite the increased emphasis on youth athlete development, the lack of longitudinal athlete monitoring literature in youth athletes is concerning, especially for indoor sports such as basketball. The aim of this study was to evaluate the effectiveness of six different athlete monitoring methods over 10 weeks of youth basketball training. Fourteen state-level youth basketball players (5 males and 9 females; 15.1 ± 1.0 years) completed this study during their pre-competition phase prior to their national basketball tournament. Daily wellness and activity surveys were completed using the mobile application, along with heart rate (HR) and inertial measurement unit (IMU) recordings at each state training session, and weekly performance testing (3x countermovement jumps [CMJs], and 3x isometric mid-thigh pulls [IMTPs]). All of the athlete monitoring methods demonstrated the coaching staff's training intent to maintain performance and avoid spikes in workload. Monitoring IMU data combined with PlayerLoad™ data analysis demonstrated more effectiveness for monitoring accumulated load (AL) compared to HR analysis. All six methods of athlete monitoring detected similar trends for all sessions despite small-trivial correlations between each method (Pearson's correlation: -0.24 < r < 0.28). The use of subjective monitoring questionnaire applications, such as , is recommended for youth sporting clubs, given its practicability and low-cost. Regular athlete education from coaches and support staff regarding the use of these questionnaires is required to gain the best data.
更多查看译文
关键词
external,internal,junior,questionnaire,workload
AI 理解论文
溯源树
样例
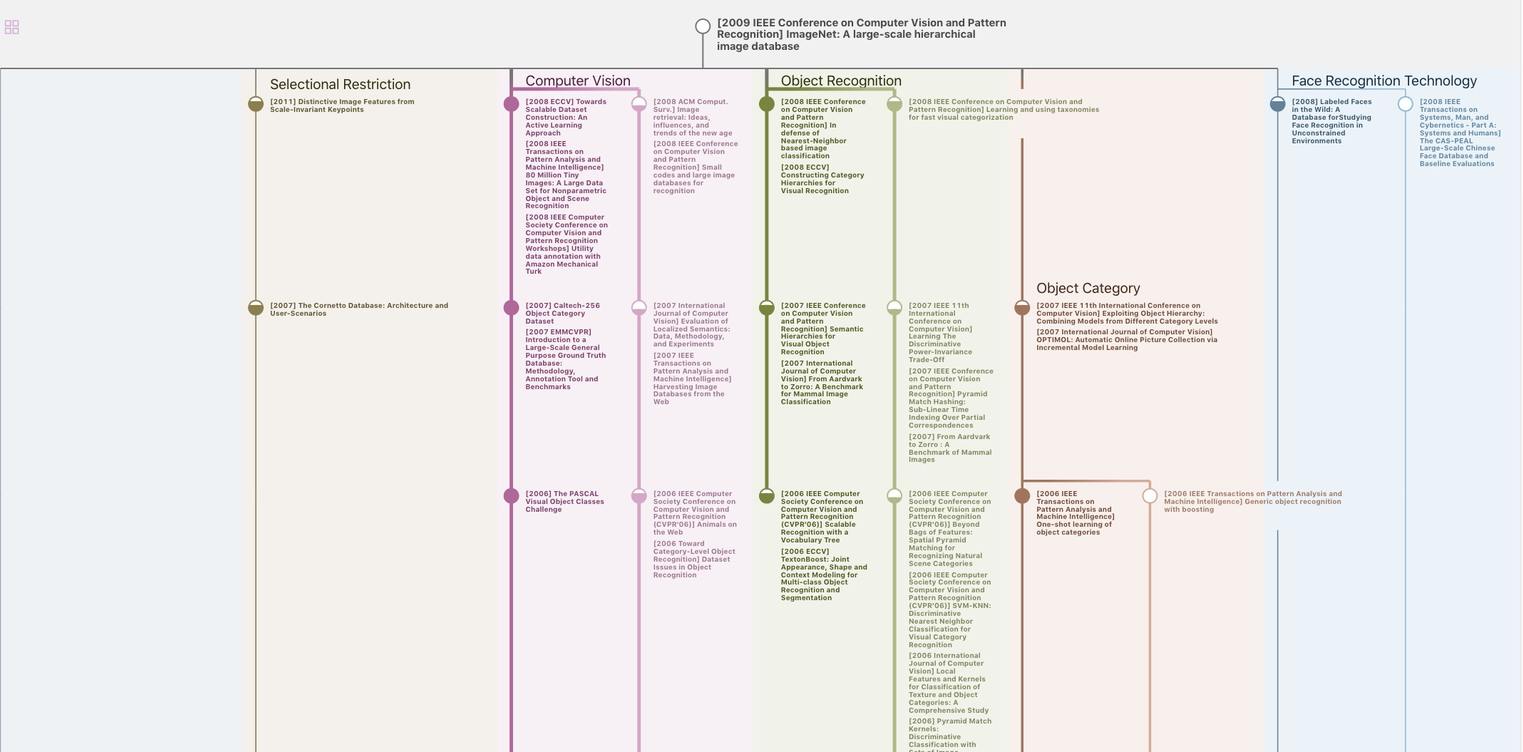
生成溯源树,研究论文发展脉络
Chat Paper
正在生成论文摘要