The promise of large language models in health care.
Lancet (London, England)(2023)
摘要
The past few months have seen a rapid acceleration of innovation in the field of generative artificial intelligence with large language models (LLMs), capturing academic, media, and public attention. GPT-3, BERT, and ChatGPT are examples of LLMs that have been trained on swathes of the internet to produce impressive responses to human queries. The ability of these generalised LLMs to write fiction, develop computer code, and speculate on the future has prompted recognition that we are approaching artificial general intelligence for the first time.1Lauret J GPT-3: the first artificial general intelligence?.https://towardsdatascience.com/gpt-3-the-first-artificial-general-intelligence-b8d9b38557a1Date: July 22, 2020Date accessed: January 14, 2023Google Scholar It has already been proposed that LLMs, such as ChatGPT, could have applications in the field of health care due to the large volumes of free-text information available for training models. It has been thought that models could help with the writing of discharge letters by summarising a patient's hospital stay after reading their medical records. It has also been proposed that LLMs could be used in medical research, which is supported by the empirical example of GatorTron, an LLM trained on more than 90 billion words of text from electronic health records.2Yang X Chen A PourNejatian N et al.A large language model for electronic health records.Npj Digit Med. 2022; 5: 1-9Crossref PubMed Scopus (9) Google Scholar The same model was tested on its ability to answer medical questions in a natural language. These capabilities attract speculation on the use of LLMs as a medical triage service or an application to provide plain English answers to medical questions from the public. However, it must be emphasised that such technologies in their current form have the potential to incite harm, an effect that has been well described in the field of mental health, where natural language processing algorithms have shown biases relating to religion, race, gender, nationality, sexuality, and age.3Straw I Callison-Burch C Artificial intelligence in mental health and the biases of language based models.PLoS One. 2020; 15e0240376Crossref Scopus (19) Google Scholar Although already attracting widespread speculation about their future potential, LLMs are still in their infancy. Current limitations include the amount of computer power required for LLMs to function, which is often costly. LLMs also require huge volumes of data to be trained effectively, which might dictate that the sharing of data between institutions could be required to train algorithms. Such data sharing presents a unique challenge in the field of health care, as strict data privacy laws and institutional data protection agreements contribute to data silos. Solutions are emerging, such as the use of federated learning or synthetic data to allow cross-border data sharing.4Arora A Arora A Generative adversarial networks and synthetic patient data: current challenges and future perspectives.Future Healthc J. 2022; 9: 190-193Crossref PubMed Google Scholar Although these techniques have proved successful in other domains, their applicability to LLMs remains uncertain. However uncertain the future of this research is does not make it impossible, nor far away. AnmA has roles with the UK National Institute for Health Research, Health Data Research UK, NHS England & Improvement, and Moorfields Eye Hospital. AnaA declares no competing interests.
更多查看译文
关键词
large language models,health care
AI 理解论文
溯源树
样例
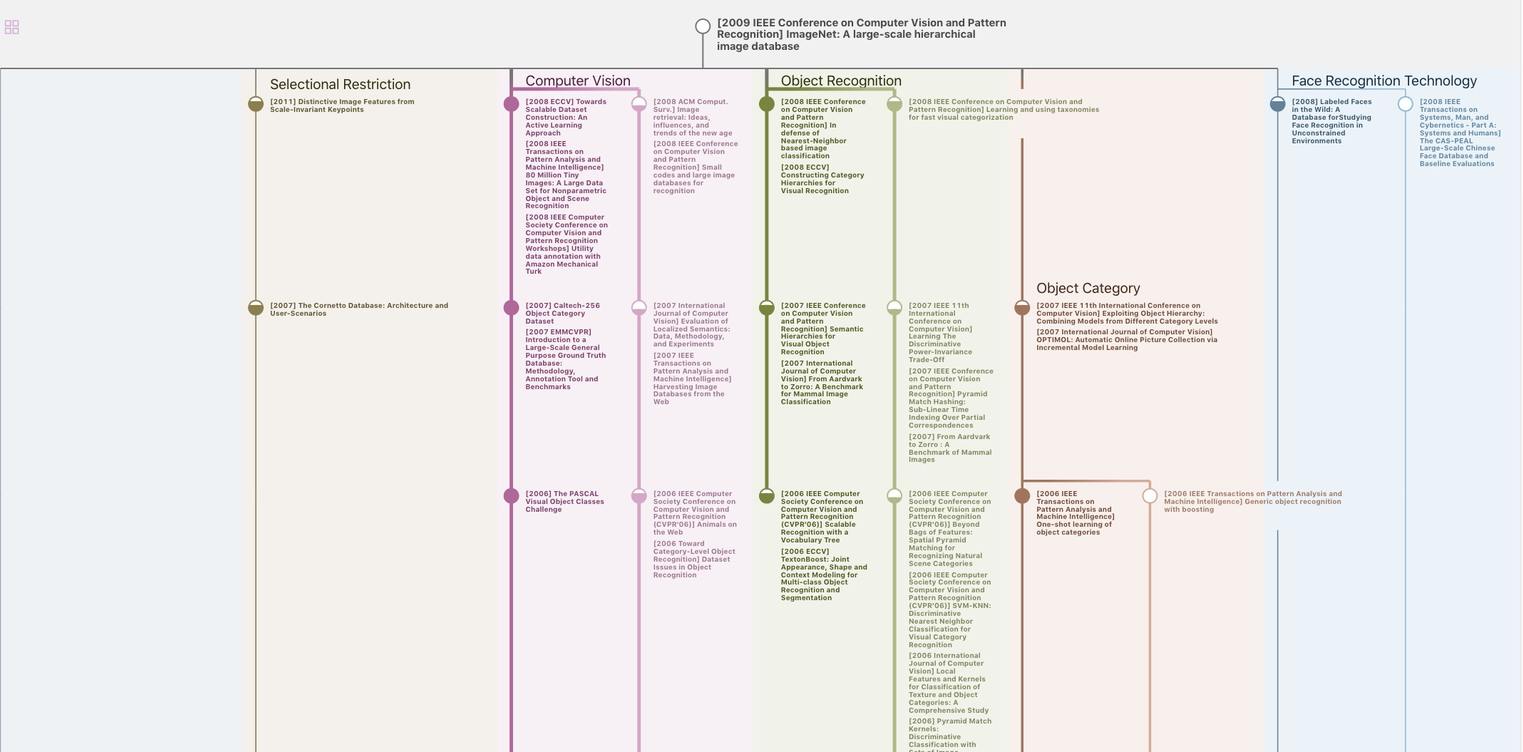
生成溯源树,研究论文发展脉络
Chat Paper
正在生成论文摘要