CDPMSR: Conditional Diffusion Probabilistic Models for Single Image Super-Resolution
arxiv(2023)
摘要
Diffusion probabilistic models (DPM) have been widely adopted in image-to-image translation to generate high-quality images. Prior attempts at applying the DPM to image super-resolution (SR) have shown that iteratively refining a pure Gaussian noise with a conditional image using a U-Net trained on denoising at various-level noises can help obtain a satisfied high-resolution image for the low-resolution one. To further improve the performance and simplify current DPM-based super-resolution methods, we propose a simple but non-trivial DPM-based super-resolution post-process framework,i.e., cDPMSR. After applying a pre-trained SR model on the to-be-test LR image to provide the conditional input, we adapt the standard DPM to conduct conditional image generation and perform super-resolution through a deterministic iterative denoising process. Our method surpasses prior attempts on both qualitative and quantitative results and can generate more photo-realistic counterparts for the low-resolution images with various benchmark datasets including Set5, Set14, Urban100, BSD100, and Manga109. Code will be published after accepted.
更多查看译文
关键词
Diffusion Probabilistic Models,Image-to-Image Translation,Conditional Image Generation,Image Super-resolution
AI 理解论文
溯源树
样例
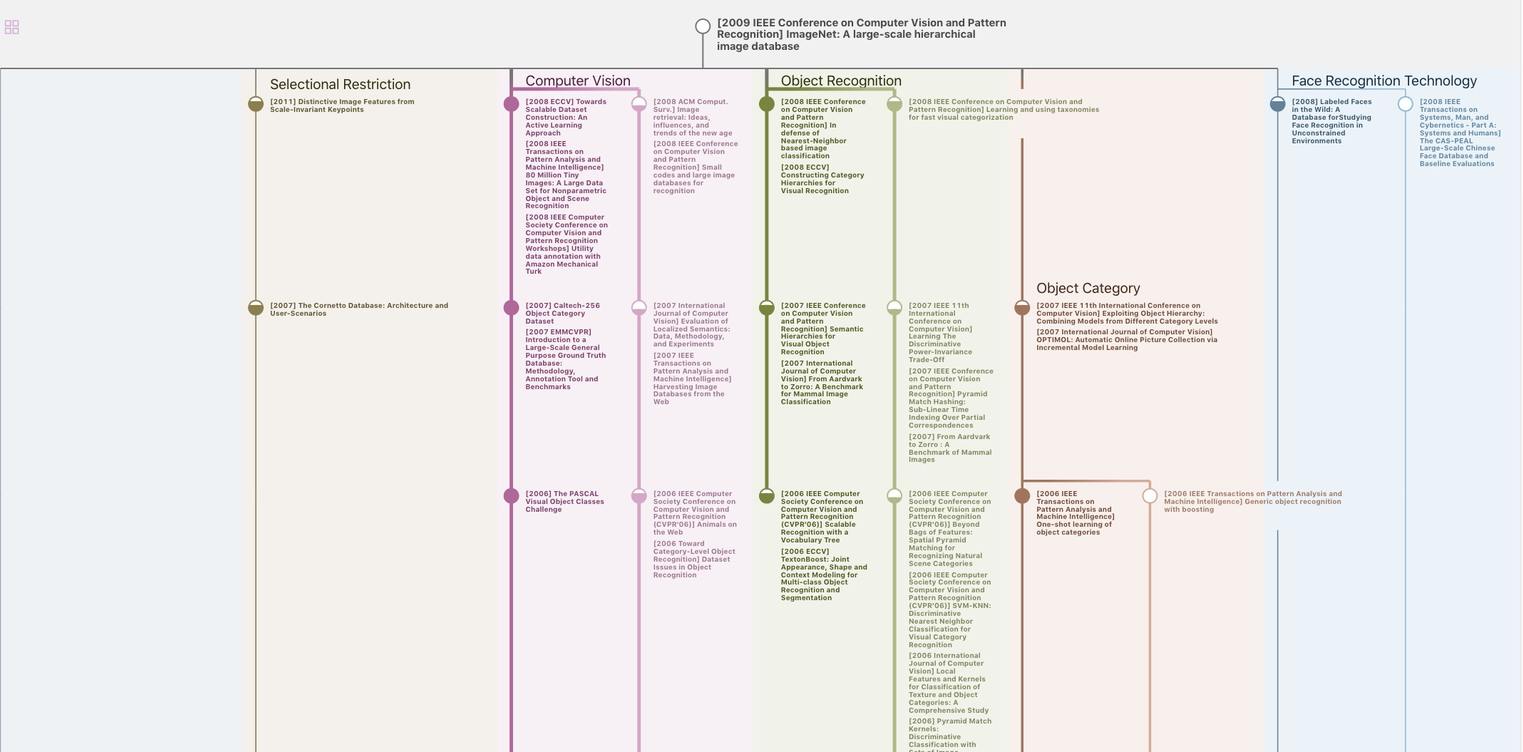
生成溯源树,研究论文发展脉络
Chat Paper
正在生成论文摘要