A deep learning approach for patchless estimation of ultrasound quantitative parametric image with uncertainty measurement
MEDICAL IMAGING 2023(2023)
摘要
Quantitative ultrasound (QUS) aims to find properties of scatterers which are related to the tissue microstructure. Among different QUS parameters, scatterer number density has been found to be a reliable biomarker to detect different abnormalities. The homodyned K-distribution (HK-distribution) is a model for the probability density function of the ultrasound echo amplitude that can model different scattering scenarios but requires a large number of samples to be estimated reliably. Parametric images of HK-distribution parameters can be formed by dividing the envelope data into small overlapping patches and estimating parameters within the patches independently. This approach imposes two limiting constraints: the HK-distribution parameters are assumed to be constant within each patch, and each patch requires enough independent samples. In order to mitigate those problems, we employ a deep learning approach to estimate parametric images of scatterer number density (related to HK-distribution shape parameter) without patching. Furthermore, an uncertainty map of the network's prediction is quantified to provide insight about the confidence of the network about the estimated HK parameter values.
更多查看译文
关键词
Quantitative Ultrasound,Homodyned K-distribution,Parametric Image,Deep Learning
AI 理解论文
溯源树
样例
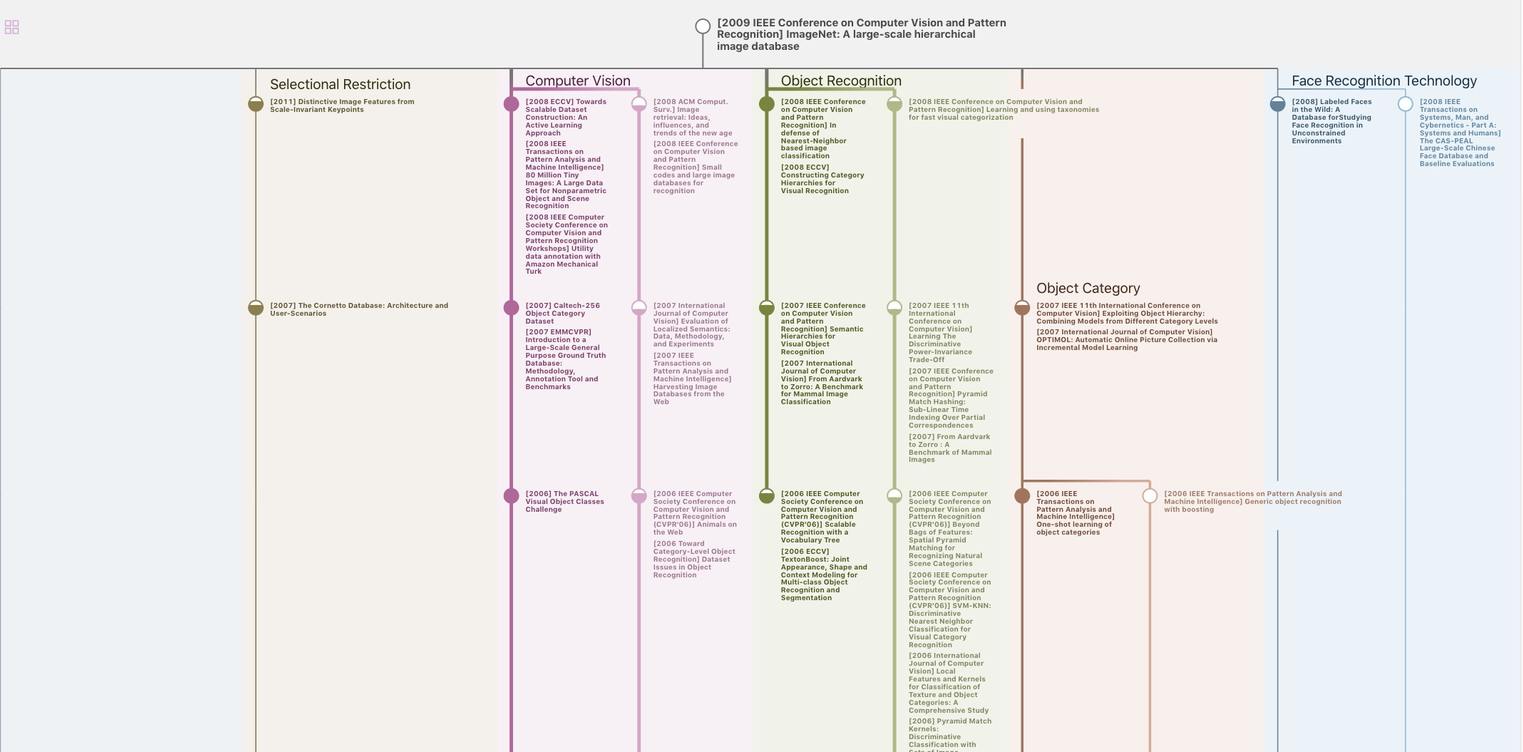
生成溯源树,研究论文发展脉络
Chat Paper
正在生成论文摘要