Choice Fusion as Knowledge for Zero-Shot Dialogue State Tracking
arxiv(2023)
摘要
With the demanding need for deploying dialogue systems in new domains with less cost, zero-shot dialogue state tracking (DST), which tracks user's requirements in task-oriented dialogues without training on desired domains, draws attention increasingly. Although prior works have leveraged question-answering (QA) data to reduce the need for in-domain training in DST, they fail to explicitly model knowledge transfer and fusion for tracking dialogue states. To address this issue, we propose CoFunDST, which is trained on domain-agnostic QA datasets and directly uses candidate choices of slot-values as knowledge for zero-shot dialogue-state generation, based on a T5 pre-trained language model. Specifically, CoFunDST selects highly-relevant choices to the reference context and fuses them to initialize the decoder to constrain the model outputs. Our experimental results show that our proposed model achieves outperformed joint goal accuracy compared to existing zero-shot DST approaches in most domains on the MultiWOZ 2.1. Extensive analyses demonstrate the effectiveness of our proposed approach for improving zero-shot DST learning from QA.
更多查看译文
关键词
Dialogue state tracking,zero-shot,question answering,pre-trained language model,knowledge fusion
AI 理解论文
溯源树
样例
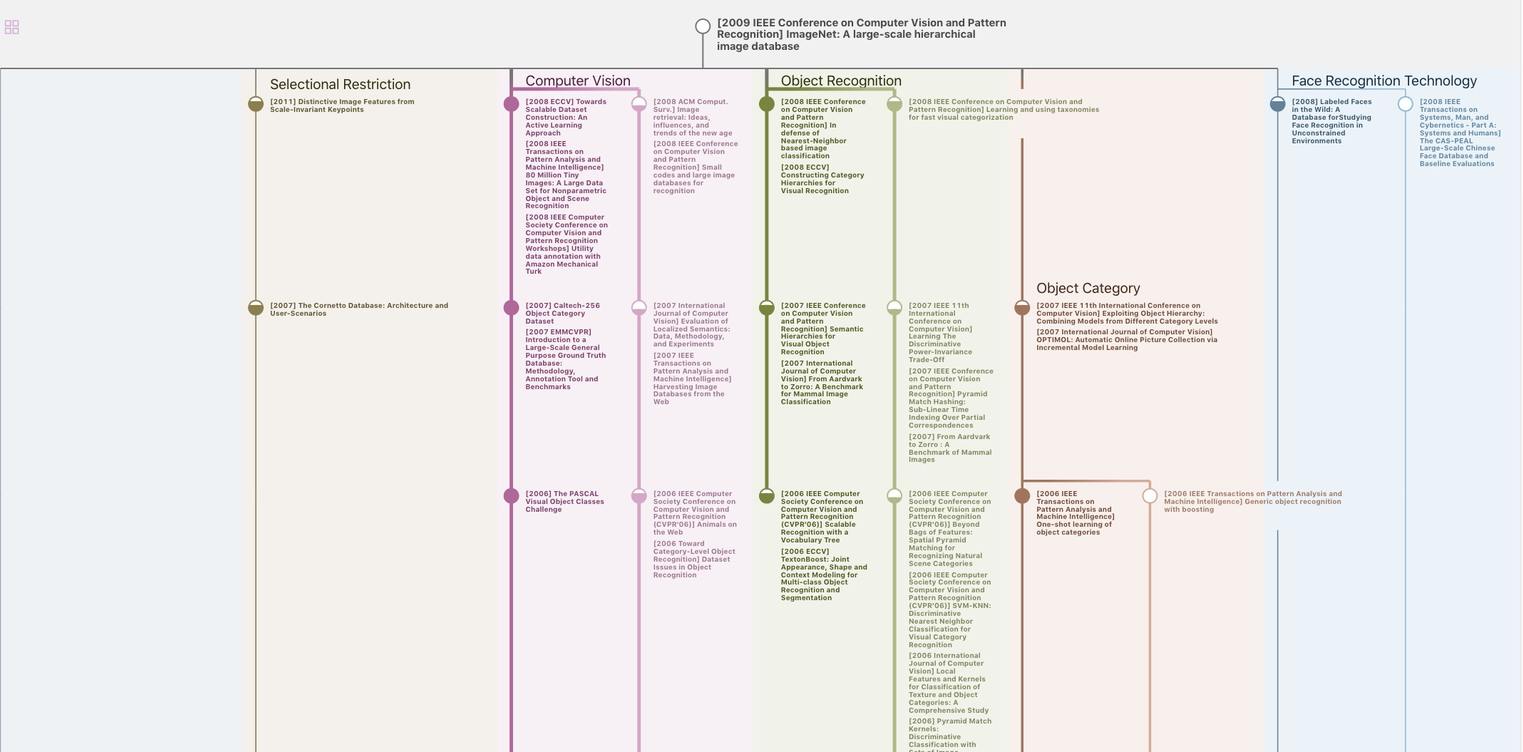
生成溯源树,研究论文发展脉络
Chat Paper
正在生成论文摘要