Concept-Level Explanation for the Generalization of a DNN
arxiv(2023)
摘要
This paper explains the generalization power of a deep neural network (DNN) from the perspective of interactive concepts. Many recent studies have quantified a clear emergence of interactive concepts encoded by the DNN, which have been observed on different DNNs during the learning process. Therefore, in this paper, we investigate the generalization power of each interactive concept, and we use the generalization power of different interactive concepts to explain the generalization power of the entire DNN. Specifically, we define the complexity of each interactive concept. We find that simple concepts can be better generalized to testing data than complex concepts. The DNN with strong generalization power usually learns simple concepts more quickly and encodes fewer complex concepts. More crucially, we discover the detouring dynamics of learning complex concepts, which explain both the high learning difficulty and the low generalization power of complex concepts.
更多查看译文
关键词
generalization,dnn,concept-level
AI 理解论文
溯源树
样例
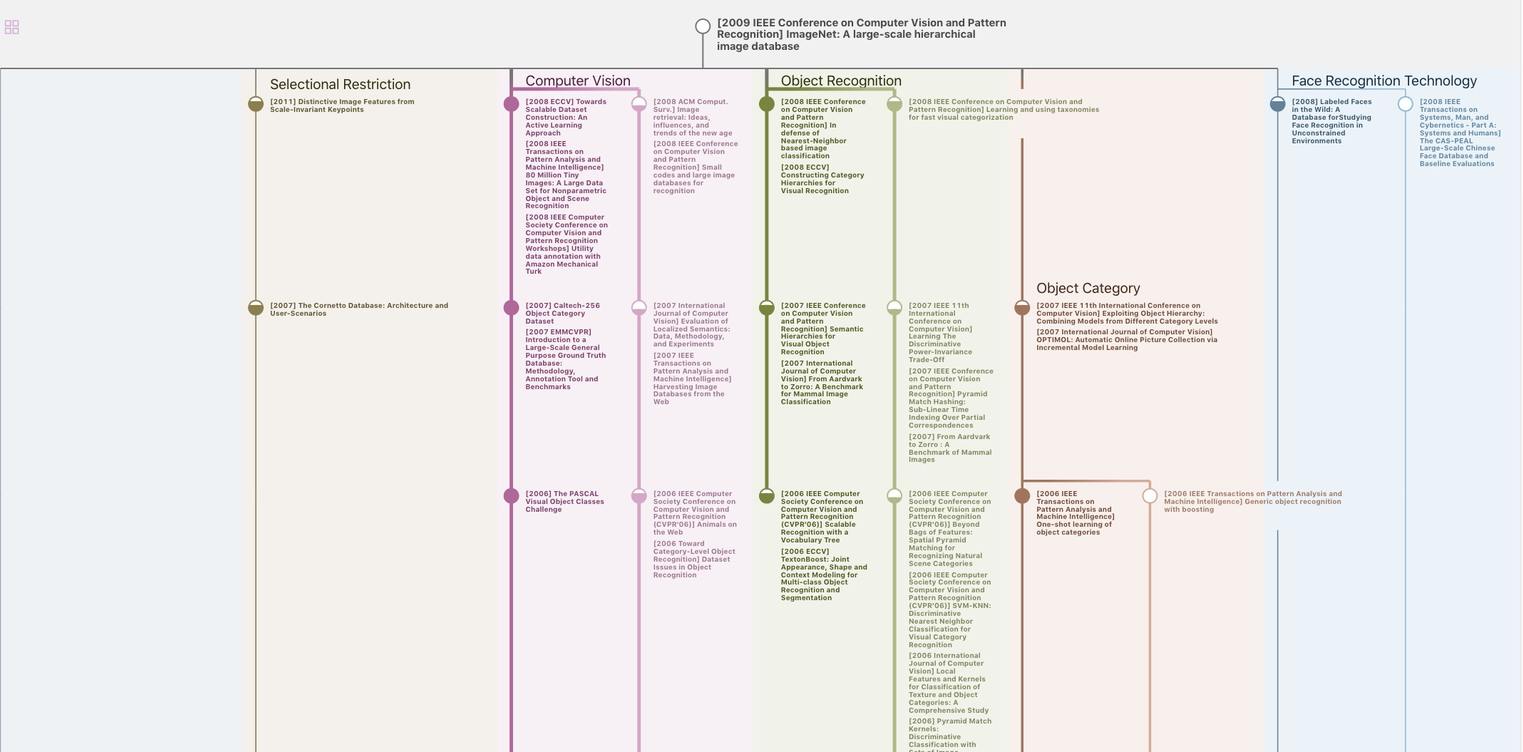
生成溯源树,研究论文发展脉络
Chat Paper
正在生成论文摘要