Optimal feedback control of dynamical systems via value-function approximation
Comptes Rendus Mécanique(2023)
摘要
A self-learning approach for optimal feedback gains for finite-horizon nonlinear continuous time control systems is proposed and analysed. It relies on parameter dependent approximations to the optimal value function obtained from a family of universal approximators. The cost functional for the training of an approximate optimal feedback law incorporates two main features. First, it contains the average over the objective functional values of the parametrized feedback control for an ensemble of initial values. Second, it is adapted to exploit the relationship between the maximum principle and dynamic programming. Based on universal approximation properties, existence, convergence and first order optimality conditions for optimal neural network feedback controllers are proved.
更多查看译文
关键词
optimal feedback control,neural networks,Hamilton–Jacobi–Bellman equation,self-learning,reinforcement learning
AI 理解论文
溯源树
样例
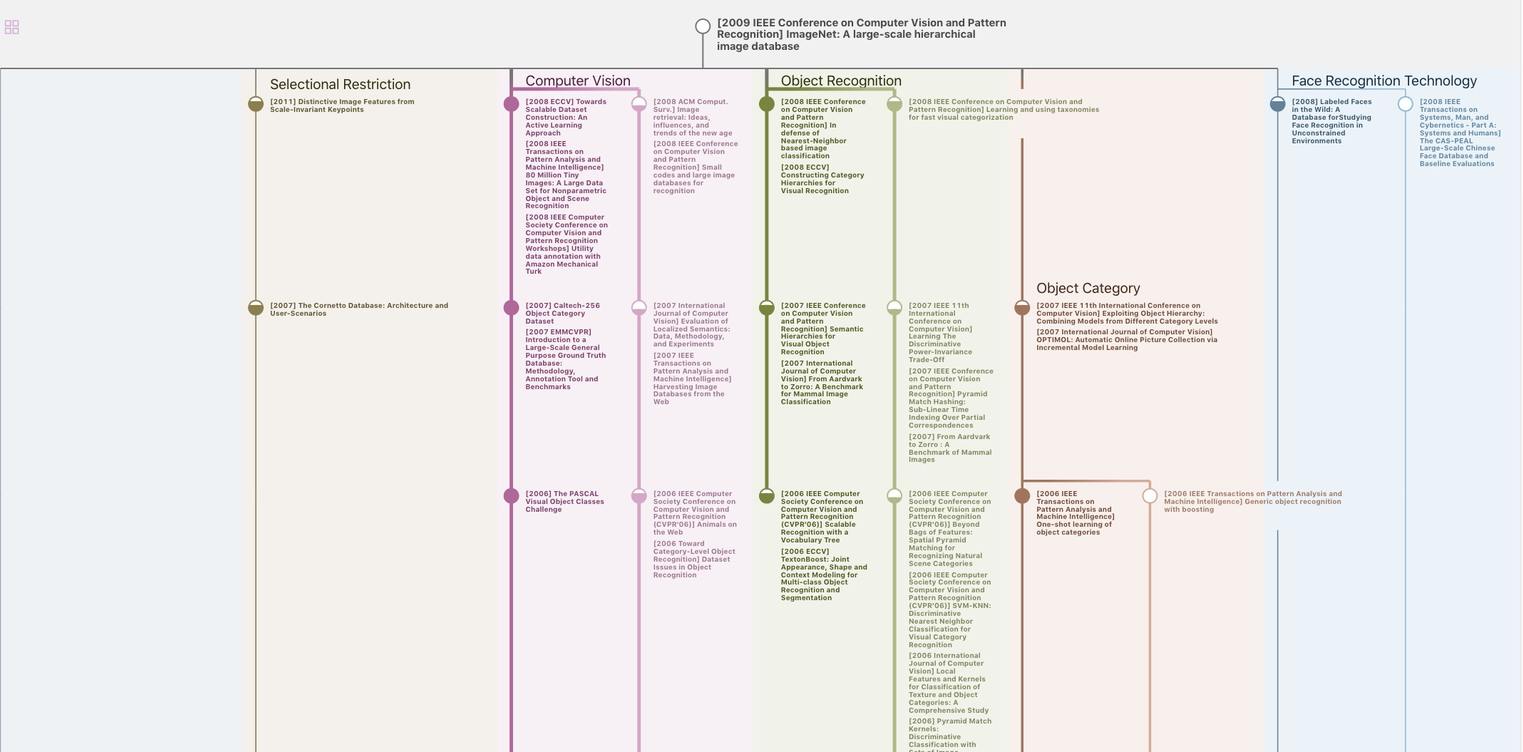
生成溯源树,研究论文发展脉络
Chat Paper
正在生成论文摘要