Embodied Self-Supervised Learning (EMSSL) with Sampling and Training Coordination for Robot Arm Inverse Kinematic Model Learning
2023 IEEE INTERNATIONAL CONFERENCE ON DEVELOPMENT AND LEARNING, ICDL(2023)
摘要
Forward and inverse kinematic models are fundamental to robot arms, serving as the basis for the robot arm's operational tasks. However, in model learning of robot arms, especially in the presence of redundant degrees of freedom, inverse kinematic model learning is more challenging than forward kinematic model learning due to the non-convex problem caused by multiple solutions. Besides, Current learning-based methods often segregate data sampling from model training, potentially leading to suboptimal data utilization and restricted model adaptability. In this paper, we introduce the concept of "Embodiment" and propose a framework for autonomous learning of the robot arm inverse kinematic model based on embodied self-supervised learning (EMSSL) with sampling and training coordination, effectively solving the non-convex problem of the inverse kinematic model and significantly enhancing data sampling efficiency. Concurrently, we investigate batch inference and parallel computation strategies for data sampling to expedite model learning. Additionally, we develop two approaches for the fast adaptation of the robot arm models. A series of experimental evaluations attest to the efficacy of our proposed method.
更多查看译文
关键词
robot arm inverse kinematics model,embodied self-supervised learning (EMSSL),data sampling,model training,coordination
AI 理解论文
溯源树
样例
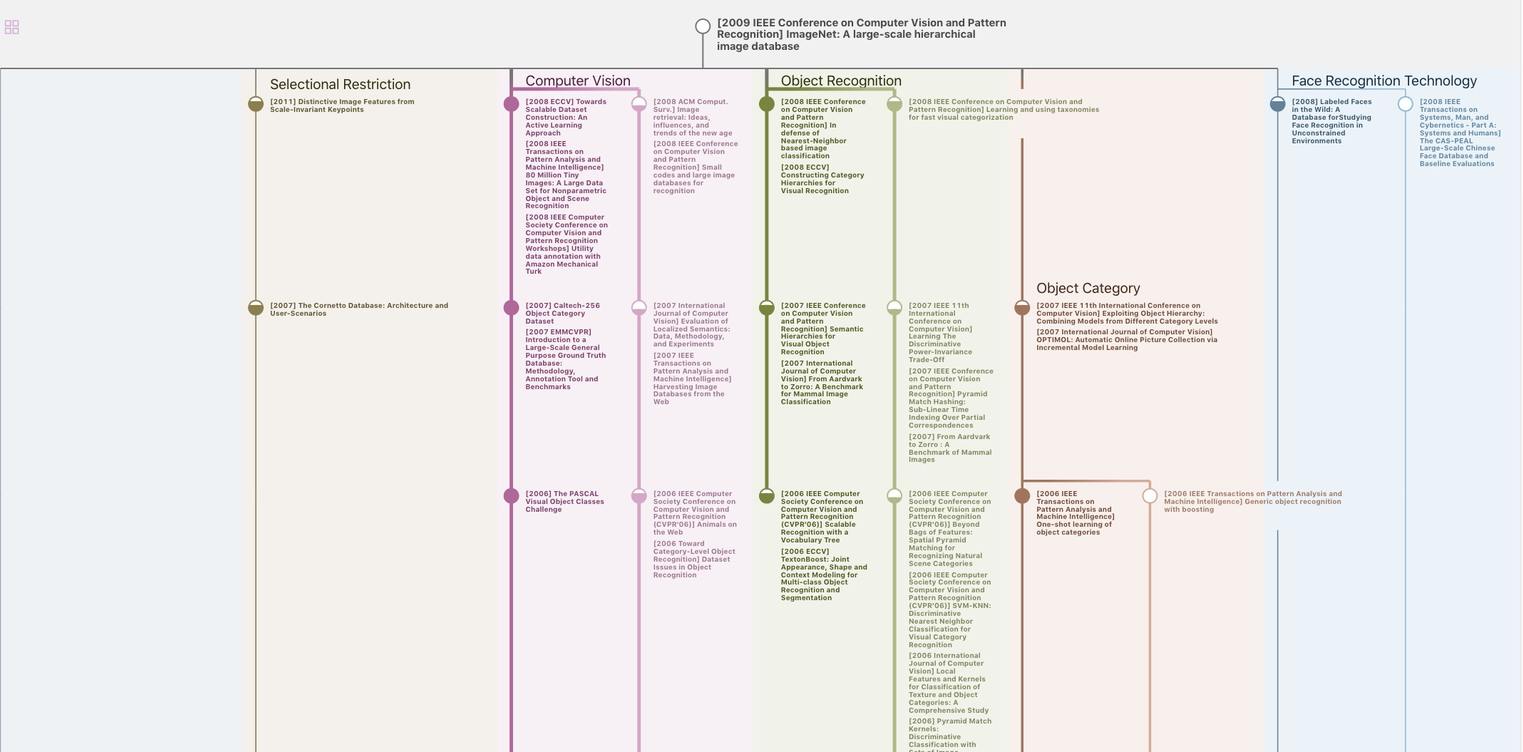
生成溯源树,研究论文发展脉络
Chat Paper
正在生成论文摘要