Repeated Purification Versus Concatenated Error Correction in Fault Tolerant Quantum Networks
arXivorg(2023)
Abstract
Entanglement distribution is a core mechanism for the future quantum Internet. The quantum world is, however, a faulty environment. Hence, successful entanglement swapping is error-prone. The occurrence of quantum state errors can be mitigated using purification and error correction, which can be repeated in the former case and concatenated in the latter case. Repeated purification merges low-fidelity qubits into higher-quality ones, while concatenated error correction builds upon the redundancy of quantum information. In this article, we study in-depth and compare the two options: repeated purification and concatenated error correction. We consider using repeated purification and concatenated error correction to mitigate the presence of faults that occur during the establishment of Bell pairs between remote network nodes. We compare their performance versus the number of repetitions or concatenations, to reach a certain level of fidelity in quantum networks. We study their resource requirements, namely, their work memory complexity (e.g., number of stored qubits) and operational complexity (e.g., number of operations). Our analysis demonstrates that concatenated error correction, versus repeated purification, requires fewer iterations and has lower operational complexity than repeated purification to reach high fidelity at the expense of increased memory requirements.
MoreTranslated text
Key words
Quantum Error Correction,Fault-tolerant Quantum Computation,Quantum Computation,Quantum Machine Learning,Computation
PDF
View via Publisher
AI Read Science
AI Summary
AI Summary is the key point extracted automatically understanding the full text of the paper, including the background, methods, results, conclusions, icons and other key content, so that you can get the outline of the paper at a glance.
Example
Background
Key content
Introduction
Methods
Results
Related work
Fund
Key content
- Pretraining has recently greatly promoted the development of natural language processing (NLP)
- We show that M6 outperforms the baselines in multimodal downstream tasks, and the large M6 with 10 parameters can reach a better performance
- We propose a method called M6 that is able to process information of multiple modalities and perform both single-modal and cross-modal understanding and generation
- The model is scaled to large model with 10 billion parameters with sophisticated deployment, and the 10 -parameter M6-large is the largest pretrained model in Chinese
- Experimental results show that our proposed M6 outperforms the baseline in a number of downstream tasks concerning both single modality and multiple modalities We will continue the pretraining of extremely large models by increasing data to explore the limit of its performance
Try using models to generate summary,it takes about 60s
Must-Reading Tree
Example
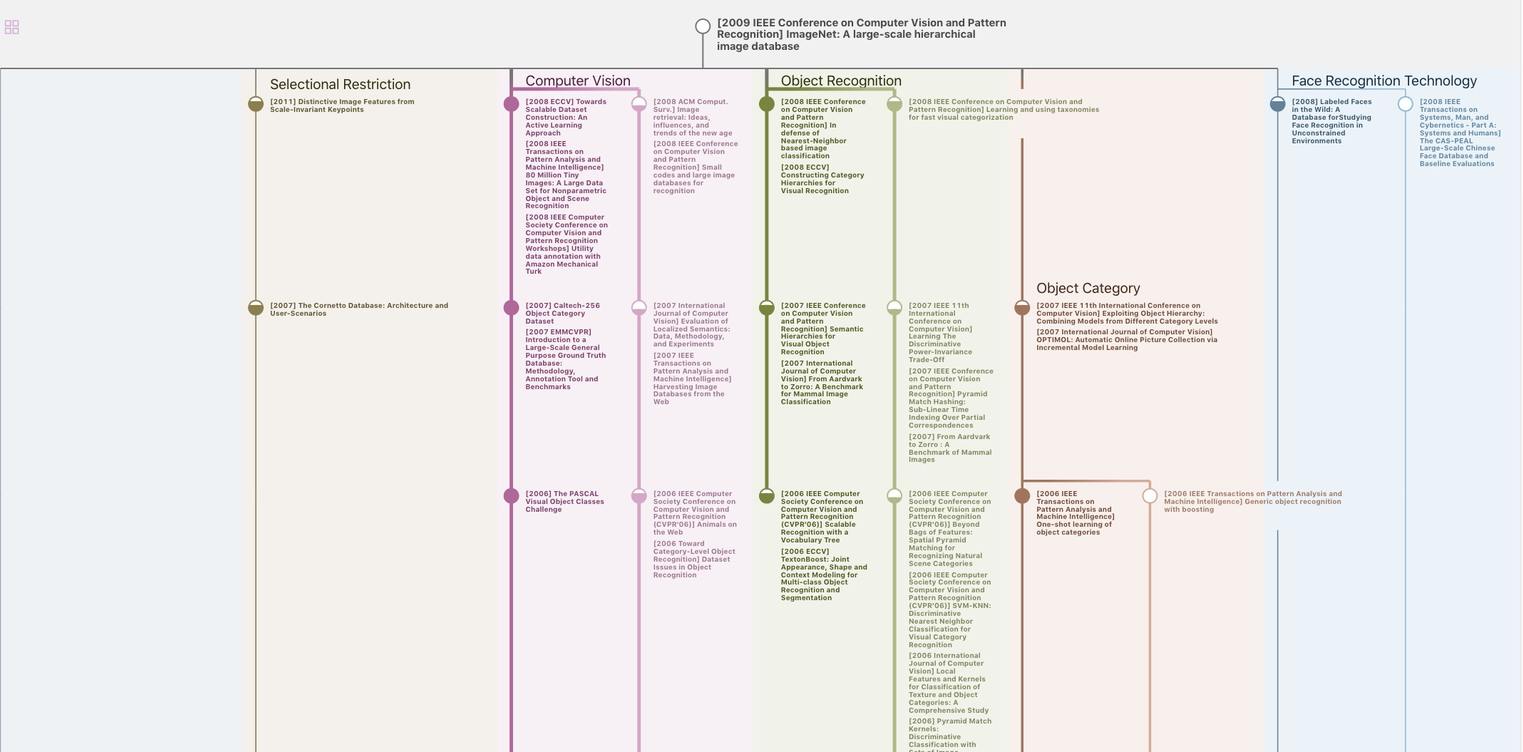
Generate MRT to find the research sequence of this paper
Related Papers
2022
被引用4 | 浏览
Data Disclaimer
The page data are from open Internet sources, cooperative publishers and automatic analysis results through AI technology. We do not make any commitments and guarantees for the validity, accuracy, correctness, reliability, completeness and timeliness of the page data. If you have any questions, please contact us by email: report@aminer.cn
Chat Paper
GPU is busy, summary generation fails
Rerequest