A Hybrid Physics and Active Learning Model For CFD-Based Pipeline CO2 and O2 Corrosion Prediction
Day 3 Fri, March 03, 2023(2023)
摘要
Abstract Pipeline corrosion induced from CO2 or O2 is a serious and costly hazard for oil/gas industry. CO2 and O2 are different complex corrosion processes. We developed an innovative hybrid model that combines both the first principal physics and advanced machine learning (ML) method to build a single model that can predict multiple corrosion mechanisms involving CO2 andO2. It can significantly speed up corrosion analyses in complex geometry using computational fluid dynamics (CFD). The ML prediction output was used to account for the local effects of mass transfer limitations, which requires only four variables: average inlet_velocity, pipe_ID, pipe_bend_angle and the ratio of pipe_bend_radius and pipe_ID instead of the 7 variables including CO2 partial pressure, pH value and temperature. The last three variables were found to be almost independent on the local flow variables because CFD solutions were only obtained at the macroscale level while the microscale surface variable values are solved using mass transfer limitation correlations. This new approach greatly lowered the number of CFD simulations needed to generate data for machine learning models. The hybrid model is about 106 times faster than the CFD simulation with acceptable accuracies.
更多查看译文
AI 理解论文
溯源树
样例
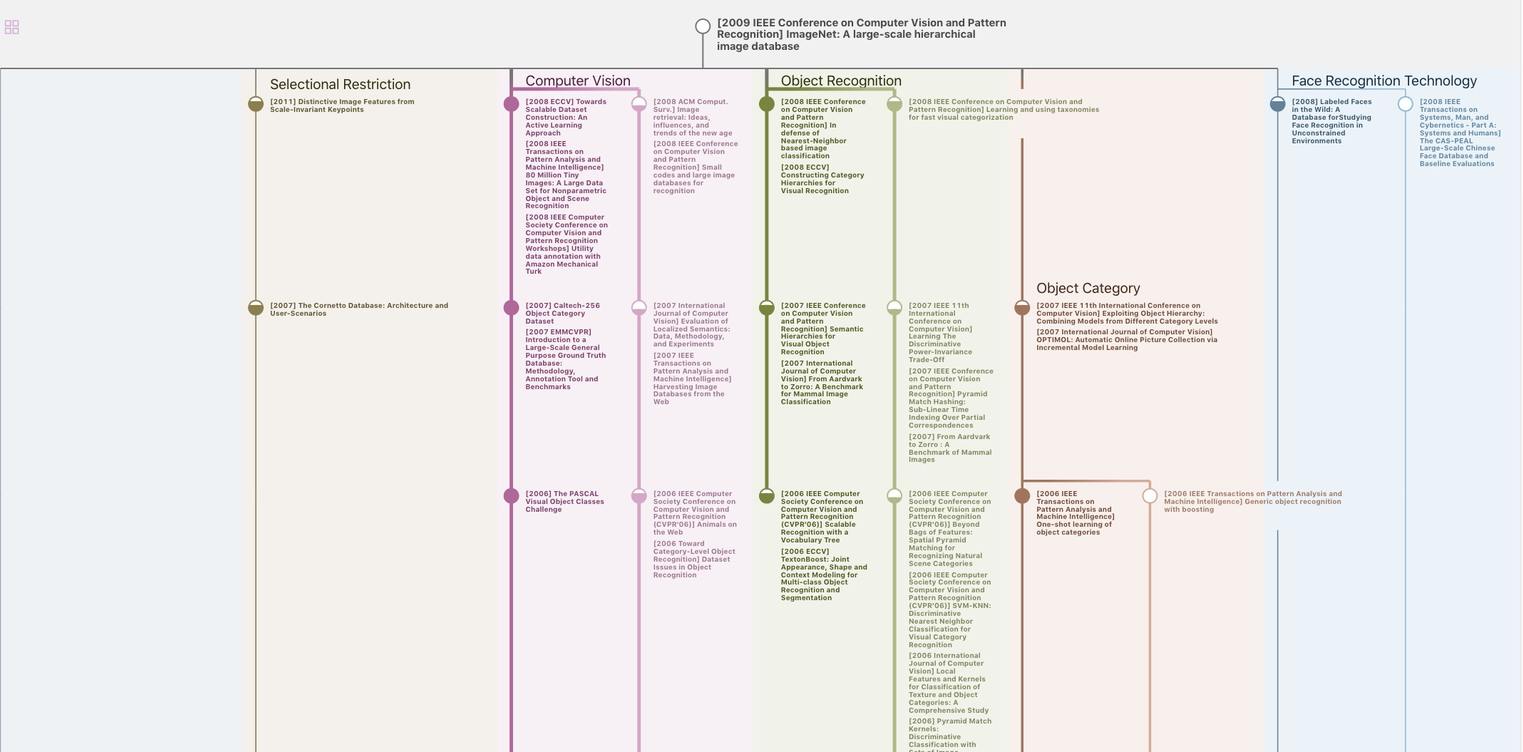
生成溯源树,研究论文发展脉络
Chat Paper
正在生成论文摘要