Jointly Learning Non-Cartesian k -Space Trajectories and Reconstruction Networks for 2D and 3D MR Imaging through Projection.
Bioengineering (Basel, Switzerland)(2023)
摘要
Compressed sensing in magnetic resonance imaging essentially involves the optimization of (1) the sampling pattern in -space under MR hardware constraints and (2) image reconstruction from undersampled -space data. Recently, deep learning methods have allowed the community to address both problems simultaneously, especially in the non-Cartesian acquisition setting. This work aims to contribute to this field by tackling some major concerns in existing approaches. Particularly, current state-of-the-art learning methods seek hardware compliant -space sampling trajectories by enforcing the hardware constraints through additional penalty terms in the training loss. Through ablation studies, we rather show the benefit of using a projection step to enforce these constraints and demonstrate that the resulting -space trajectories are more flexible under a projection-based scheme, which results in superior performance in reconstructed image quality. In 2D studies, our novel method trajectories present an improved image reconstruction quality at a 20-fold acceleration factor on the fastMRI data set with SSIM scores of nearly 0.92-0.95 in our retrospective studies as compared to the corresponding Cartesian reference and also see a 3-4 dB gain in PSNR as compared to earlier state-of-the-art methods. Finally, we extend the algorithm to 3D and by comparing optimization as learning-based projection schemes, we show that data-driven joint learning-based method trajectories outperform model-based methods such as SPARKLING through a 2 dB gain in PSNR and 0.02 gain in SSIM.
更多查看译文
关键词
MRI,k-space trajectories,non-Cartesian,reconstruction networks
AI 理解论文
溯源树
样例
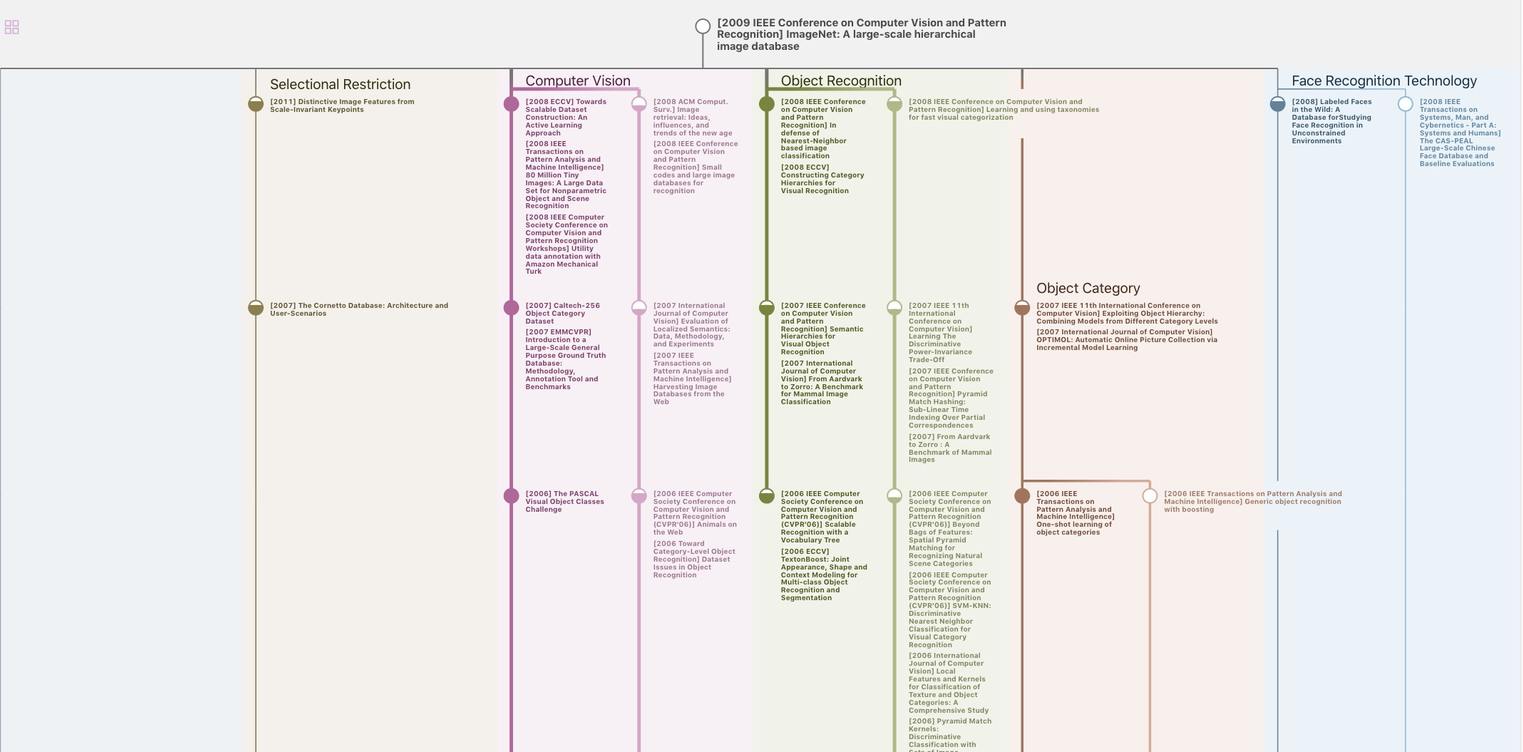
生成溯源树,研究论文发展脉络
Chat Paper
正在生成论文摘要