Dual Semi-Supervised Learning for Classification of Alzheimer's Disease and Mild Cognitive Impairment Based on Neuropsychological Data.
Brain sciences(2023)
摘要
Deep learning has shown impressive diagnostic abilities in Alzheimer's disease (AD) research in recent years. However, although neuropsychological tests play a crucial role in screening AD and mild cognitive impairment (MCI), there is still a lack of deep learning algorithms only using such basic diagnostic methods. This paper proposes a novel semi-supervised method using neuropsychological test scores and scarce labeled data, which introduces difference regularization and consistency regularization with pseudo-labeling. A total of 188 AD, 402 MCI, and 229 normal controls (NC) were enrolled in the study from the Alzheimer's Disease Neuroimaging Initiative (ADNI) database. We first chose the 15 features most associated with the diagnostic outcome by feature selection among the seven neuropsychological tests. Next, we proposed a dual semi-supervised learning (DSSL) framework that uses two encoders to learn two different feature vectors. The diagnosed 60 and 120 subjects were randomly selected as training labels for the model. The experimental results show that DSSL achieves the best accuracy and stability in classifying AD, MCI, and NC (85.47% accuracy for 60 labels and 88.40% accuracy for 120 labels) compared to other semi-supervised methods. DSSL is an excellent semi-supervised method to provide clinical insight for physicians to diagnose AD and MCI.
更多查看译文
关键词
Alzheimer’s disease,difference regularization,neuropsychological test,semi-supervised Learning
AI 理解论文
溯源树
样例
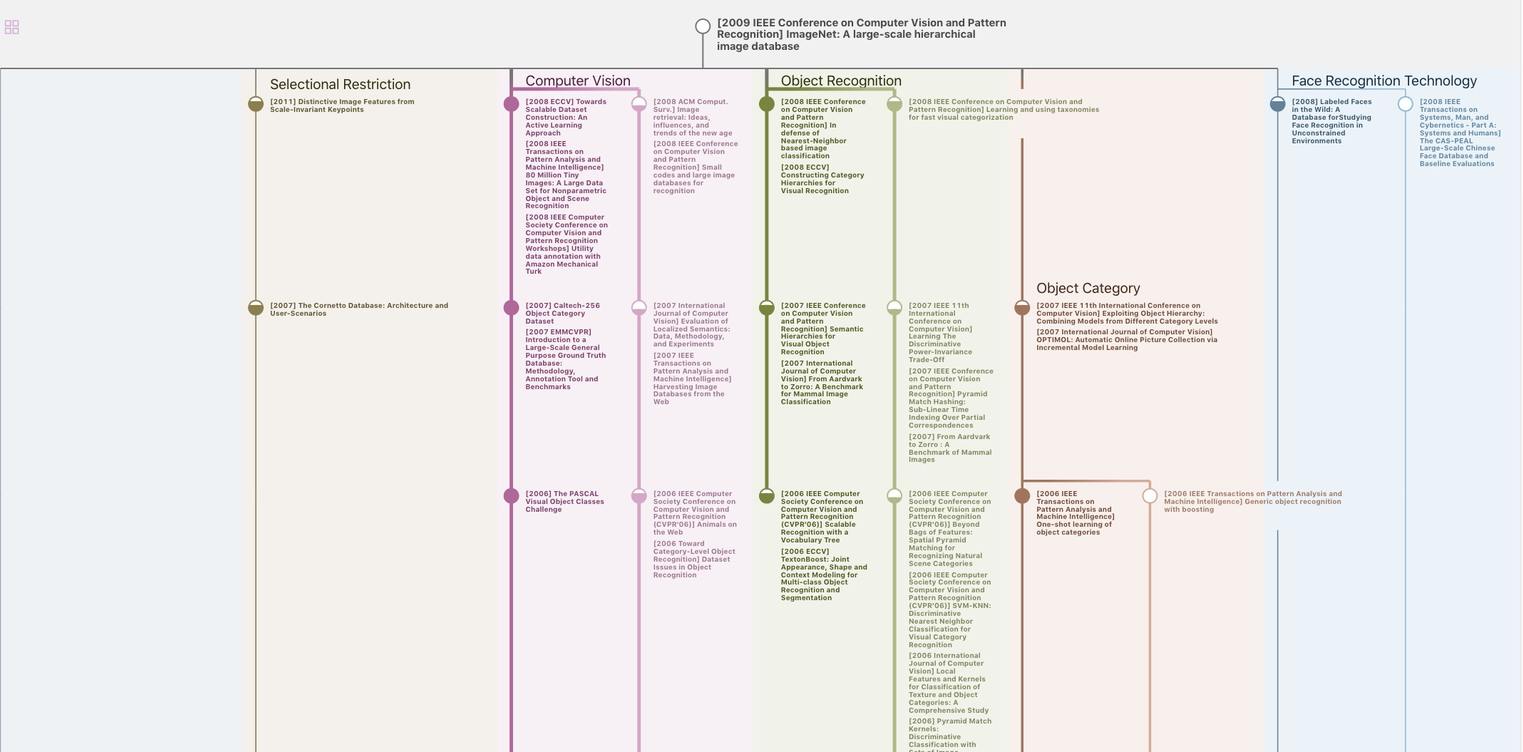
生成溯源树,研究论文发展脉络
Chat Paper
正在生成论文摘要