Tooth Type Enhanced Transformer for Children Caries Diagnosis on Dental Panoramic Radiographs.
Diagnostics (Basel, Switzerland)(2023)
摘要
The objective of this study was to introduce a novel deep learning technique for more accurate children caries diagnosis on dental panoramic radiographs. Specifically, a swin transformer is introduced, which is compared with the state-of-the-art convolutional neural network (CNN) methods that are widely used for caries diagnosis. A tooth type enhanced swin transformer is further proposed by considering the differences among canine, molar and incisor. Modeling the above differences in swin transformer, the proposed method was expected to mine domain knowledge for more accurate caries diagnosis. To test the proposed method, a children panoramic radiograph database was built and labeled with a total of 6028 teeth. Swin transformer shows better diagnosis performance compared with typical CNN methods, which indicates the usefulness of this new technique for children caries diagnosis on panoramic radiographs. Furthermore, the proposed tooth type enhanced swin transformer outperforms the naive swin transformer with the accuracy, precision, recall, F1 and area-under-the-curve being 0.8557, 0.8832, 0.8317, 0.8567 and 0.9223, respectively. This indicates that the transformer model can be further improved with a consideration of domain knowledge instead of a copy of previous transformer models designed for natural images. Finally, we compare the proposed tooth type enhanced swin transformer with two attending doctors. The proposed method shows higher caries diagnosis accuracy for the first and second primary molars, which may assist dentists in caries diagnosis.
更多查看译文
关键词
artificial intelligence,caries diagnosis,children,dental panoramic radiographs,transformer
AI 理解论文
溯源树
样例
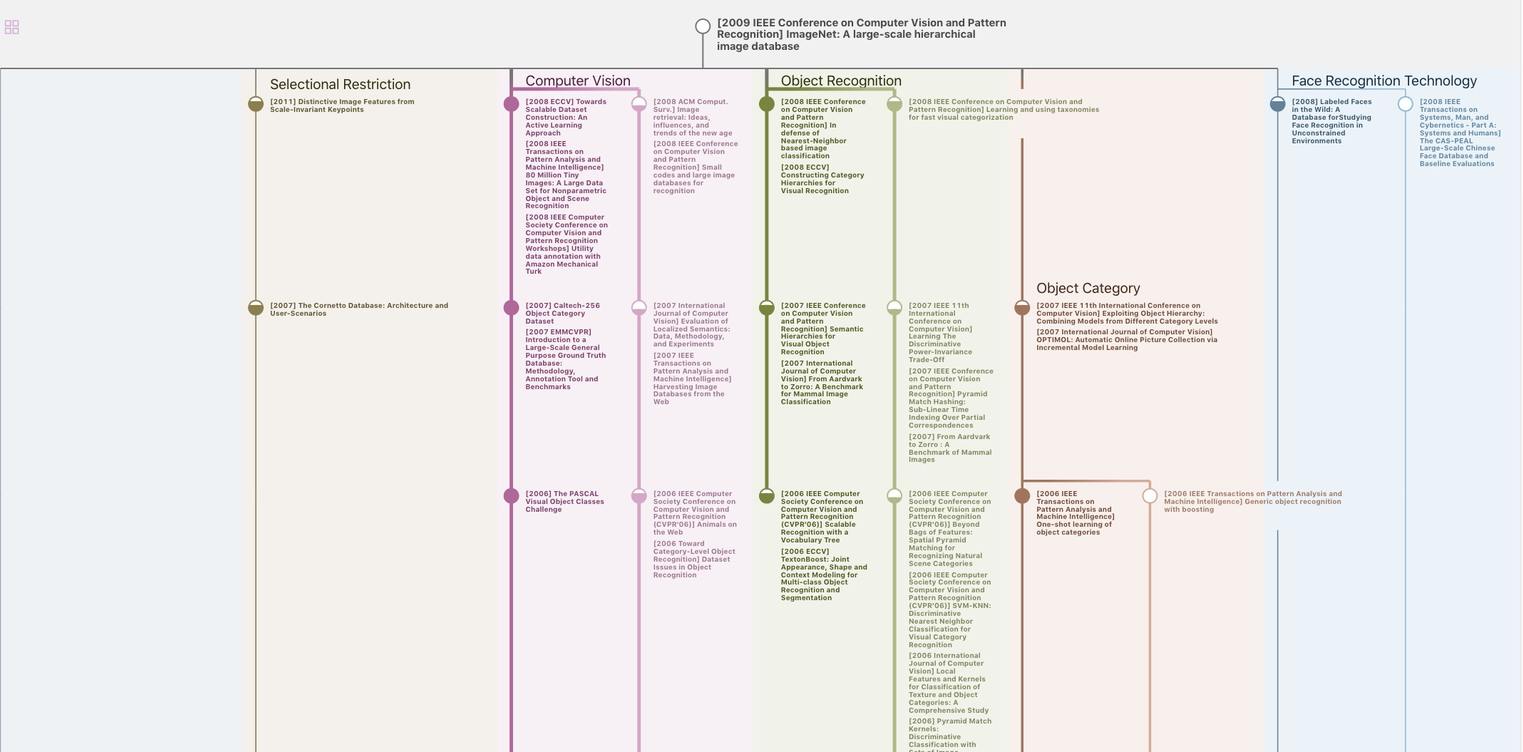
生成溯源树,研究论文发展脉络
Chat Paper
正在生成论文摘要