Improved Link Entropy with Dynamic Community Number Detection for Quantifying Significance of Edges in Complex Social Networks.
Entropy(2023)
摘要
Discovering communities in complex networks is essential in performing analyses, such as dynamics of political fragmentation and echo chambers in social networks. In this work, we study the problem of quantifying the significance of edges in a complex network, and propose a significantly improved version of the Link Entropy method. Using Louvain, Leiden and Walktrap methods, our proposal detects the number of communities in each iteration on discovering the communities. Running experiments on various benchmark networks, we show that our proposed method outperforms the Link Entropy method in quantifying edge significance. Considering also the computational complexities and possible defects, we conclude that Leiden or Louvain algorithms are the best choice for community number detection in quantifying edge significance. We also discuss designing a new algorithm for not only discovering the number of communities, but also computing the community membership uncertainties.
更多查看译文
关键词
social networks,edge significance,link entropy,deep link entropy,Leiden,Louvain,Walktrap
AI 理解论文
溯源树
样例
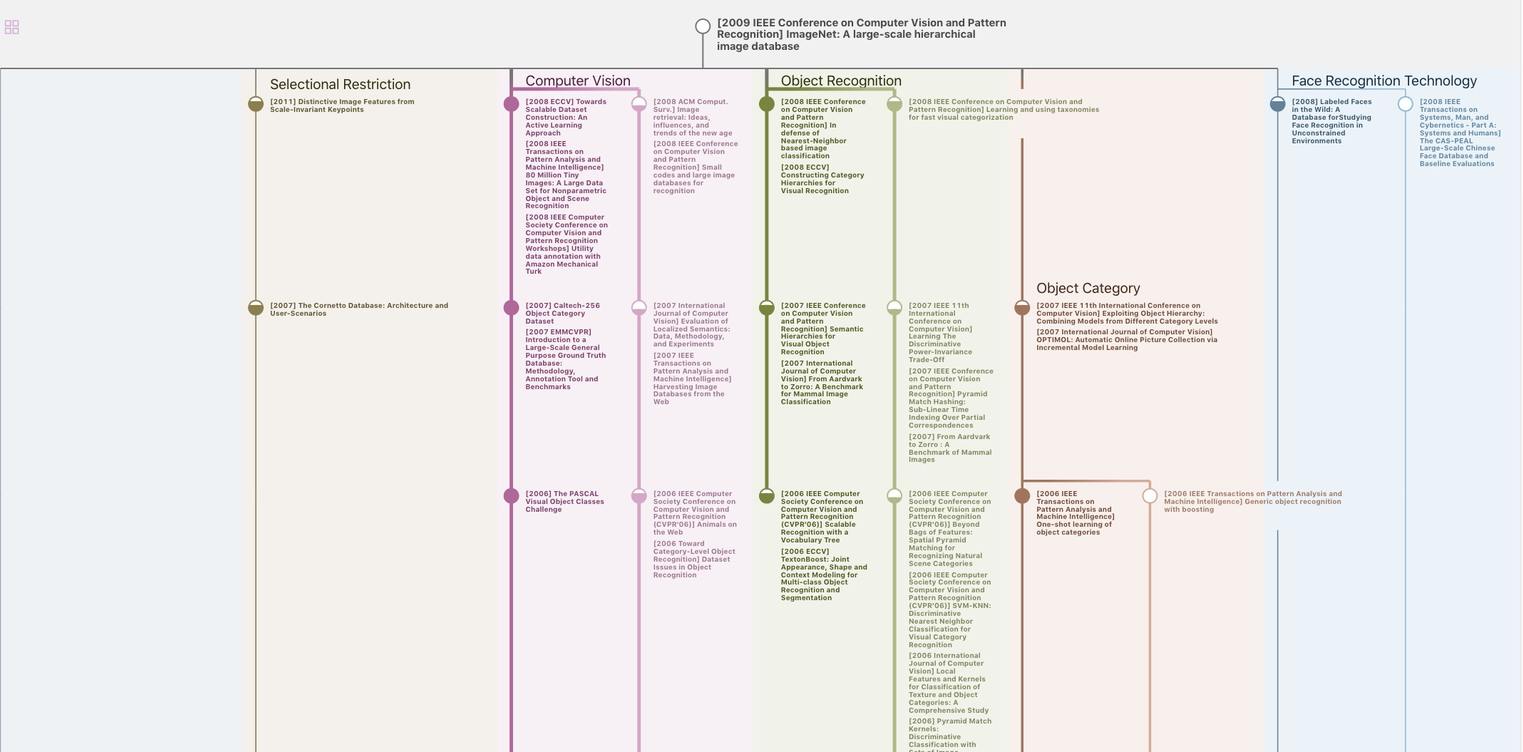
生成溯源树,研究论文发展脉络
Chat Paper
正在生成论文摘要