Alibaba HPN: A Data Center Network for Large Language Model Training
SIGCOMM 2024(2024)
Abstract
This paper presents HPN, Alibaba Cloud's data center network for large language model (LLM) training. Due to the differences between LLMs and general cloud computing (e.g., in terms of traffic patterns and fault tolerance), traditional data center networks are not well-suited for LLM training. LLM training produces a small number of periodic, bursty flows (e.g., 400Gbps) on each host. This characteristic of LLM training predisposes Equal-Cost Multi-Path (ECMP) to hash polarization, causing issues such as uneven traffic distribution. HPN introduces a 2-tier, dual-plane architecture capable of interconnecting 15K GPUs within one Pod, typically accommodated by the traditional 3-tier Clos architecture. Such a new architecture design not only avoids hash polarization but also greatly reduces the search space for path selection. Another challenge in LLM training is that its requirement for GPUs to complete iterations in synchronization makes it more sensitive to singlepoint failure (typically occurring on ToR). HPN proposes a new dual-ToR design to replace the single-ToR in traditional data center networks. HPN has been deployed in our production for more than eight months. We share our experience in designing, and building HPN, as well as the operational lessons of HPN in production.
MoreTranslated text
AI Read Science
Must-Reading Tree
Example
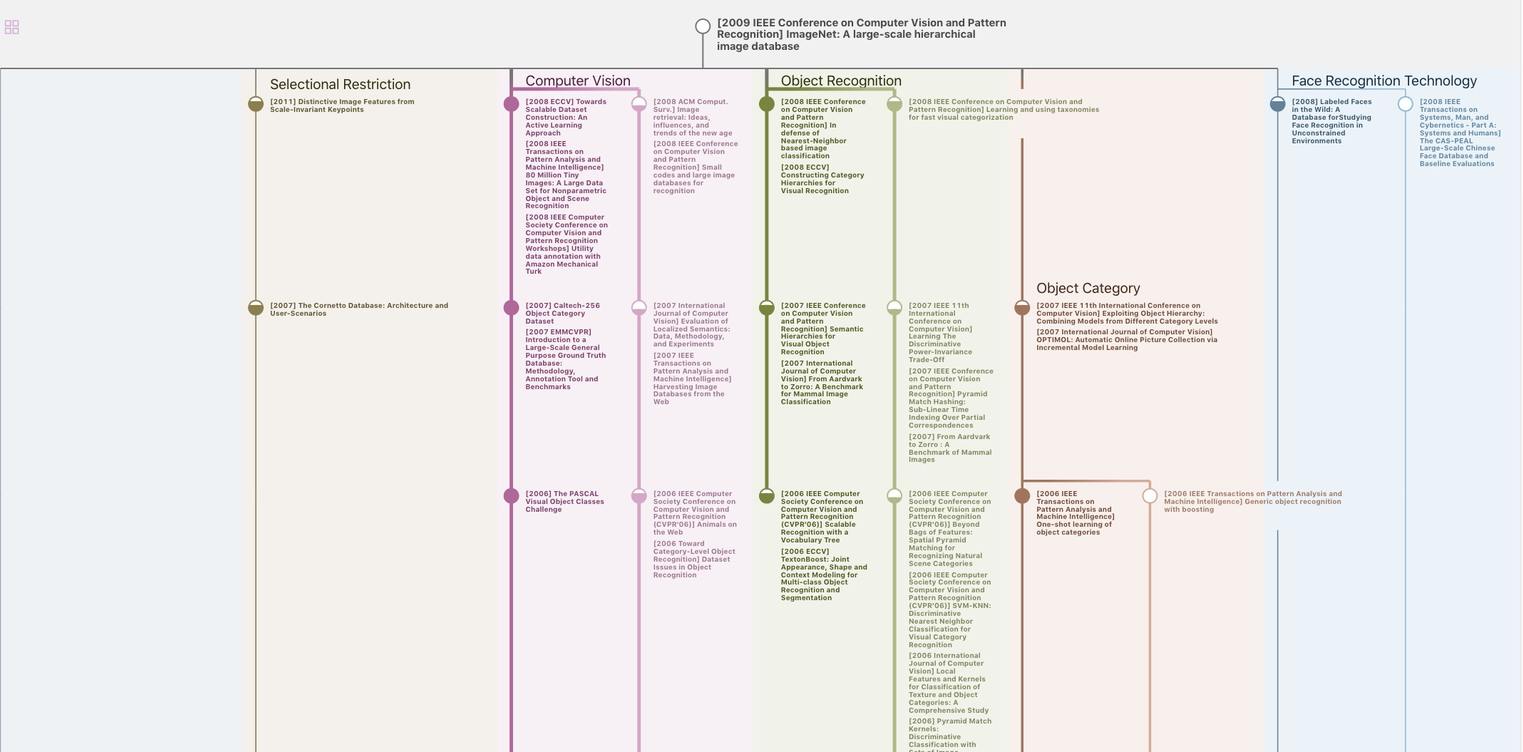
Generate MRT to find the research sequence of this paper
Chat Paper
Summary is being generated by the instructions you defined