A generalizable Cas9/sgRNA prediction model using machine transfer learning with small high-quality datasets
biorxiv(2023)
摘要
The CRISPR/Cas9 nuclease from Streptococcus pyogenes (SpCas9) can be used with single guide RNAs (sgRNAs) as a sequence-specific antimicrobial agent and as a genome-engineering tool. However, current bacterial sgRNA activity models struggle with accurate predictions and do not generalize well, possibly because the underlying datasets used to train the models do not accurately measure SpCas9/sgRNA activity and cannot distinguish on-target cleavage from toxicity. Here, we solve this problem by using a two-plasmid positive selection system to generate high-quality data that more accurately reports on SpCas9/sgRNA cleavage and that separates activity from toxicity. We develop a machine learning architecture (crisprHAL) that can be trained on existing datasets, that shows marked improvements in sgRNA activity prediction accuracy when transfer learning is used with small amounts of high-quality data, and that can generalize predictions to different bacteria. The crisprHAL model recapitulates known SpCas9/sgRNA-target DNA interactions and provides a pathway to a generalizable sgRNA bacterial activity prediction tool that will enable accurate antimicrobial and genome engineering applications.
更多查看译文
关键词
cas9/sgrna prediction model,generalizable cas9/sgrna,transfer learning,high-quality
AI 理解论文
溯源树
样例
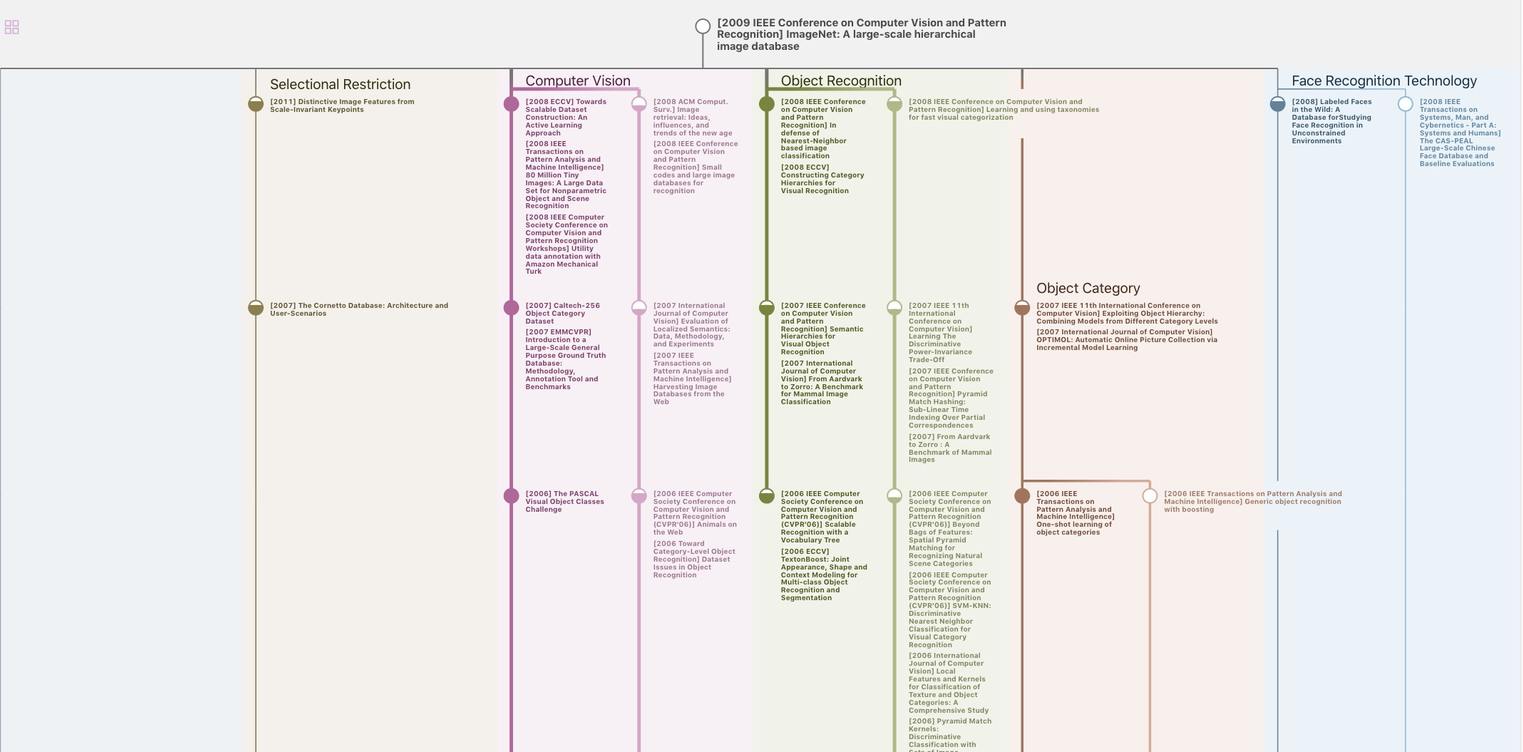
生成溯源树,研究论文发展脉络
Chat Paper
正在生成论文摘要