Design-Based Inference for Multi-arm Bandits
arXiv (Cornell University)(2023)
摘要
Multi-arm bandits are gaining popularity as they enable real-world sequential decision-making across application areas, including clinical trials, recommender systems, and online decision-making. Consequently, there is an increased desire to use the available adaptively collected datasets to distinguish whether one arm was more effective than the other, e.g., which product or treatment was more effective. Unfortunately, existing tools fail to provide valid inference when data is collected adaptively or require many untestable and technical assumptions, e.g., stationarity, iid rewards, bounded random variables, etc. Our paper introduces the design-based approach to inference for multi-arm bandits, where we condition the full set of potential outcomes and perform inference on the obtained sample. Our paper constructs valid confidence intervals for both the reward mean of any arm and the mean reward difference between any arms in an assumption-light manner, allowing the rewards to be arbitrarily distributed, non-iid, and from non-stationary distributions. In addition to confidence intervals, we also provide valid design-based confidence sequences, sequences of confidence intervals that have uniform type-1 error guarantees over time. Confidence sequences allow the agent to perform a hypothesis test as the data arrives sequentially and stop the experiment as soon as the agent is satisfied with the inference, e.g., the mean reward of an arm is statistically significantly higher than a desired threshold.
更多查看译文
关键词
inference,design-based,multi-arm
AI 理解论文
溯源树
样例
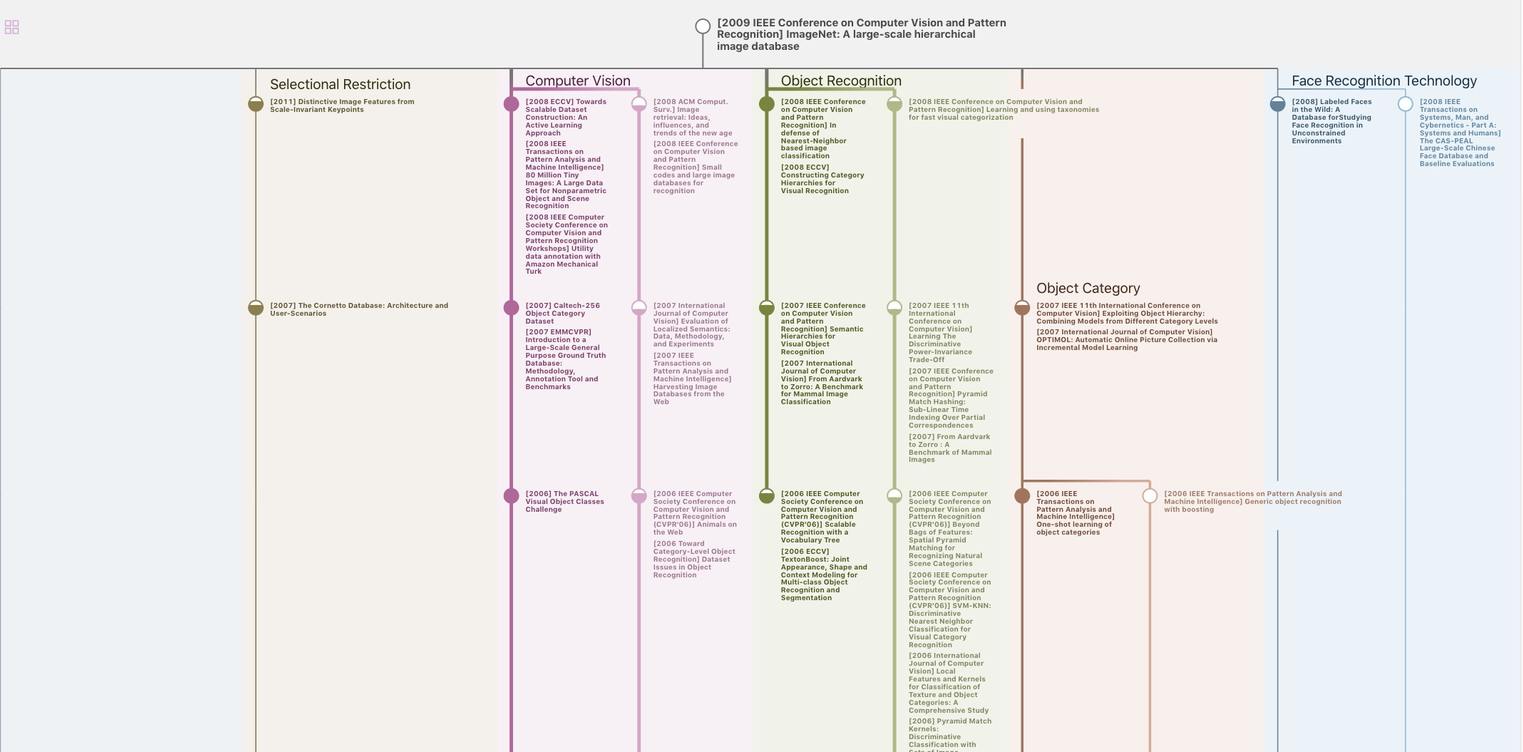
生成溯源树,研究论文发展脉络
Chat Paper
正在生成论文摘要