Learning Sparse Control Tasks from Pixels by Latent Nearest-Neighbor-Guided Explorations
CoRR(2023)
Abstract
Recent progress in deep reinforcement learning (RL) and computer vision enables artificial agents to solve complex tasks, including locomotion, manipulation and video games from high-dimensional pixel observations. However, domain specific reward functions are often engineered to provide sufficient learning signals, requiring expert knowledge. While it is possible to train vision-based RL agents using only sparse rewards, additional challenges in exploration arise. We present a novel and efficient method to solve sparse-reward robot manipulation tasks from only image observations by utilizing a few demonstrations. First, we learn an embedded neural dynamics model from demonstration transitions and further fine-tune it with the replay buffer. Next, we reward the agents for staying close to the demonstrated trajectories using a distance metric defined in the embedding space. Finally, we use an off-policy, model-free vision RL algorithm to update the control policies. Our method achieves state-of-the-art sample efficiency in simulation and enables efficient training of a real Franka Emika Panda manipulator.
MoreTranslated text
Key words
sparse control tasks,explorations,learning,nearest-neighbor-guided
AI Read Science
Must-Reading Tree
Example
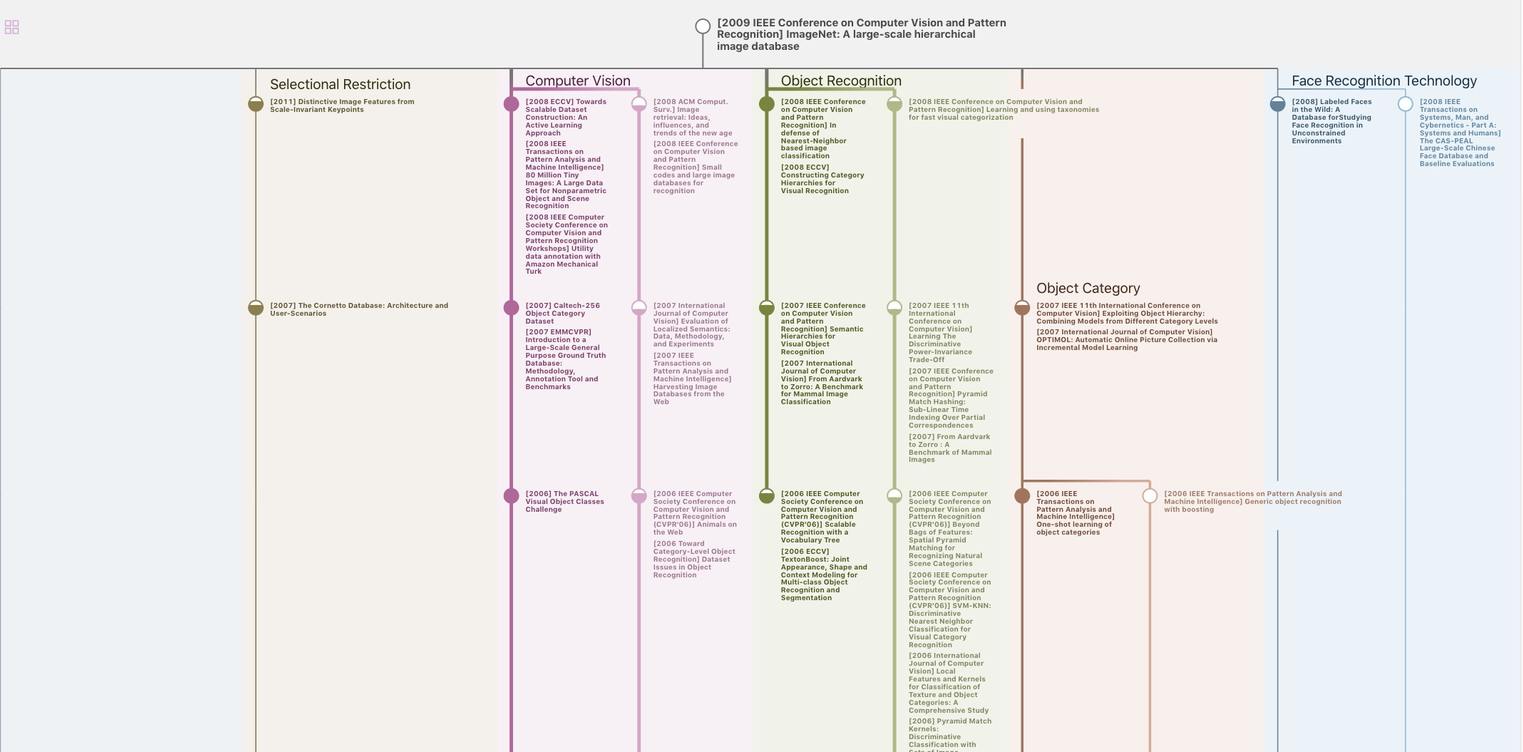
Generate MRT to find the research sequence of this paper
Chat Paper
Summary is being generated by the instructions you defined