Information and Criticality in Complex Stochastic Systems
arxiv(2023)
摘要
This Thesis explores how tools from Statistical Physics and Information Theory can help us describe and understand complex systems. In the first part, we study the interplay between internal interactions, environmental changes, and effective representations of complex stochastic systems. We model the environment as an unobserved stochastic process and investigate how the mutual information between internal degrees of freedom encodes internal and environmental processes, and how it can help us disentangle them. Then, we attempt to build effective representations via information-preserving projections that preserve the dependencies of a complex system. In the paradigmatic case of underdamped systems, we find that optimal effective representations may be unexpectedly singular, revealing fundamental limits of approximating complex models. In the second part of this Thesis, we apply these approaches and ideas from criticality to neural systems at different scales, showing that unobserved neural activity may lead to power-law neuronal avalanches in the absence of criticality. Remarkably, the properties of mutual information suggest that, whereas avalanches may emerge from stochastic modulation, interactions between neural populations are at the source of scale-free correlations. We further explore the role of the structure of interactions by showing how interaction networks change the nature of the phase transition of a well-known cellular automaton. Finally, we test a phenomenological coarse-graining procedure for neural timeseries in different settings, and in particular in the presence of stochastic environments. Our results shed light on the role of the interaction network, its topological features, and unobserved modulation in the emergence of collective patterns of brain activity and general complex systems.
更多查看译文
关键词
criticality,information,complex,systems
AI 理解论文
溯源树
样例
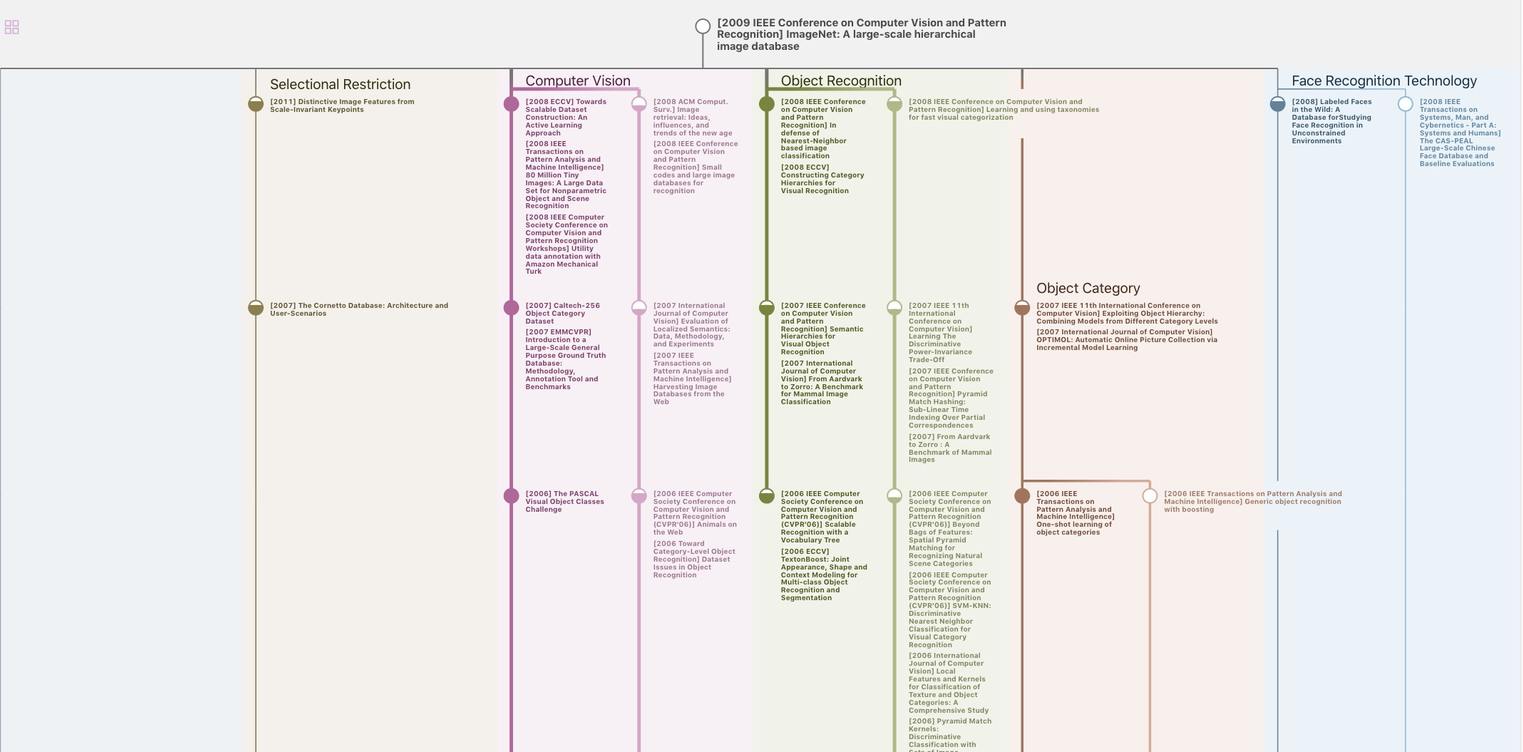
生成溯源树,研究论文发展脉络
Chat Paper
正在生成论文摘要