EEG-Based Emotion Identification Using 1-D Deep Residual Shrinkage Network With Microstate Features
IEEE Sensors Journal(2023)
摘要
Previous studies on emotion identification from electroencephalogram (EEG) mostly focused on normal and depressed people. However, hearing-impaired subjects may require emotional identification due to their chronic lack of perception of auditory information. In this article, we designed an experiment to collect EEG signals from 15 hearing-impaired subjects when they are watching the four kinds of emotional movie clips (happiness, calmness, sadness, and fear). The novel
${K}$
-means method is used to extract the ten kinds of microstates (as A, B, C, D, E, F, G, H, I, and J) from the raw EEG signal, and then the new EEG single will be retrofitted by those ten microstates. For feature extraction, six kinds of microstate features (global explained variance (GEV), GEV total (GEVT), global field power (GFP), coverage, duration, and occurrence) are calculated. To classify the microstate features, a 1-D deep residual shrinkage network (1-D-DRSN) is utilized, which can filter the emotional irrelevant noise information, and capture emotional representational information. Experimental results show that the proposed model can significantly improve performance compared with other machine learning methods, with an average accuracy of 87.48%. Moreover, we explore different combinations of microstate features to reduce redundant information, and the combination of occurrence, GEV, and coverage reaches 90.15%. From the exploration of each microstate, we find that microstate C has the advantage with an average accuracy of 49.07%.
更多查看译文
关键词
Deep learning,electroencephalogram (EEG),emotion identification,hearing-impaired subjects,microstate features
AI 理解论文
溯源树
样例
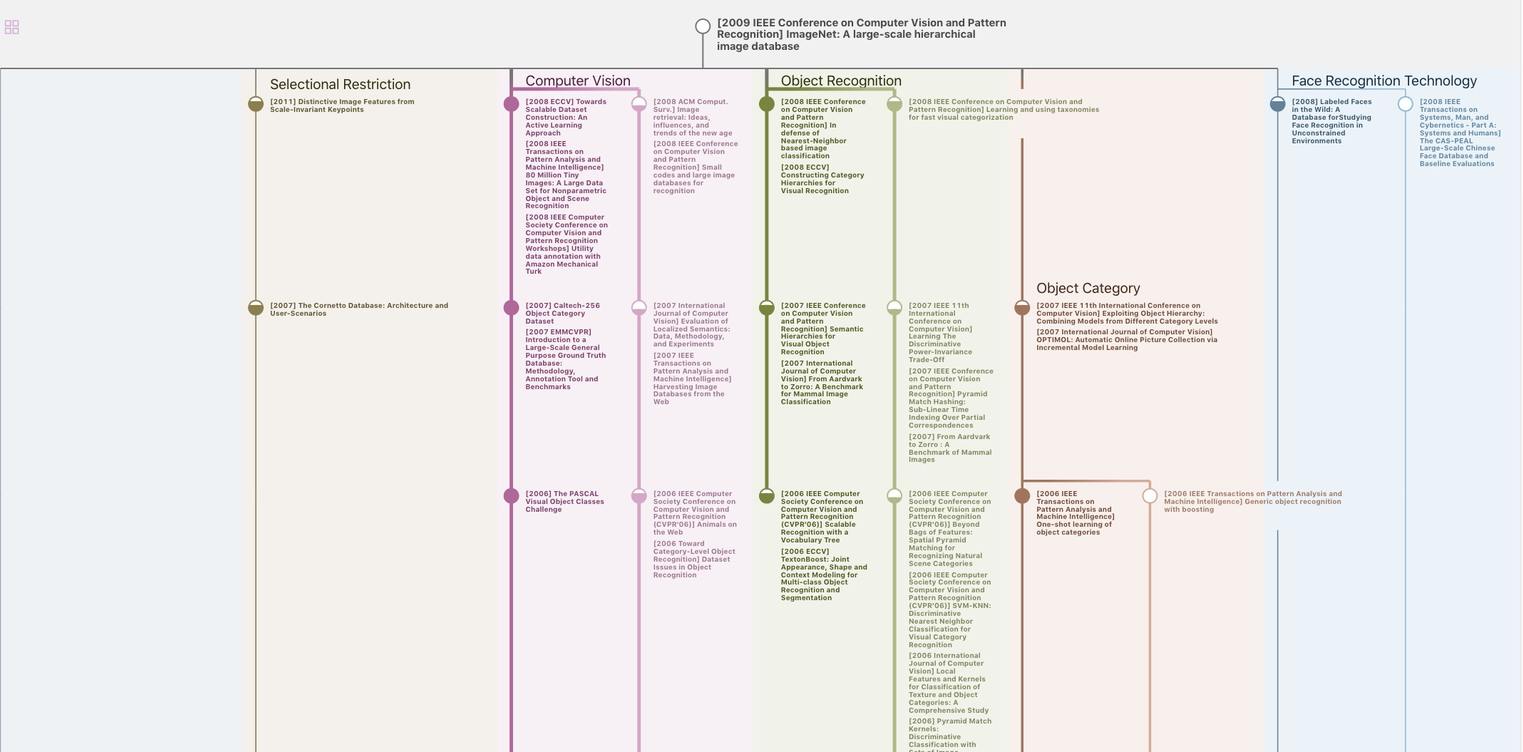
生成溯源树,研究论文发展脉络
Chat Paper
正在生成论文摘要