Speeding Up EfficientNet: Selecting Update Blocks of Convolutional Neural Networks Using Genetic Algorithm in Transfer Learning
arXivorg(2023)
摘要
The performance of convolutional neural networks (CNN) depends heavily on their architectures. Transfer learning performance of a CNN relies quite strongly on selection of its trainable layers. Selecting the most effective update layers for a certain target dataset often requires expert knowledge on CNN architecture which many practitioners do not posses. General users prefer to use an available architecture (e.g. GoogleNet, ResNet, EfficientNet etc.) that is developed by domain experts. With the ever-growing number of layers, it is increasingly becoming quite difficult and cumbersome to handpick the update layers. Therefore, in this paper we explore the application of genetic algorithm to mitigate this problem. The convolutional layers of popular pretrained networks are often grouped into modules that constitute their building blocks. We devise a genetic algorithm to select blocks of layers for updating the parameters. By experimenting with EfficientNetB0 pre-trained on ImageNet and using Food-101, CIFAR-100 and MangoLeafBD as target datasets, we show that our algorithm yields similar or better results than the baseline in terms of accuracy, and requires lower training and evaluation time due to learning less number of parameters. We also devise a metric called block importance to measure efficacy of each block as update block and analyze the importance of the blocks selected by our algorithm.
更多查看译文
关键词
Transfer Learning,Robust Learning,Neural Network Architectures,Meta-Learning,Deep Learning
AI 理解论文
溯源树
样例
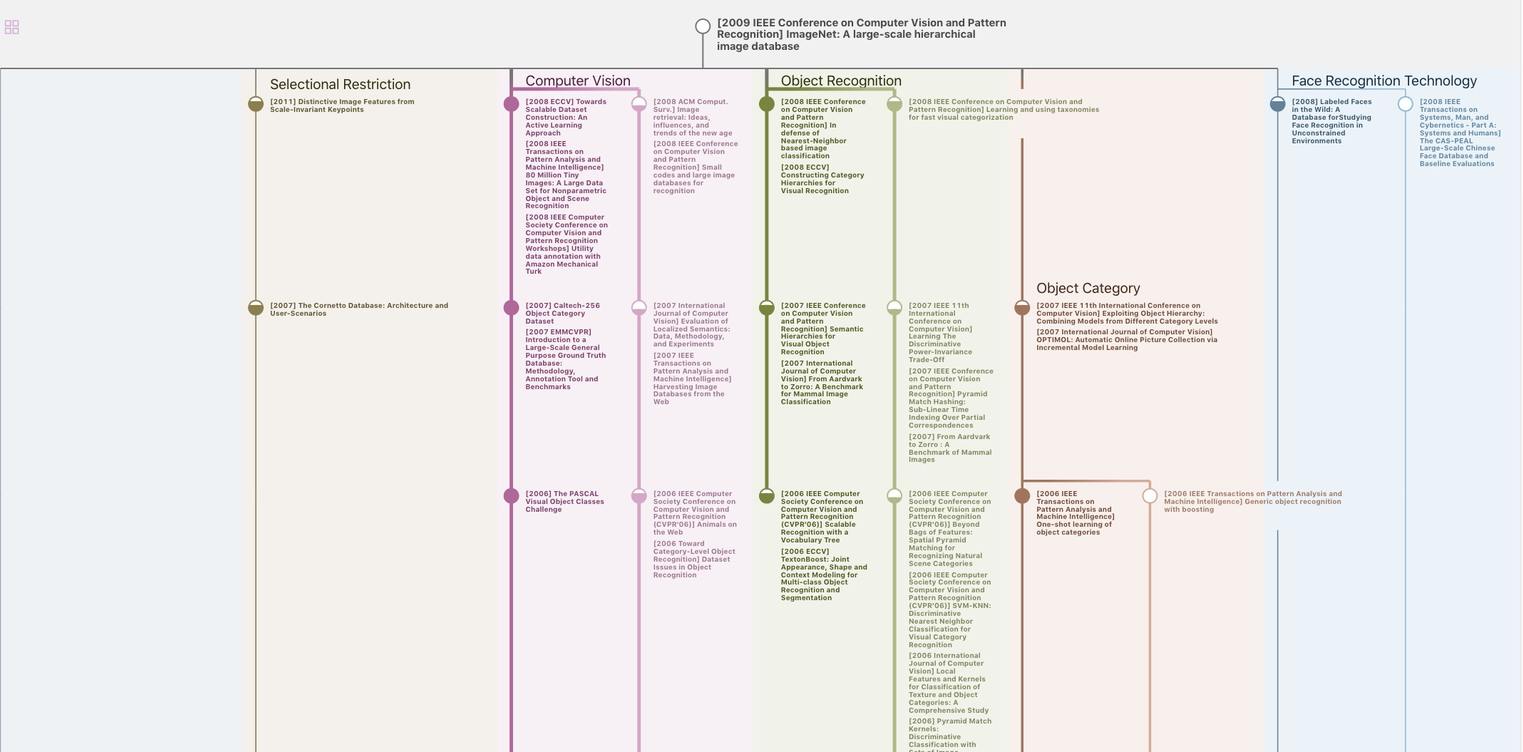
生成溯源树,研究论文发展脉络
Chat Paper
正在生成论文摘要