FedScore: A privacy-preserving framework for federated scoring system development
Journal of biomedical informatics(2023)
摘要
We propose FedScore, a privacy-preserving federated learning framework for scoring system generation across multiple sites to facilitate cross-institutional collaborations. The FedScore framework includes five modules: federated variable ranking, federated variable transformation, federated score derivation, federated model selection and federated model evaluation. To illustrate usage and assess FedScore's performance, we built a hypothetical global scoring system for mortality prediction within 30 days after a visit to an emergency department using 10 simulated sites divided from a tertiary hospital in Singapore. We employed a pre-existing score generator to construct 10 local scoring systems independently at each site and we also developed a scoring system using centralized data for comparison. We compared the acquired FedScore model's performance with that of other scoring models using the receiver operating characteristic (ROC) analysis. The FedScore model achieved an average area under the curve (AUC) value of 0.763 across all sites, with a standard deviation (SD) of 0.020. We also calculated the average AUC values and SDs for each local model, and the FedScore model showed promising accuracy and stability with a high average AUC value which was closest to the one of the pooled model and SD which was lower than that of most local models. This study demonstrates that FedScore is a privacy-preserving scoring system generator with potentially good generalizability.
更多查看译文
关键词
Clinical decision making,Distributed algorithm,Electronic health record,Electronic medical record,Federated learning,Scoring system
AI 理解论文
溯源树
样例
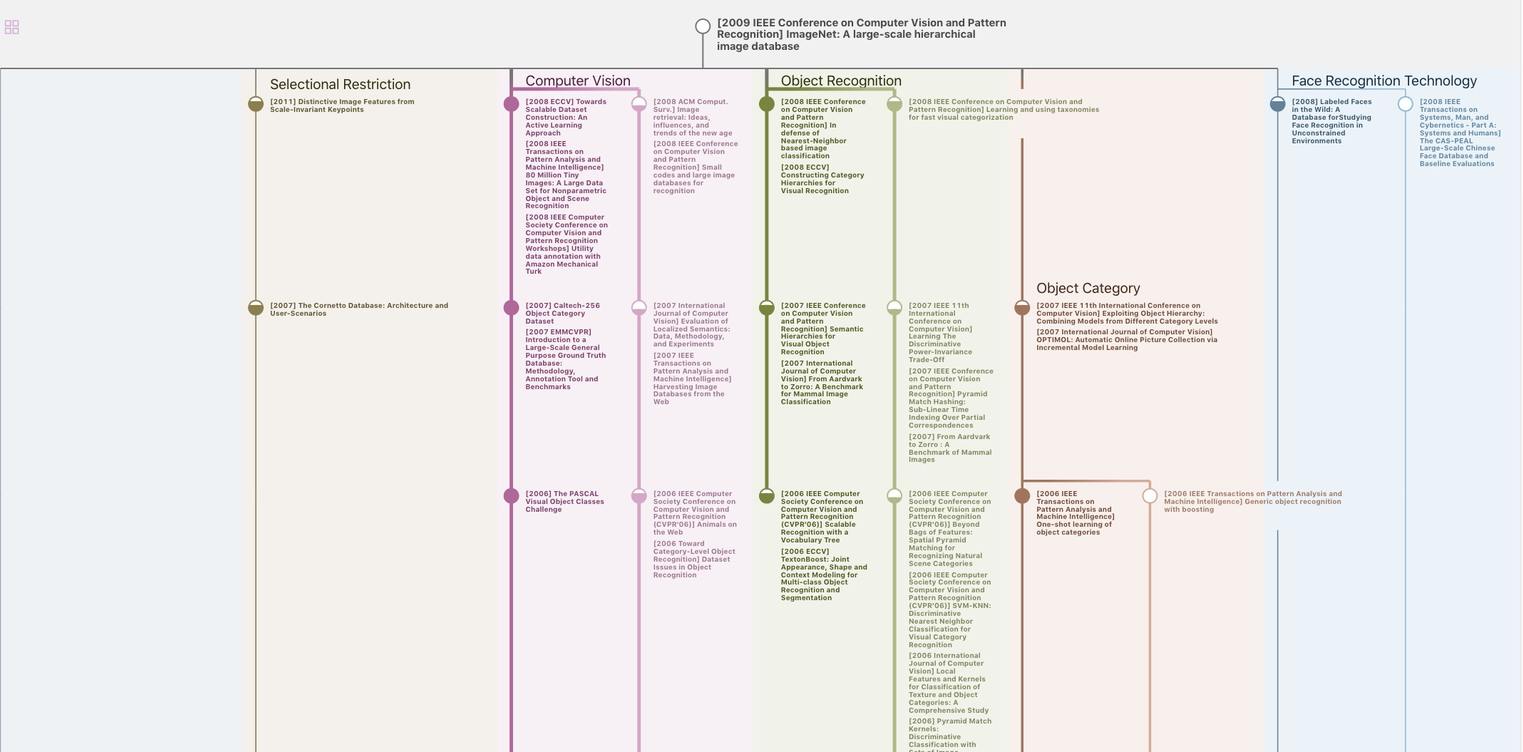
生成溯源树,研究论文发展脉络
Chat Paper
正在生成论文摘要