Application of Deep Learning Methods for Distinguishing Gamma-Ray Bursts from Fermi/GBM TTE Data
arXiv (Cornell University)(2023)
摘要
To investigate GRBs in depth, it is crucial to develop an effective method
for identifying GRBs accurately. Current criteria, e.g., onboard blind search,
ground blind search, and target search, are limited by manually set thresholds
and perhaps miss GRBs, especially for sub-threshold events. We propose a novel
approach that utilizes convolutional neural networks (CNNs) to distinguish GRBs
and non-GRBs directly. We structured three CNN models, plain-CNN, ResNet, and
ResNet-CBAM, and endeavored to exercise fusing strategy models. Count maps of
NaI detectors onboard Fermi/GBM were employed as the input samples of datasets
and models were implemented to evaluate their performance on different time
scale data. The ResNet-CBAM model trained on 64 ms dataset achieves high
accuracy overall, which includes residual and attention mechanism modules. The
visualization methods of Grad-CAM and t-SNE explicitly displayed that the
optimal model focuses on the key features of GRBs precisely. The model was
applied to analyze one-year data, accurately identifying approximately 98
GRBs listed in the Fermi burst catalog, 8 out of 9 sub-threshold GRBs, and 5
GRBs triggered by other satellites, which demonstrated the deep learning
methods could effectively distinguish GRBs from observational data. Besides,
thousands of unknown candidates were retrieved and compared with the bursts of
SGR J1935+2154 for instance, which exemplified the potential scientific value
of these candidates indeed. Detailed studies on integrating our model into
real-time analysis pipelines thus may improve their accuracy of inspection, and
provide valuable guidance for rapid follow-up observations of multi-band
telescopes.
更多查看译文
关键词
deep learning methods,deep learning,fermi/gbm,gamma-ray
AI 理解论文
溯源树
样例
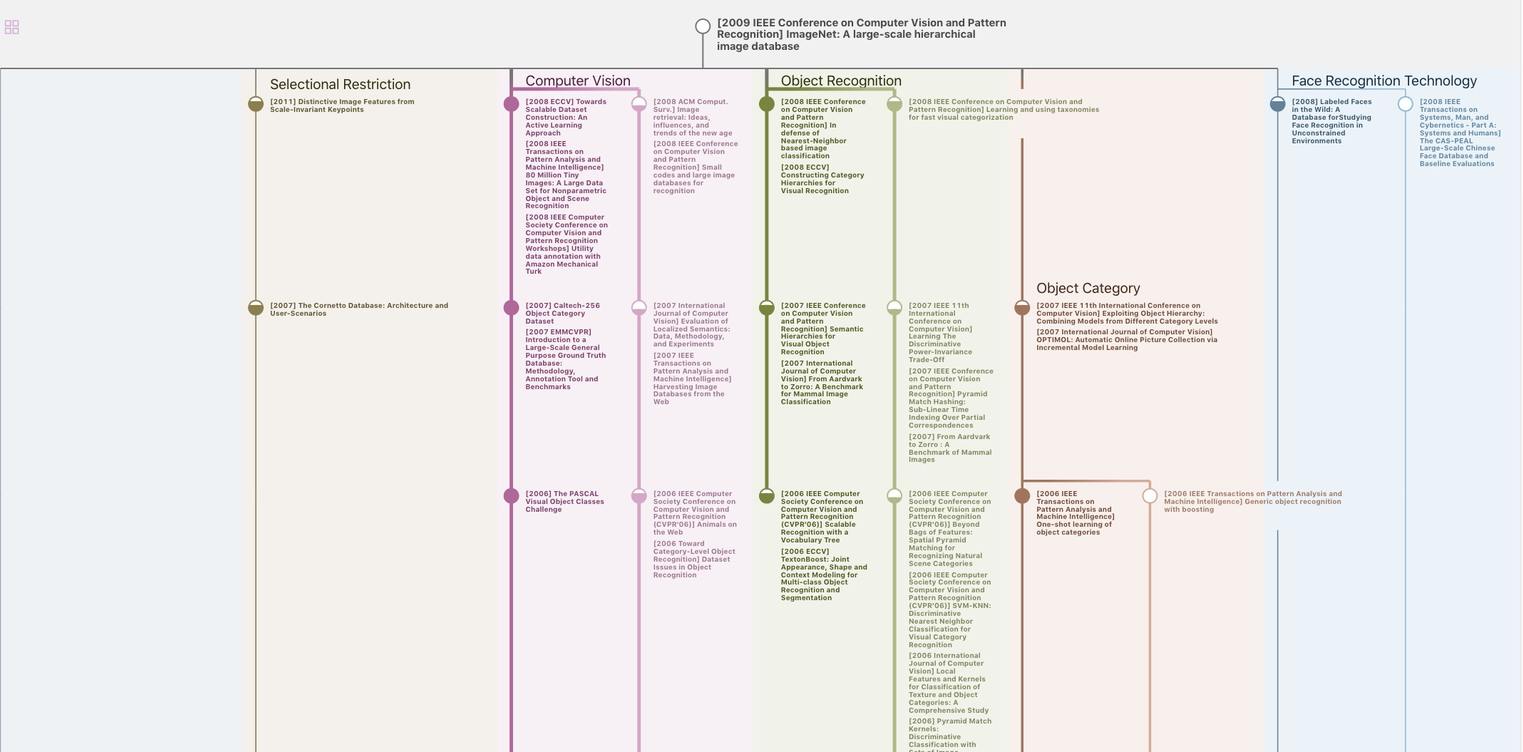
生成溯源树,研究论文发展脉络
Chat Paper
正在生成论文摘要