Semi-Supervised Constrained Clustering: An In-Depth Overview, Ranked Taxonomy and Future Research Directions
arxiv(2023)
摘要
Clustering is a well-known unsupervised machine learning approach capable of automatically grouping discrete sets of instances with similar characteristics. Constrained clustering is a semi-supervised extension to this process that can be used when expert knowledge is available to indicate constraints that can be exploited. Well-known examples of such constraints are must-link (indicating that two instances belong to the same group) and cannot-link (two instances definitely do not belong together). The research area of constrained clustering has grown significantly over the years with a large variety of new algorithms and more advanced types of constraints being proposed. However, no unifying overview is available to easily understand the wide variety of available methods, constraints and benchmarks. To remedy this, this study presents in-detail the background of constrained clustering and provides a novel ranked taxonomy of the types of constraints that can be used in constrained clustering. In addition, it focuses on the instance-level pairwise constraints, and gives an overview of its applications and its historical context. Finally, it presents a statistical analysis covering 307 constrained clustering methods, categorizes them according to their features, and provides a ranking score indicating which methods have the most potential based on their popularity and validation quality. Finally, based upon this analysis, potential pitfalls and future research directions are provided.
更多查看译文
关键词
clustering,taxonomy,semi-supervised,in-depth
AI 理解论文
溯源树
样例
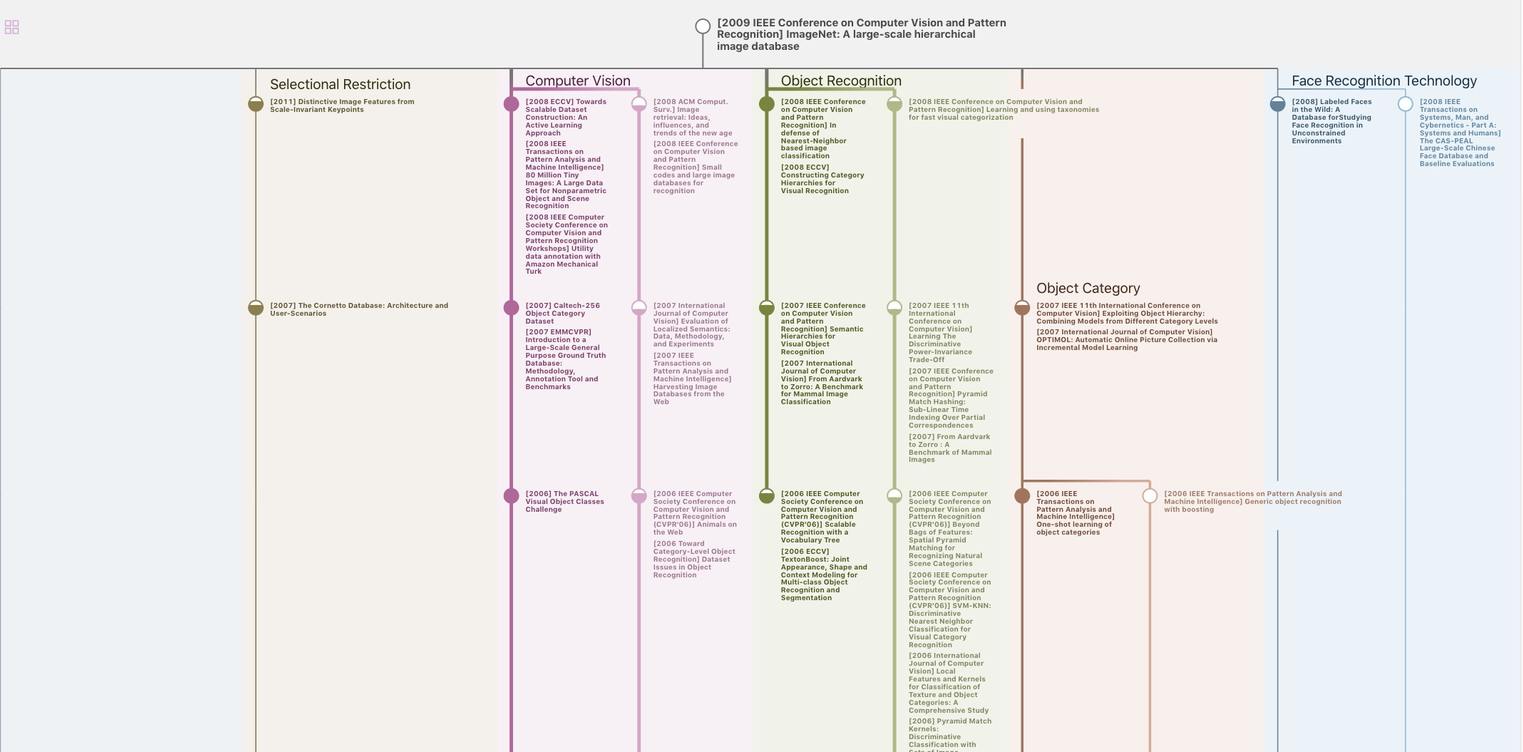
生成溯源树,研究论文发展脉络
Chat Paper
正在生成论文摘要