Squeezing More Past Knowledge for Online Class-Incremental Continual Learning
IEEE/CAA Journal of Automatica Sinica(2023)
摘要
Continual learning (CL) studies the problem of learning to accumulate knowledge over time from a stream of data. A crucial challenge is that neural networks suffer from performance degradation on previously seen data, known as catastrophic forgetting, due to allowing parameter sharing. In this work, we consider a more practical online class-incremental CL setting, where the model learns new samples in an online manner and may continuously experience new classes. Moreover, prior knowledge is unavailable during training and evaluation. Existing works usually explore sample usages from a single dimension, which ignores a lot of valuable supervisory information. To better tackle the setting, we propose a novel replay-based CL method, which leverages multi-level representations produced by the intermediate process of training samples for replay and strengthens supervision to consolidate previous knowledge. Specifically, besides the previous raw samples, we store the corresponding logits and features in the memory. Furthermore, to imitate the prediction of the past model, we construct extra constraints by leveraging multi-level information stored in the memory. With the same number of samples for replay, our method can use more past knowledge to prevent interference. We conduct extensive evaluations on several popular CL datasets, and experiments show that our method consistently outperforms state-of-the-art methods with various sizes of episodic memory. We further provide a detailed analysis of these results and demonstrate that our method is more viable in practical scenarios.
更多查看译文
关键词
Catastrophic forgetting,class-incremental learning,continual learning (CL),experience replay
AI 理解论文
溯源树
样例
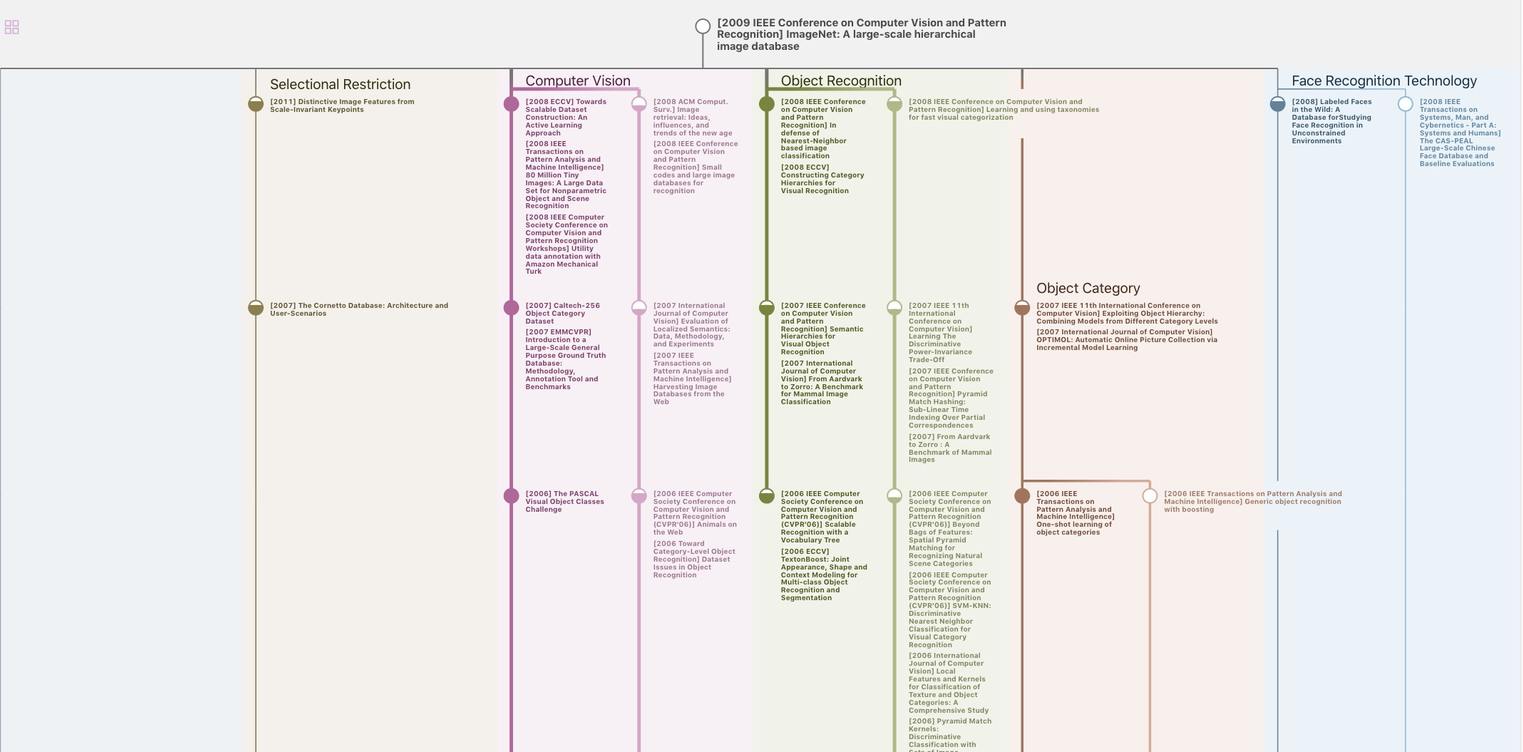
生成溯源树,研究论文发展脉络
Chat Paper
正在生成论文摘要