FHSINet: A hybrid model for the analysis of hyperspectral images
2022 Seventh International Conference on Parallel, Distributed and Grid Computing (PDGC)(2022)
摘要
Hyperspectral images (HSI) contain a rich set of high-dimensional features. Although these features are widely used for land cover classification. However, extraction of spectral and special features is difficult due to several bands of HSI. In the proposed study, a novel hybrid deep convolution model FHSINet (Fusion Hyperspectral Image Network) efficiently extracts features from HSI. This model has two scale feature extraction modules, each with 4 convolutions layers followed by feature fusion and classification blocks. At each scale, spatial features are extracted from HSI. After that, these features are fused to form a pool of features. We investigated the performance of HFSINet on two datasets. It achieved competitive performance compared to state-of-the-art models. Our model classification accuracy on the Pavia University dataset and Pavia Centre scenes are 94.40% and 98.72% rest reactively. In addition, the Kappa score is more than 98% on the Pavia centre scene dataset. Furthermore, the training and validation loss of the proposed model is less compared to state-of-the-art models.
更多查看译文
关键词
Hyperspectral image,Remote sensing,hybrid,classification
AI 理解论文
溯源树
样例
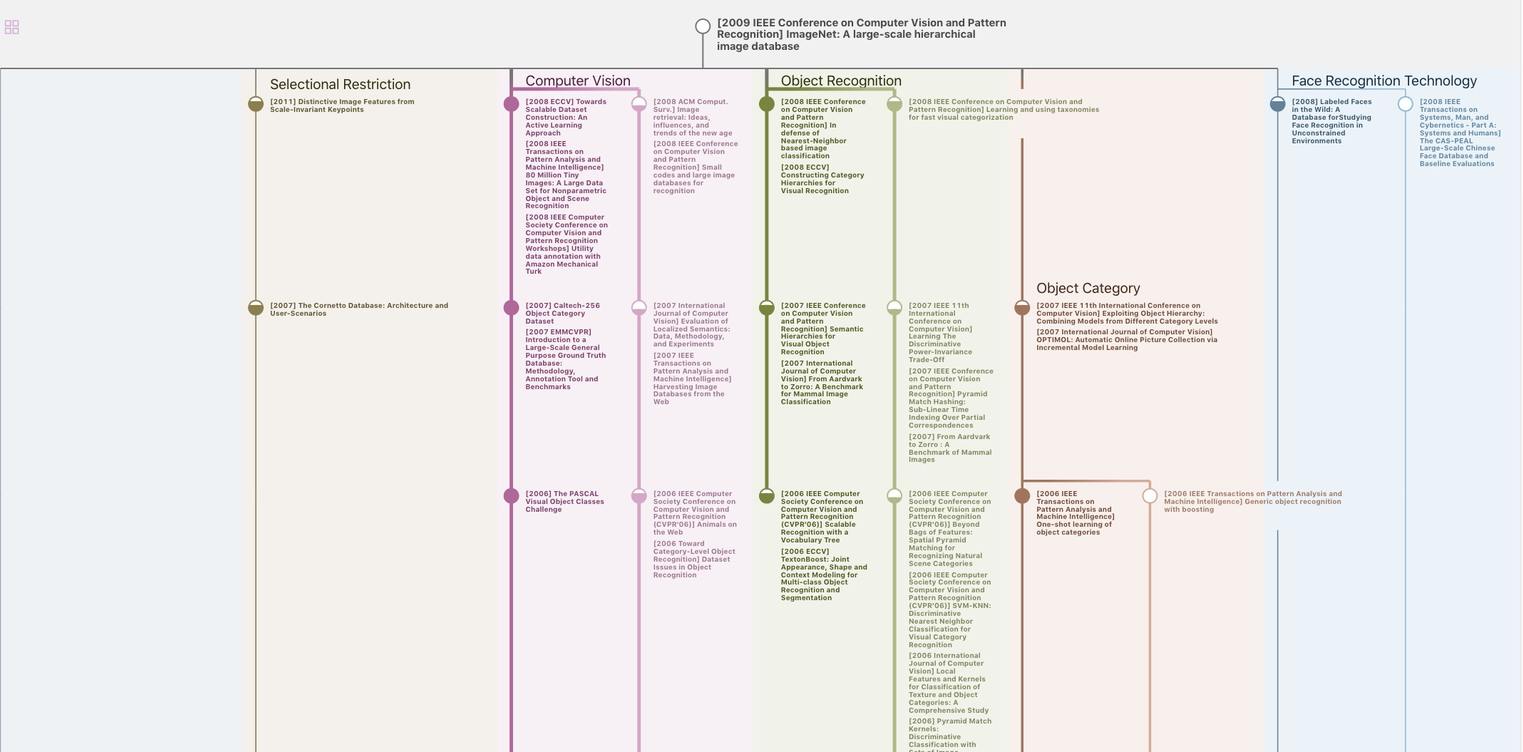
生成溯源树,研究论文发展脉络
Chat Paper
正在生成论文摘要