Implementation and Evaluation of a Machine Learned Mesoscale Eddy Parameterization into a Numerical Ocean Circulation Model
arxiv(2023)
摘要
We address the question of how to use a machine learned parameterization in a general circulation model, and assess its performance both computationally and physically. We take one particular machine learned parameterization \cite{Guillaumin1&Zanna-JAMES21} and evaluate the online performance in a different model from which it was previously tested. This parameterization is a deep convolutional network that predicts parameters for a stochastic model of subgrid momentum forcing by mesoscale eddies. We treat the parameterization as we would a conventional parameterization once implemented in the numerical model. This includes trying the parameterization in a different flow regime from that in which it was trained, at different spatial resolutions, and with other differences, all to test generalization. We assess whether tuning is possible, which is a common practice in general circulation model development. We find the parameterization, without modification or special treatment, to be stable and that the action of the parameterization to be diminishing as spatial resolution is refined. We also find some limitations of the machine learning model in implementation: 1) tuning of the outputs from the parameterization at various depths is necessary; 2) the forcing near boundaries is not predicted as well as in the open ocean; 3) the cost of the parameterization is prohibitively high on CPUs. We discuss these limitations, present some solutions to problems, and conclude that this particular ML parameterization does inject energy, and improve backscatter, as intended but it might need further refinement before we can use it in production mode in contemporary climate models.
更多查看译文
关键词
stochastic deep learning, parameterization, subgrid ocean processes, MOM6
AI 理解论文
溯源树
样例
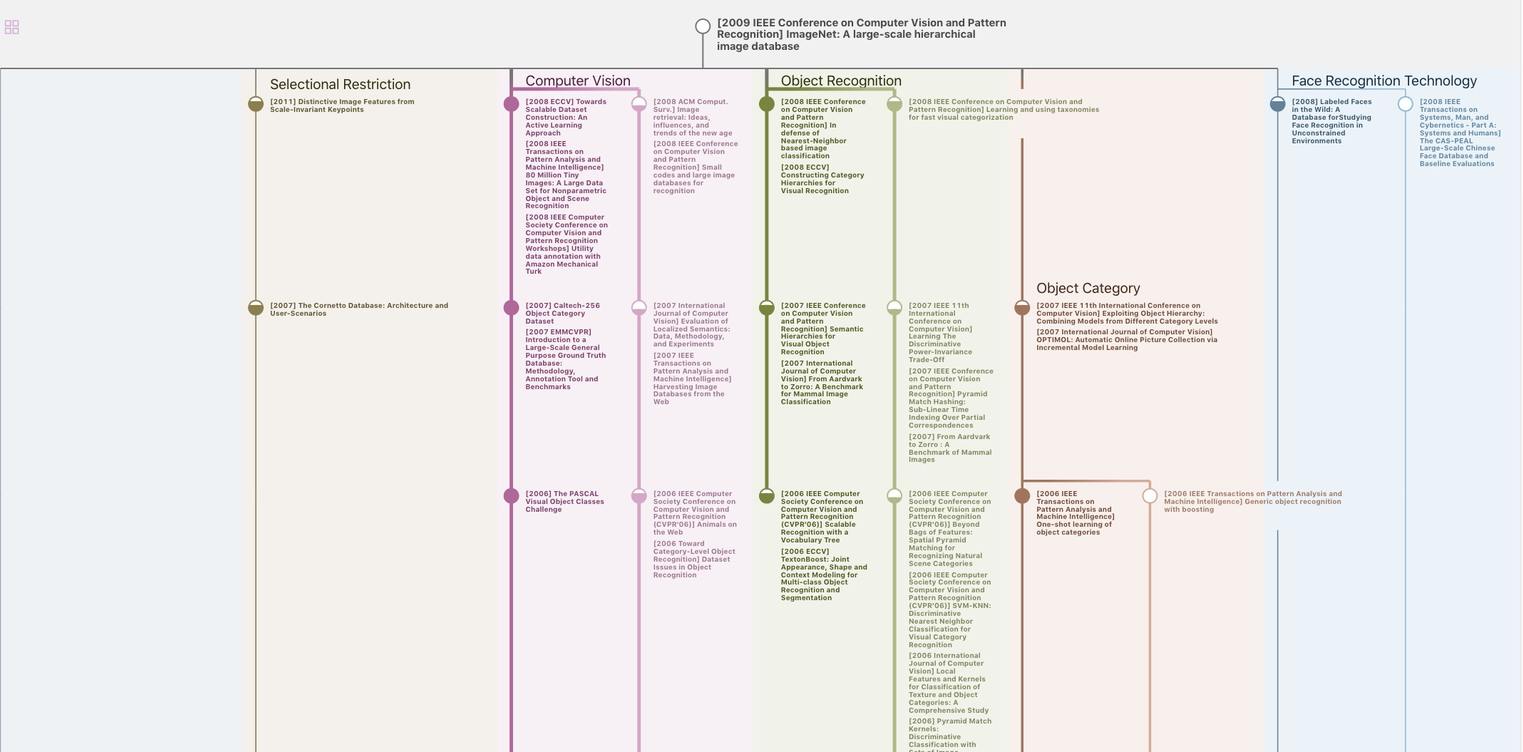
生成溯源树,研究论文发展脉络
Chat Paper
正在生成论文摘要