Almanac: Retrieval-Augmented Language Models for Clinical Medicine
Research square(2023)
摘要
Large-language models have recently demonstrated impressive zero-shot capabilities in a variety of natural language tasks such as summarization, dialogue generation, and question-answering. Despite many promising applications in clinical medicine, adoption of these models in real-world settings has been largely limited by their tendency to generate incorrect and sometimes even toxic statements. In this study, we develop Almanac, a large language model framework augmented with retrieval capabilities for medical guideline and treatment recommendations. Performance on a novel dataset of clinical scenarios (n = 130) evaluated by a panel of 5 board-certified and resident physicians demonstrates significant increases in factuality (mean of 18% at p-value < 0.05) across all specialties, with improvements in completeness and safety. Our results demonstrate the potential for large language models to be effective tools in the clinical decision-making process, while also emphasizing the importance of careful testing and deployment to mitigate their shortcomings.
更多查看译文
关键词
Topic Modeling,Clinical Event Prediction,Clinical Decision Support,Medical Concept Embedding,Medical Imaging
AI 理解论文
溯源树
样例
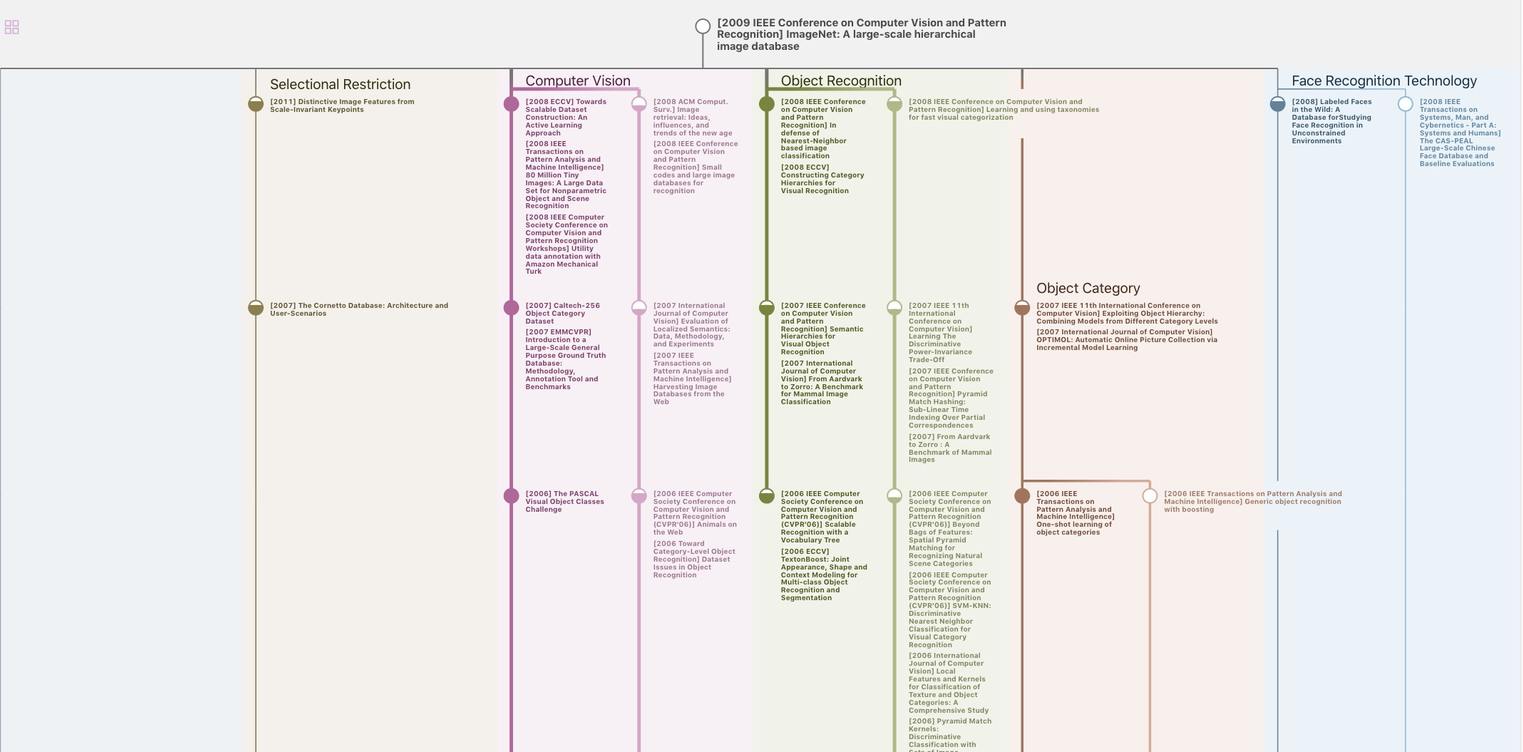
生成溯源树,研究论文发展脉络
Chat Paper
正在生成论文摘要