Penalising the biases in norm regularisation enforces sparsity
NeurIPS(2023)
摘要
Controlling the parameters' norm often yields good generalisation when training neural networks. Beyond simple intuitions, the relation between parameters' norm and obtained estimators theoretically remains misunderstood. For one hidden ReLU layer networks with unidimensional data, this work shows the minimal parameters' norm required to represent a function is given by the total variation of its second derivative, weighted by a $\sqrt{1+x^2}$ factor. As a comparison, this $\sqrt{1+x^2}$ weighting disappears when the norm of the bias terms are ignored. This additional weighting is of crucial importance, since it is shown in this work to enforce uniqueness and sparsity (in number of kinks) of the minimal norm interpolator. On the other hand, omitting the bias' norm allows for non-sparse solutions. Penalising the bias terms in the regularisation, either explicitly or implicitly, thus leads to sparse estimators. This sparsity might take part in the good generalisation of neural networks that is empirically observed.
更多查看译文
关键词
norm regularisation enforces sparsity,biases
AI 理解论文
溯源树
样例
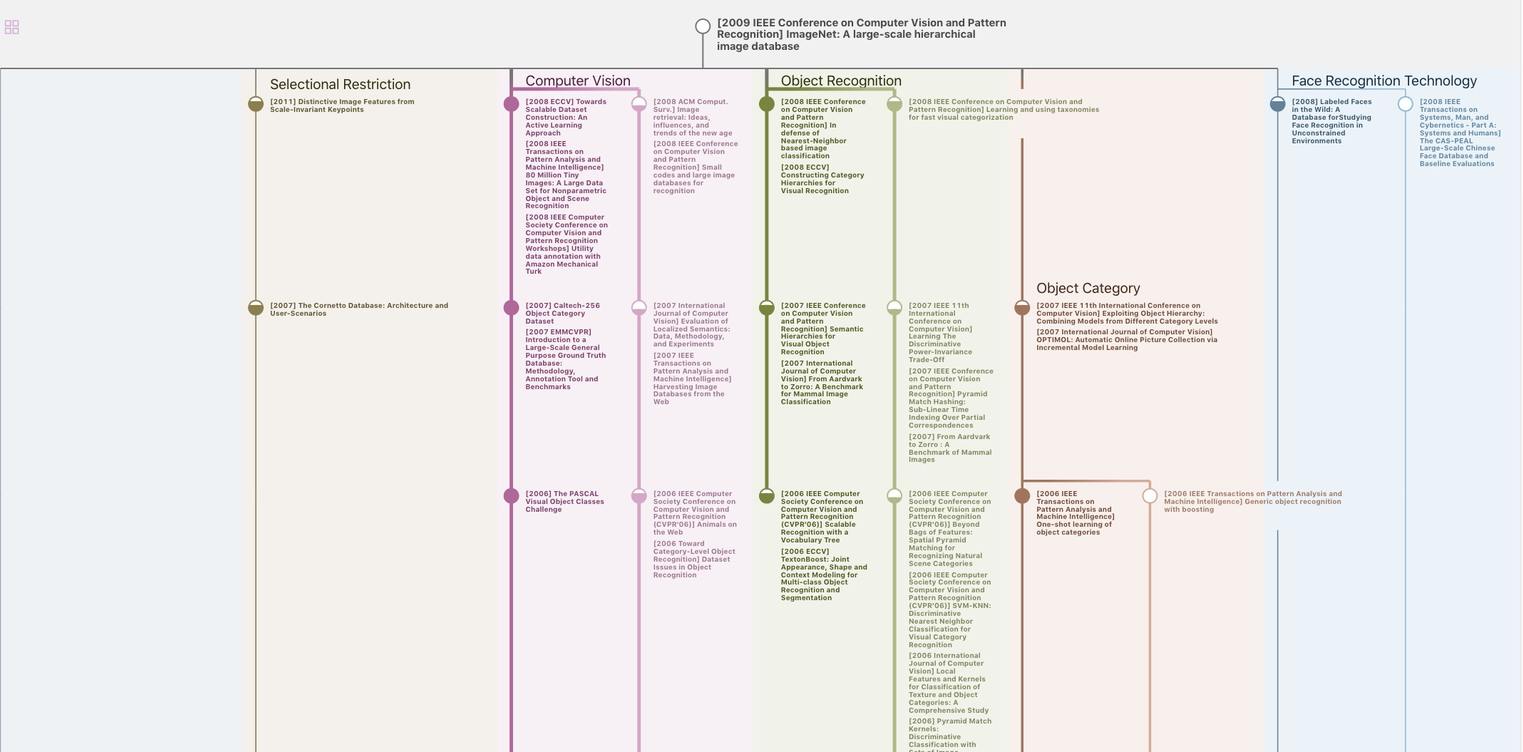
生成溯源树,研究论文发展脉络
Chat Paper
正在生成论文摘要