MRFS: Mining Rating Fraud Subgraph in Bipartite Graph for Users and Products
IEEE TRANSACTIONS ON COMPUTATIONAL SOCIAL SYSTEMS(2023)
摘要
Fraud in e-commerce fields (e.g., Amazon, Taobao, and so on) and social networks (e.g., Twitter and Weibo) has recently brought a very bad user experience. Rating fraud detection is an urgent issue for improving user experiences. However, existing methods have lots of limitations in some respects, because it is always very hard to acquire sufficient labeled data for fraud detection and detect new fraud patterns. Fortunately, the relationship for users rating (e.g., purchasing and following) products can be represented as a bipartite graph. So the problem of rating fraud detection can be transformed into the problem of abnormal subgraph detection in the bipartite graph. The major challenge of fraud detection is to distinguish fake rates from real user rates. In this article, we focus on mining rating fraud-connected subgraphs in a bipartite graph. The motivation for this work is fraud detection tasks, which can usually be formulated as mining a bipartite graph formed by source nodes (followers and users) and target nodes (followees and products) for malicious patterns. Now, smart fraudsters evade existing detection methods by buying a large pool of users and hijacking honest users, making them look "normal "-this behavior is called "camouflage. " Accordingly, we propose a fraud detection approach for mining rating fraud subgraph (MRFS), which addresses the problem from the intrinsic metric (e.g., fraudulence, badness and unreliability). The proposed MRFS mines the intrinsic characteristics of nodes and edges from node behavior information, which is an effective and scalable (linear on the input size) algorithm. A large number of comparative experimental results on real-world rating networks show that our proposed MRFS is efficient and universal.
更多查看译文
关键词
Bipartite graph,e-commerce,fraud detection,graph mining,time series
AI 理解论文
溯源树
样例
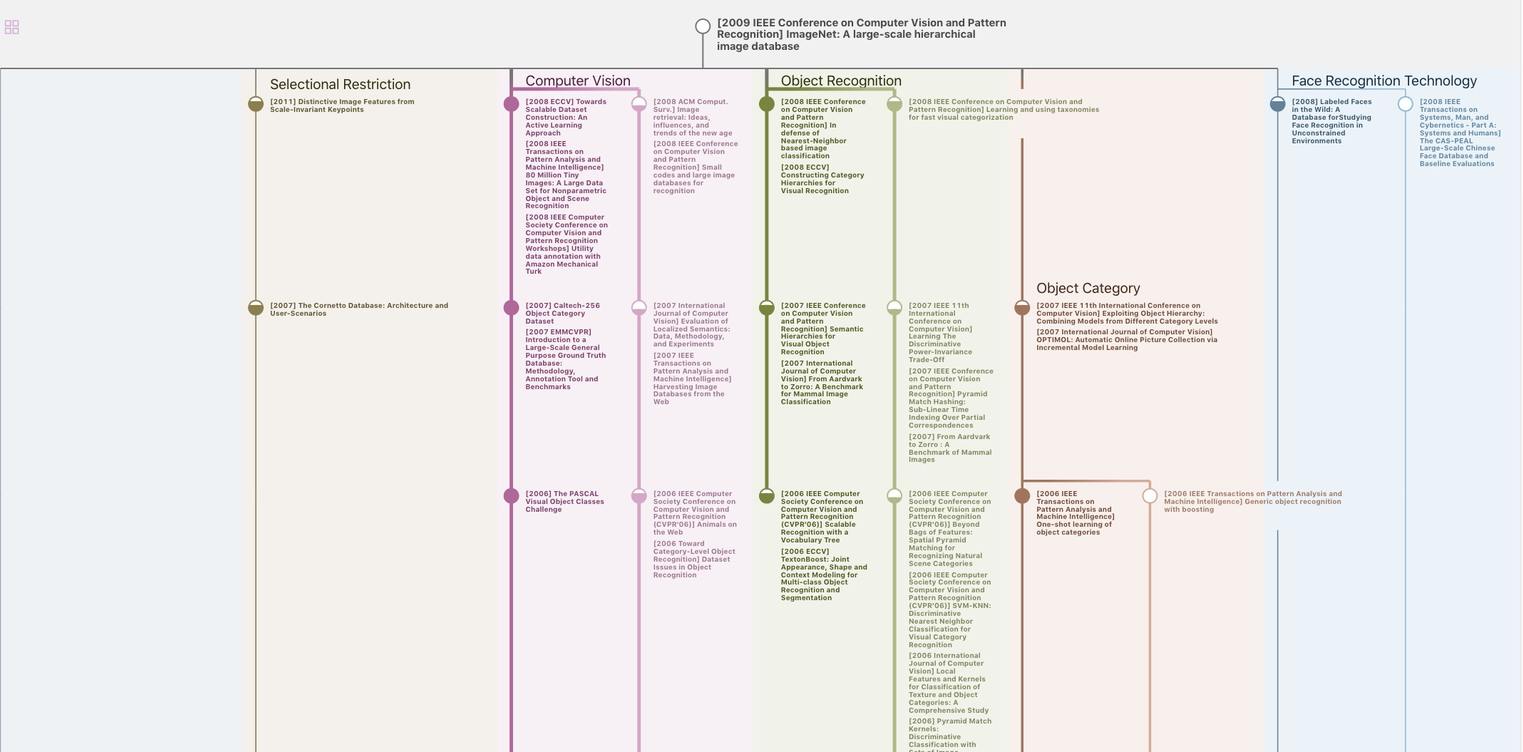
生成溯源树,研究论文发展脉络
Chat Paper
正在生成论文摘要