Gradient Learning With the Mode-Induced Loss: Consistency Analysis and Applications
IEEE TRANSACTIONS ON NEURAL NETWORKS AND LEARNING SYSTEMS(2023)
摘要
Variable selection methods aim to select the key covariates related to the response variable for learning problems with high-dimensional data. Typical methods of variable selection are formulated in terms of sparse mean regression with a parametric hypothesis class, such as linear functions or additive functions. Despite rapid progress, the existing methods depend heavily on the chosen parametric function class and are incapable of handling variable selection for problems where the data noise is heavy-tailed or skewed. To circumvent these drawbacks, we propose sparse gradient learning with the mode-induced loss (SGLML) for robust model-free (MF) variable selection. The theoretical analysis is established for SGLML on the upper bound of excess risk and the consistency of variable selection, which guarantees its ability for gradient estimation from the lens of gradient risk and informative variable identification under mild conditions. Experimental analysis on the simulated and real data demonstrates the competitive performance of our method over the previous gradient learning (GL) methods.
更多查看译文
关键词
Input variables,Estimation,Additives,Robustness,Kernel,Optimization,Learning systems,Gradient learning (GL),learning theory,mode-induced loss,Rademacher complexity,variable selection
AI 理解论文
溯源树
样例
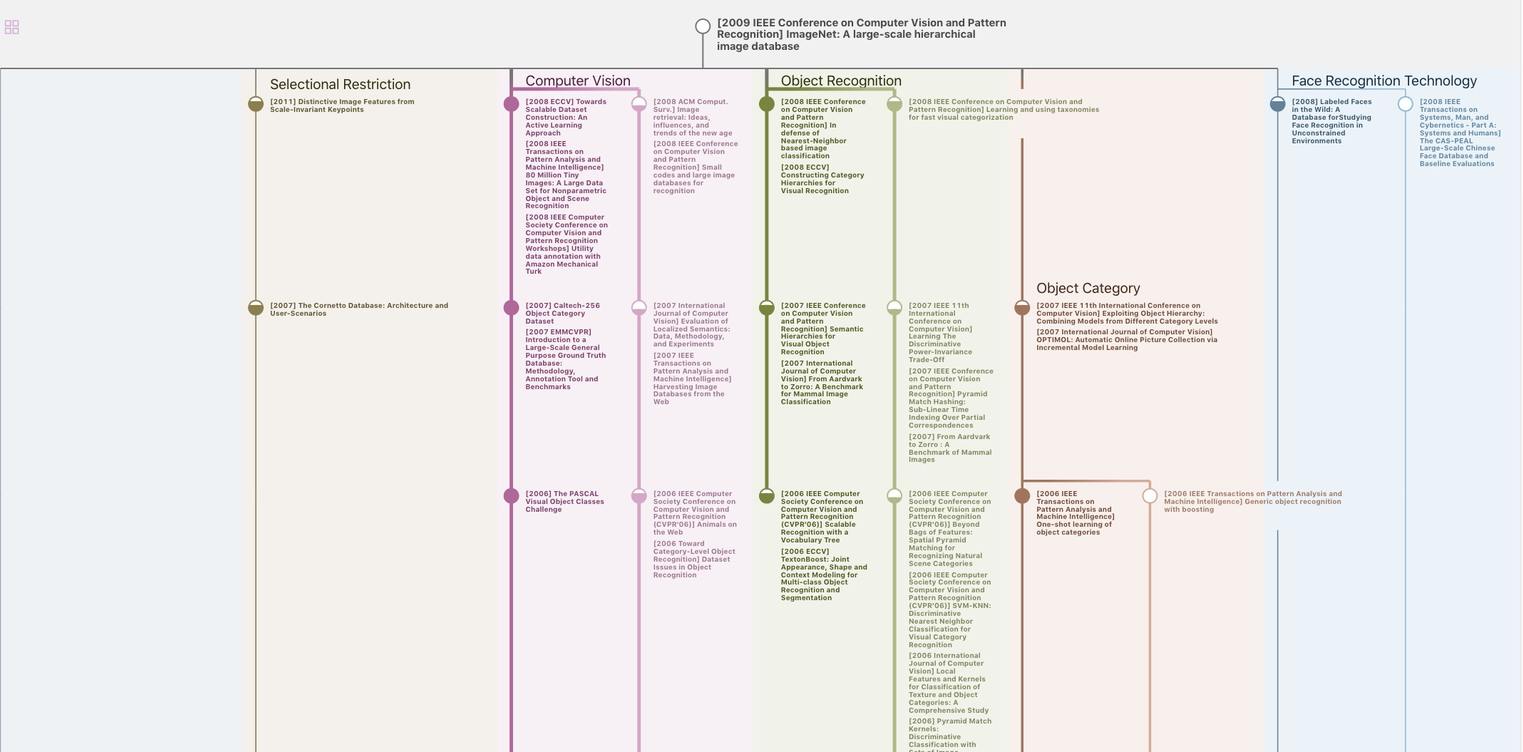
生成溯源树,研究论文发展脉络
Chat Paper
正在生成论文摘要