A study on relationship between prediction uncertainty and robustness to noisy data
INTERNATIONAL JOURNAL OF SYSTEMS SCIENCE(2023)
摘要
The presence of noise results in the decrease of the data quality, and thus affects seriously the learning performance. In this paper, we investigate theoretically and empirically how adjusting the temperature value of the softmax function to control the prediction uncertainty can help improve the model robustness to noise. Moreover, we design a modified loss function, where a regularisation term based on the uncertainty of distinguishing those non-ground truth labels is incorporated in the loss function to simultaneously improve the training accuracy and the model robustness to noise. This study theoretically and experimentally validates that the temperature parameter of the softmax function significantly impacts the classification accuracy and the proposed loss function is effective in improving the performance in the presence of noise. The study provides some practical guidelines for improving classifier performance through clearly expressing and understanding the relationship between uncertainty and noise robustness.
更多查看译文
关键词
Noisy data,uncertainty,softmax function,temperature parameter,classification accuracy
AI 理解论文
溯源树
样例
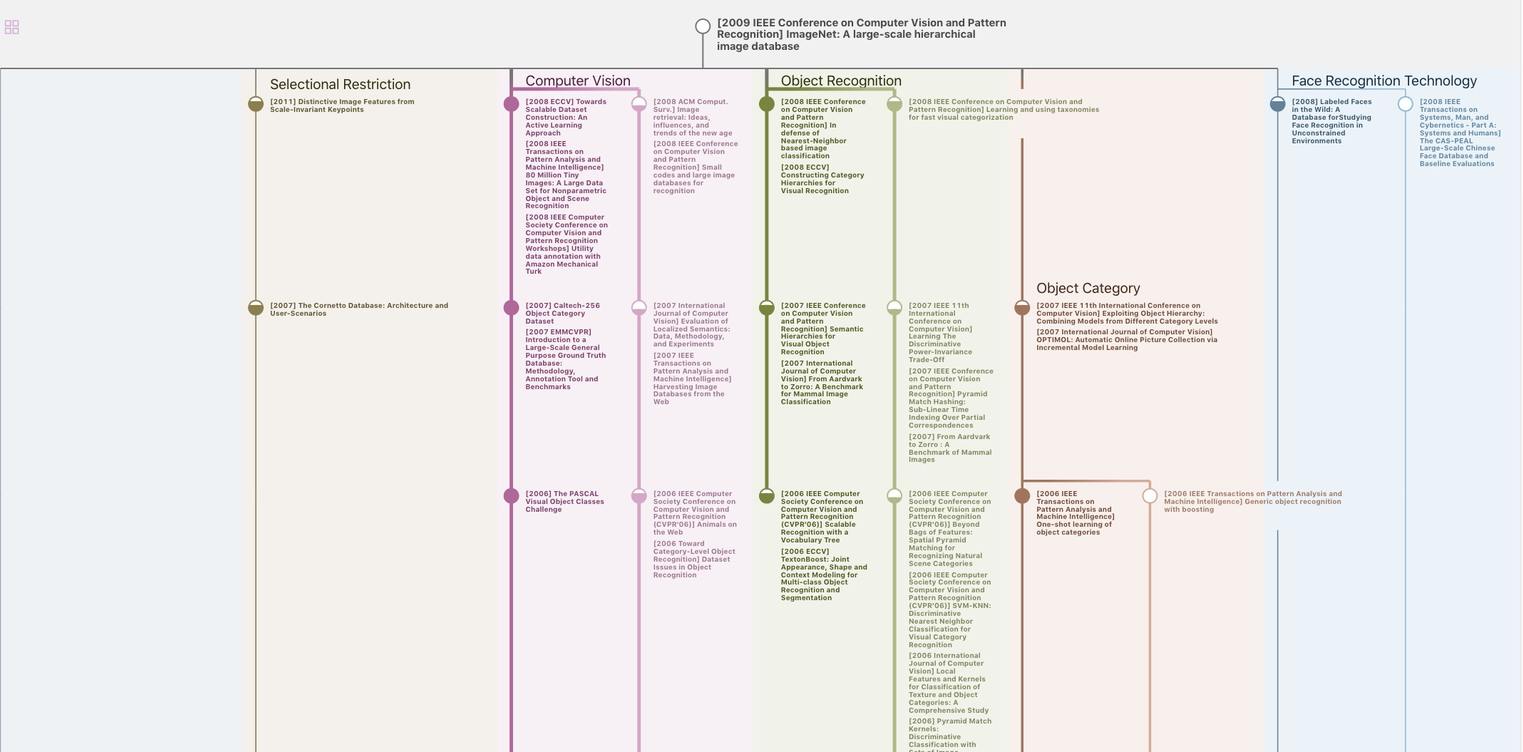
生成溯源树,研究论文发展脉络
Chat Paper
正在生成论文摘要