Metric Learning: Harnessing the Power of Machine Learning in Nanophotonics
ACS photonics(2023)
摘要
We present a novel metric-learning approach based on combined triplet loss and mean-squared error for providing more functionality (e.g., more effective similarity measures) to the machine-learning algorithms used for the knowledge discovery and inverse design of nanophotonic structures compared to commonly used mean-squared error and mean-absolute error. We demonstrate the main shortcoming of the existing metrics (or loss functions) in mapping the nanophotonic responses into lower-dimensional spaces in keeping similar responses close to each other. We show how a systematic metric-learning paradigm can resolve this issue and provide physically interpretable mappings of the nanophotonic responses while facilitating the visualization. The presented metric-learning paradigm can be combined with almost all existing machine-learning and deep-learning approaches for the investigation of nanophotonic structures. Thus, the results of this paper can have a transformative impact on using machine learning and deep learning for knowledge discovery and inverse design in nanophotonics.
更多查看译文
关键词
metric learning,similarity measure,inverse design,knowledge discovery,nanophotonics
AI 理解论文
溯源树
样例
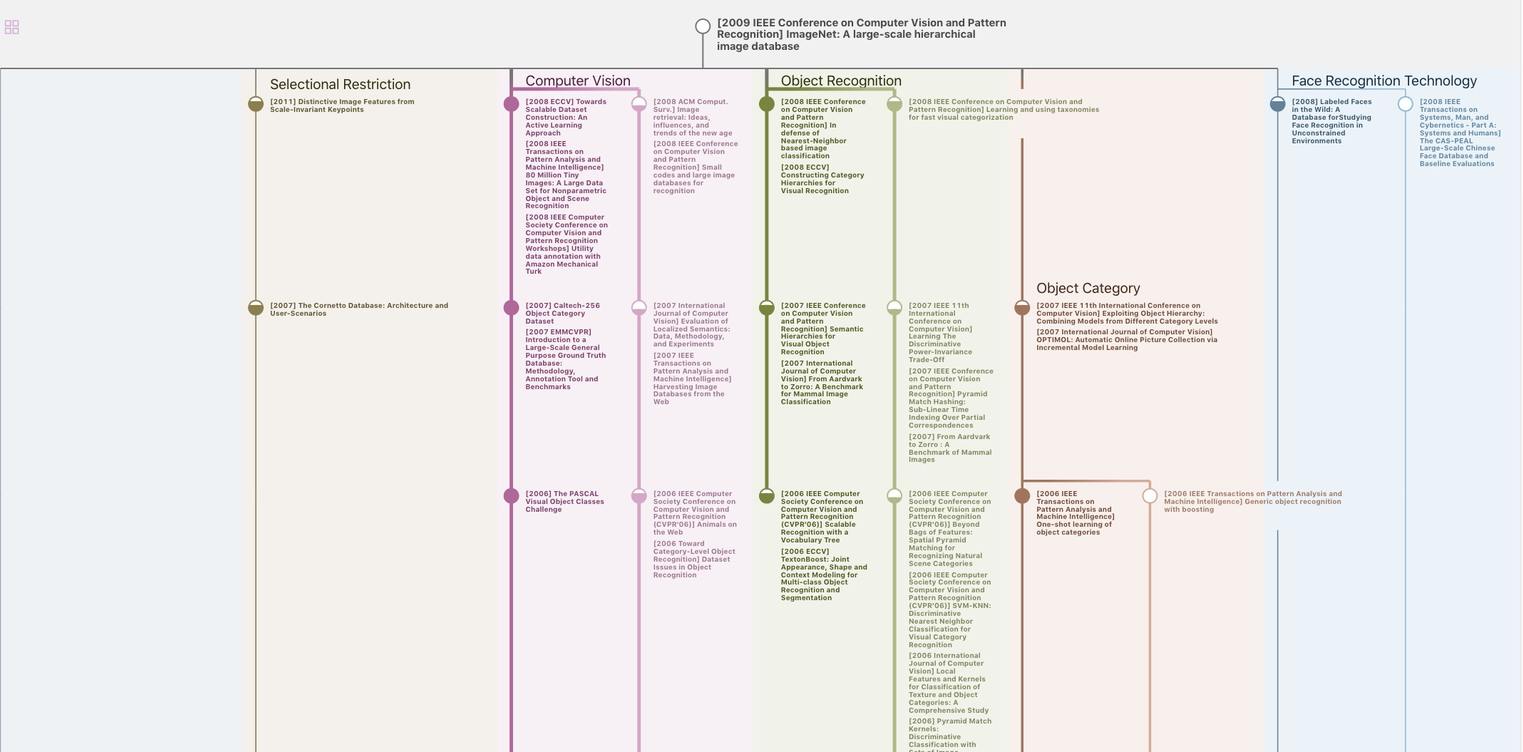
生成溯源树,研究论文发展脉络
Chat Paper
正在生成论文摘要