Toward a Brain-Inspired Theory of Artificial Learning
COGNITIVE COMPUTATION(2023)
摘要
Despite the rapid progress made by artificial neural networks (ANNs), the design of these models makes it unlikely that incremental improvements will eventually bring them on par with human-level cognitive capabilities. Here, three fundamental shortcomings of ANNs are described, namely the strictly statistical nature of the learning process, the inability to handle universal mappings, and the lack of key structural brain features that constitute the building blocks of behavior and cognition. Solutions to these issues are discussed, including the use of few-shot learning paradigms, network architectures inspired by cytoarchitectural features of the brain, and neuromodulator-derived rules for learning and updating environmental variables. We cast these solutions in the broader context of recent discoveries about the brain. Their implementation in ANNs, however, will require a deeper understanding of the cognitome — the map between elementary cognitive functions and the patterns of neural connections that support them. We contend that to reach true human-level cognitive capabilities, ANNs require both a principled approach to extending their architecture and learning rules and a deep understanding of the cognitome linking brain structures to mental operations.
更多查看译文
关键词
Artificial neural networks,One-shot learning,Neuromodulators,Universal mappings,Cognitome
AI 理解论文
溯源树
样例
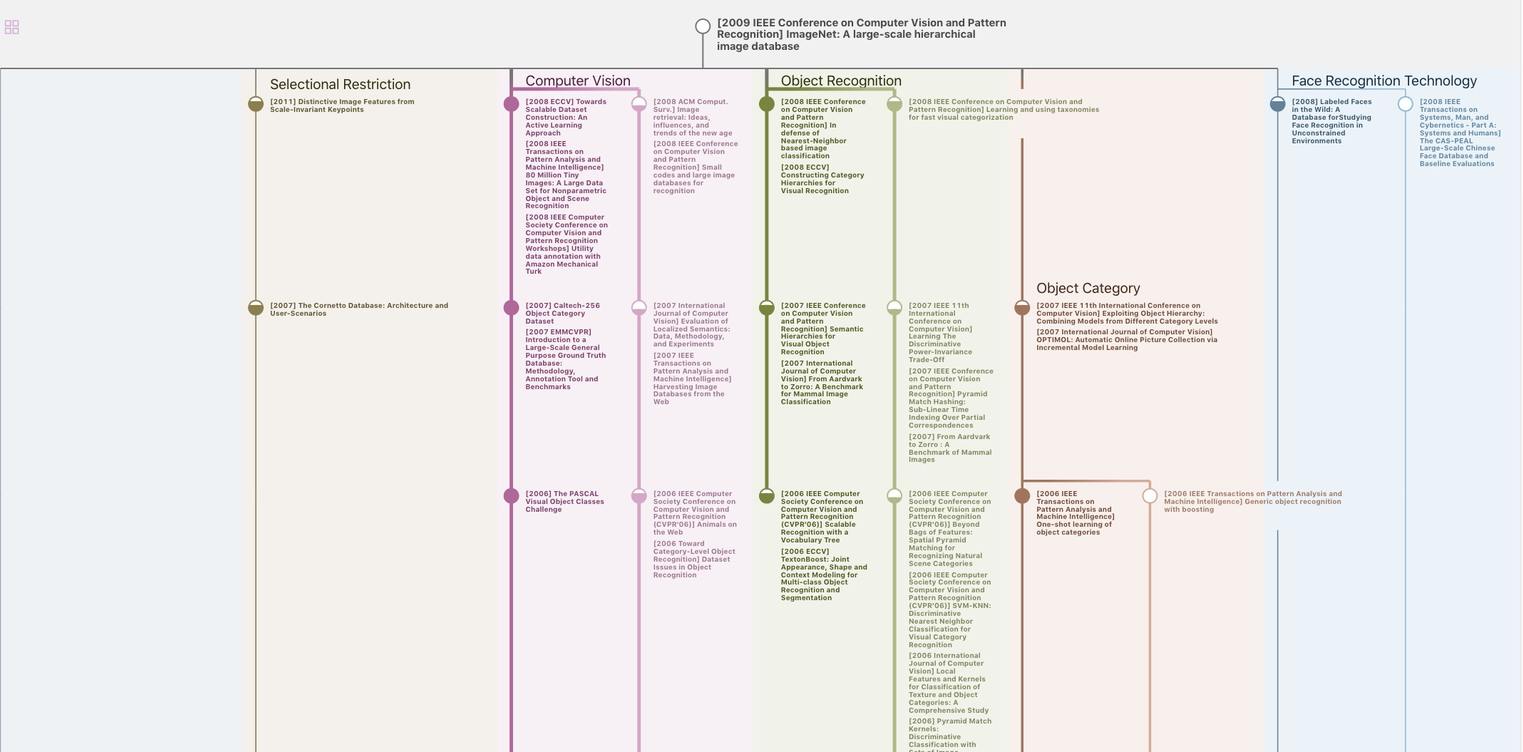
生成溯源树,研究论文发展脉络
Chat Paper
正在生成论文摘要