Data-driven modeling of multimode chemical process: Validation with a real-world distillation column
CHEMICAL ENGINEERING JOURNAL(2023)
摘要
Real-world industrial processes frequently operate in different modes such as start-up, transient, and steady-state operation. Since different operating modes are governed by different process dynamics, deriving a single data-driven model representing the entire operation of such multimode processes is not a viable option. A reasonable solution to this problem is to develop a separate model for each operating mode, which requires the extraction of data for each operating mode from raw data. Based on this viewpoint, this work develops a data-driven modeling approach using clustering and featuring selection techniques to improve the quality of raw data and develop a predictive model for a multimode industrial process. In particular, the developed method focuses on training a steady-state predictive model as monitoring steady-state conditions is crucial for achieving the desired product quality. Firstly, K-means clustering is performed to extract data describing the steady-state operation mode from the available raw data. Next, feature selection is applied to the clustered data using Pearson's correlation coefficient to identify input features relevant to target features. Finally, an LSTM model is trained using the clustered data and identified features to predict the steady-state operation. The validity and effectiveness of the developed method are demonstrated using a real-world 2,3-Butanediol distillation process dataset.
更多查看译文
关键词
LSTM,Clustering,Feature selection,Distillation,Multimode process
AI 理解论文
溯源树
样例
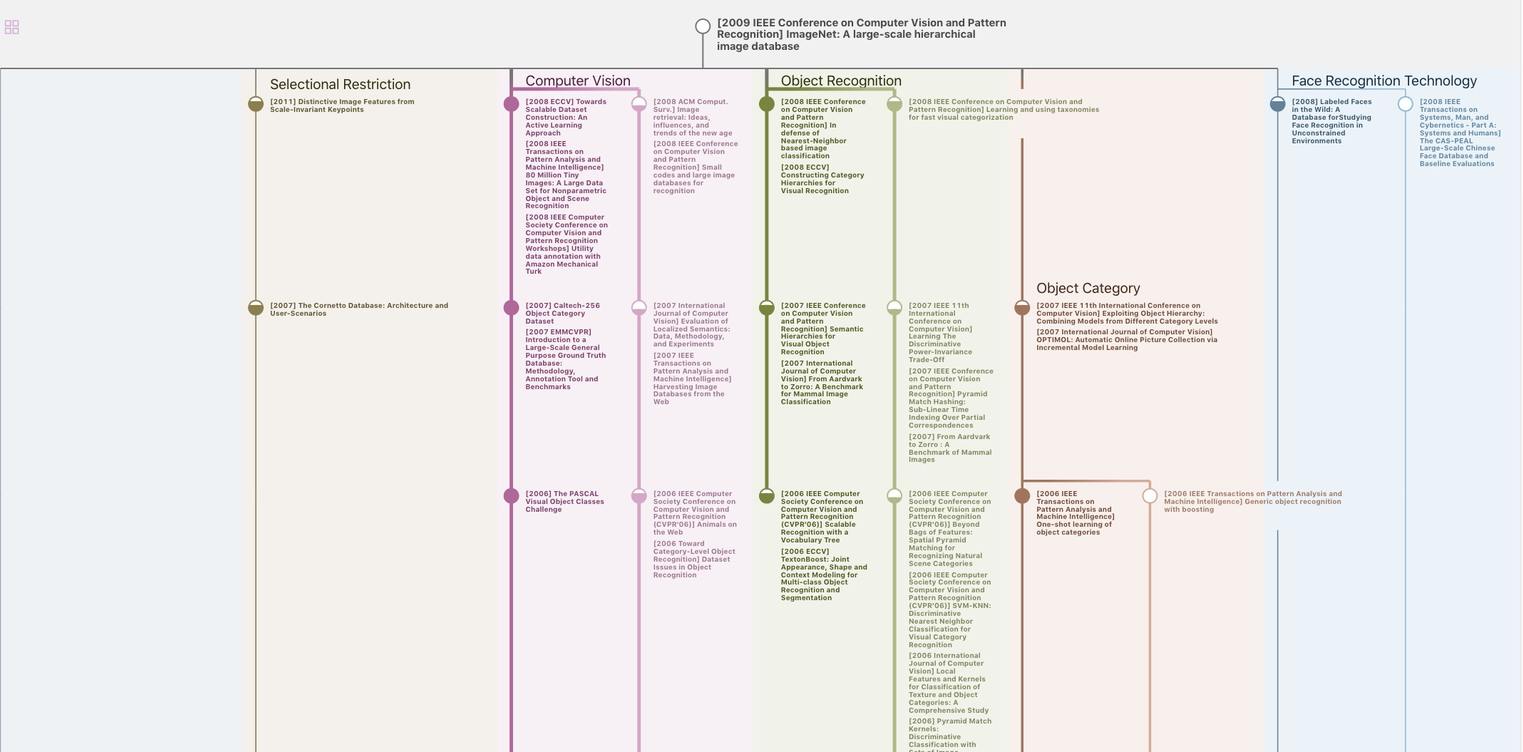
生成溯源树,研究论文发展脉络
Chat Paper
正在生成论文摘要