Data augmentation using Heuristic Masked Language Modeling
INTERNATIONAL JOURNAL OF MACHINE LEARNING AND CYBERNETICS(2023)
摘要
Data augmentation has played an important role in generalization capability and performance improvement for data-driven deep learning models in recent years. However, most of the existing data augmentation methods in NLP suffer from high manpower consumption or low promotion, which limits the practical applications. To this end, we propose a simple yet effective approach named Heuristic Masked Language Modeling(HMLM) to obtain high-quality data by introducing mask language modeling embedded in pre-trained models. More specifically, the HMLM method first identifies the core words of the sentence and masks some non-core fragments in the sentence. Then, these masked fragments will be filled with words created by the pre-trained model to match the contextual semantics. Compared with the previous data augmentation approaches, the proposed method can create more grammatical and contextual augmented data without a heavy cost. We conducted experiments on typical text classification tasks e.g., intent recognition, news classification and sentiment analysis separately. Experimental results demonstrate that our proposed method is comparable to state-of-the-art data augmentation approaches.
更多查看译文
关键词
Data augmentation,Mask language modeling,Pre-trained models,Text classification
AI 理解论文
溯源树
样例
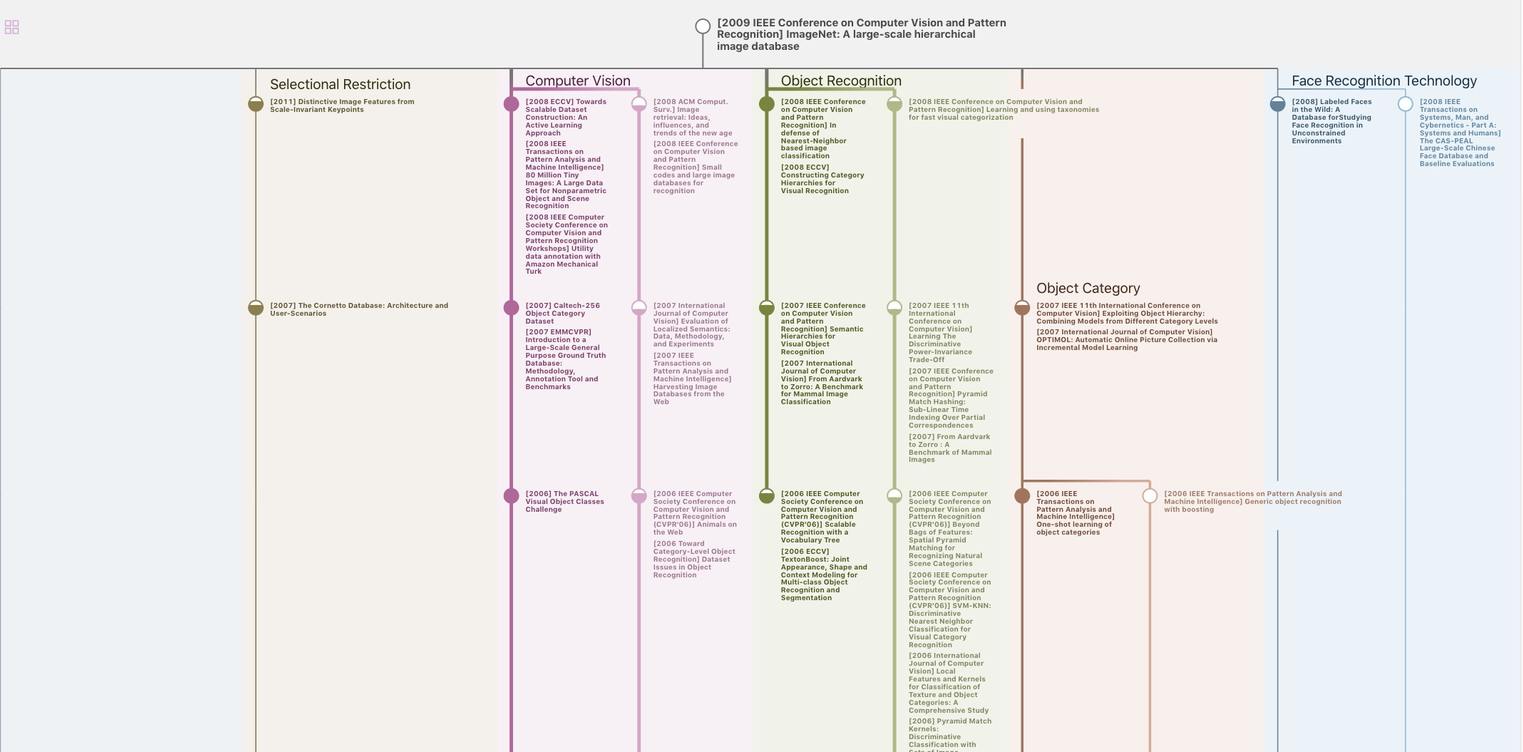
生成溯源树,研究论文发展脉络
Chat Paper
正在生成论文摘要