Global-chronological graph interactive networks for multi-domain dialogue state tracking
INTERNATIONAL JOURNAL OF MACHINE LEARNING AND CYBERNETICS(2023)
摘要
Dialogue state tracking (DST) provides necessary information for the policy learning module to decide the next action according to the historical state and the utterance related details of the dialogue, so robust dialogue state tracking is a core part of high-quality task-oriented dialogue systems. The tasks addressed by dialogue state tracking are becoming increasingly complex, and due to the multi-domain switching in the dialogue relevant contexts, the model needs to have the ability to generate appropriate responses to accurately fit the domains, slots and slot values. However, efficient encoding of state information and fundamentally simulating the logical relationship between domains and slots to dynamically extract variation features of the multiple domains are still challenges for DST. In this paper, we propose a novel framework global-chronological graph interactive networks (GCGIN), which encodes both global and chronological information of the dialogue from dual perspectives, and leverages coupling and co-affine modules to interact with the above two different granularities information. Our model adopts the mask mechanism to improve anti-noise ability, and constructs the dynamic schema graph structure to dynamically model domain-slot and domain-value relations via dialogue chronological order to achieve cross-domain features learning and improve model scalability. Extensive experimental results on three benchmark datasets demonstrate that our proposed model outperforms existing mainstream methods, and comprehensive analysis validates the effectiveness of each component.
更多查看译文
关键词
Task-oriented dialogue systems,Dialogue state tracking,Dual perspective encoding,Dynamic schema graph structure
AI 理解论文
溯源树
样例
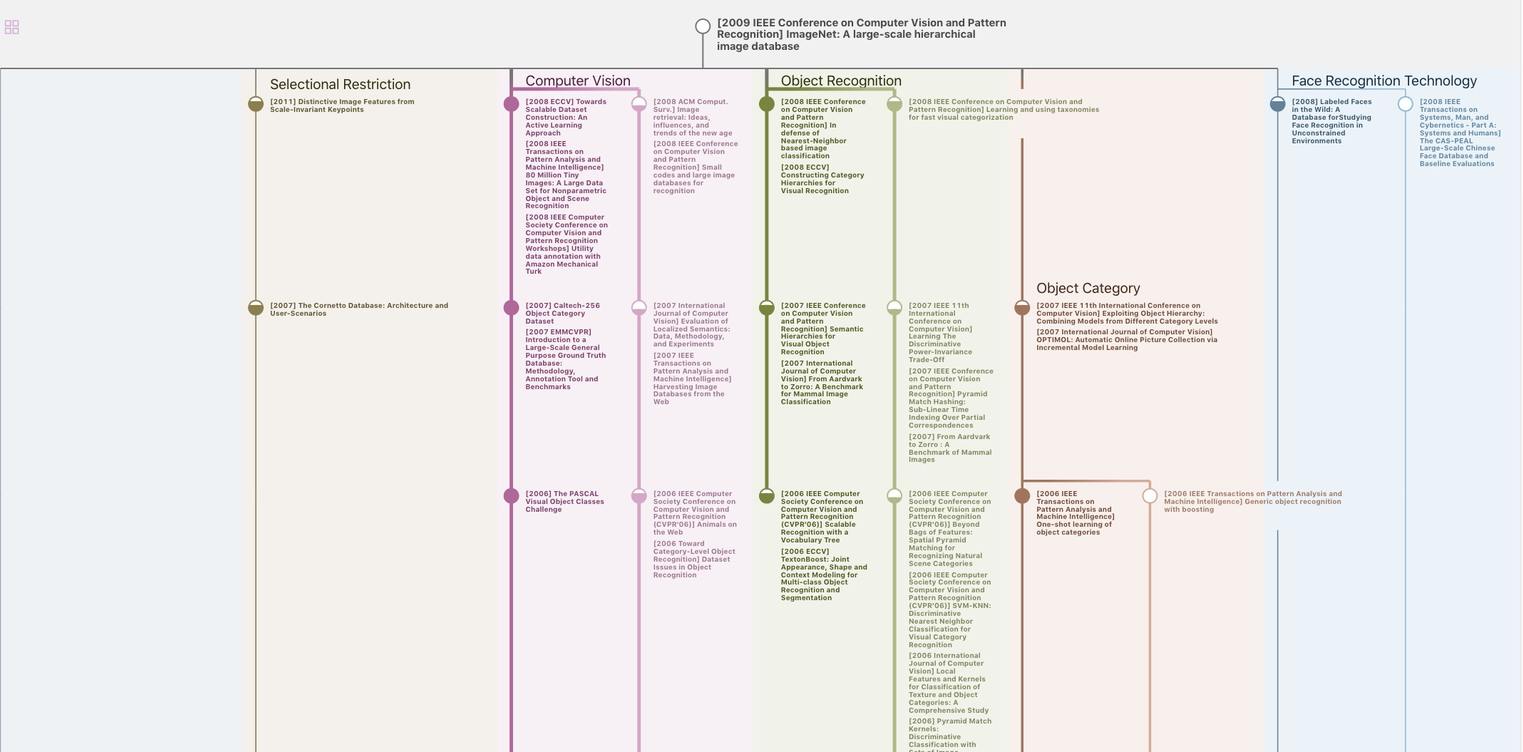
生成溯源树,研究论文发展脉络
Chat Paper
正在生成论文摘要