Spatiotemporal correlation modelling for machine learning-based traffic state predictions: state-of-the-art and beyond
TRANSPORT REVIEWS(2023)
摘要
Predicting traffic states has gained more attention because of its practical significance. However, the existing literature lacks a critical review regarding how to address the spatiotemporal correlation in the ML-based traffic state prediction models from a traffic-oriented perspective. Therefore, this study aims to comprehensively and critically review the spatiotemporal correlation modelling (STCM) approaches adopted for developing ML-based traffic state prediction models and provide future research directions based on traffic-oriented characteristics and ML techniques. Concretely, we investigate the neural network-based traffic state prediction models and characterise the STCM of these models by a proposed systematic review framework including three components: (i) spatial feature representation that demonstrates how the spatial information regarding road network is formulated, (ii) temporal feature representation that illustrates a variety of approaches to extract the temporal features, and (iii) model structure analyses the model layout to address the spatial correlations and temporal correlations simultaneously. Finally, several open challenges regarding incorporating traffic-oriented characteristics such as signal effects with ML techniques are put up with future research directions provided and discussed.
更多查看译文
关键词
Literature review,traffic state prediction,spatiotemporal correlation,neural networks,intelligent transportation systems
AI 理解论文
溯源树
样例
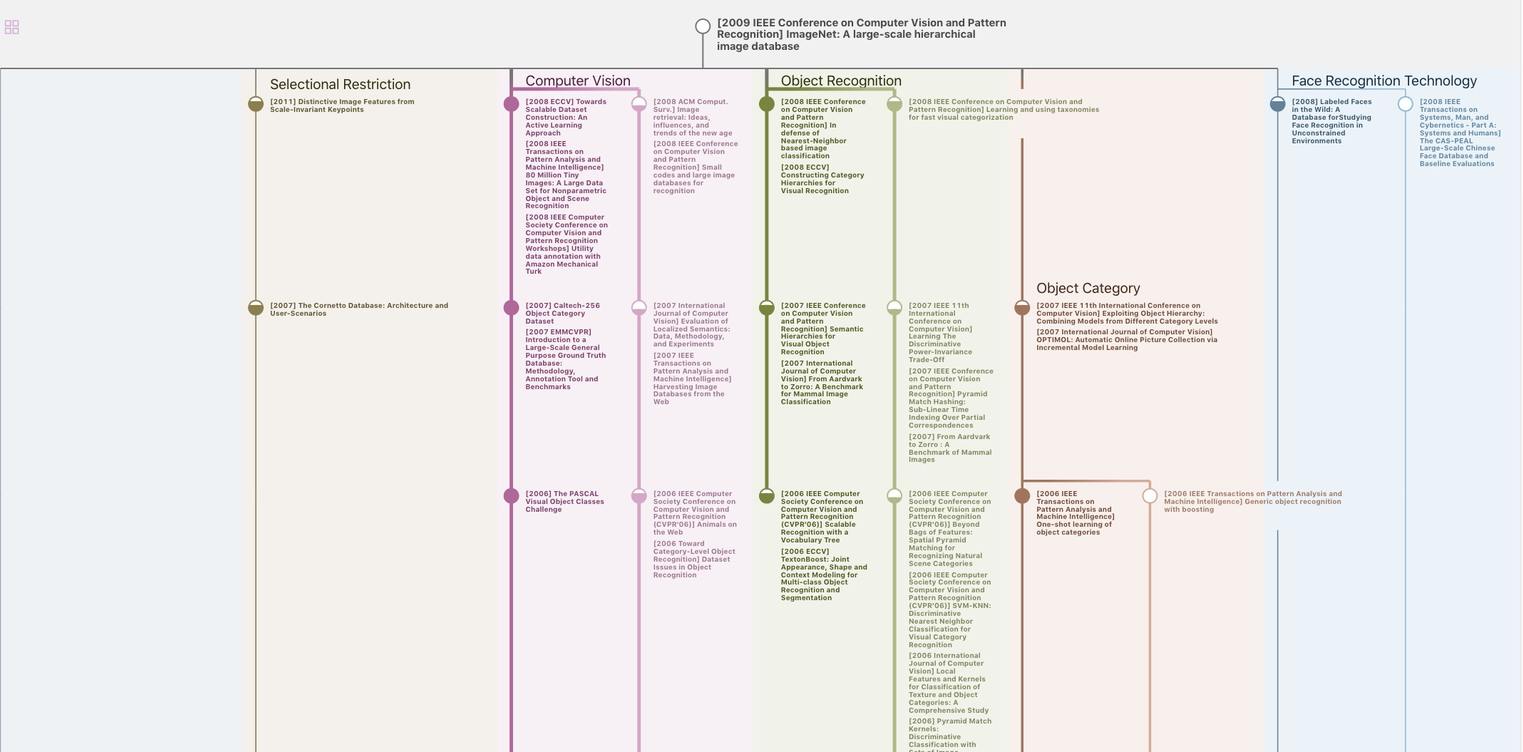
生成溯源树,研究论文发展脉络
Chat Paper
正在生成论文摘要