Geometric deep learning reveals the spatiotemporal features of microscopic motion
NATURE MACHINE INTELLIGENCE(2023)
摘要
The characterization of dynamical processes in living systems provides important clues for their mechanistic interpretation and link to biological functions. Owing to recent advances in microscopy techniques, it is now possible to routinely record the motion of cells, organelles and individual molecules at multiple spatiotemporal scales in physiological conditions. However, the automated analysis of dynamics occurring in crowded and complex environments still lags behind the acquisition of microscopic image sequences. Here we present a framework based on geometric deep learning that achieves the accurate estimation of dynamical properties in various biologically relevant scenarios. This deep-learning approach relies on a graph neural network enhanced by attention-based components. By processing object features with geometric priors, the network is capable of performing multiple tasks, from linking coordinates into trajectories to inferring local and global dynamic properties. We demonstrate the flexibility and reliability of this approach by applying it to real and simulated data corresponding to a broad range of biological experiments.
更多查看译文
关键词
geometric deep learning,spatiotemporal features,deep learning,motion
AI 理解论文
溯源树
样例
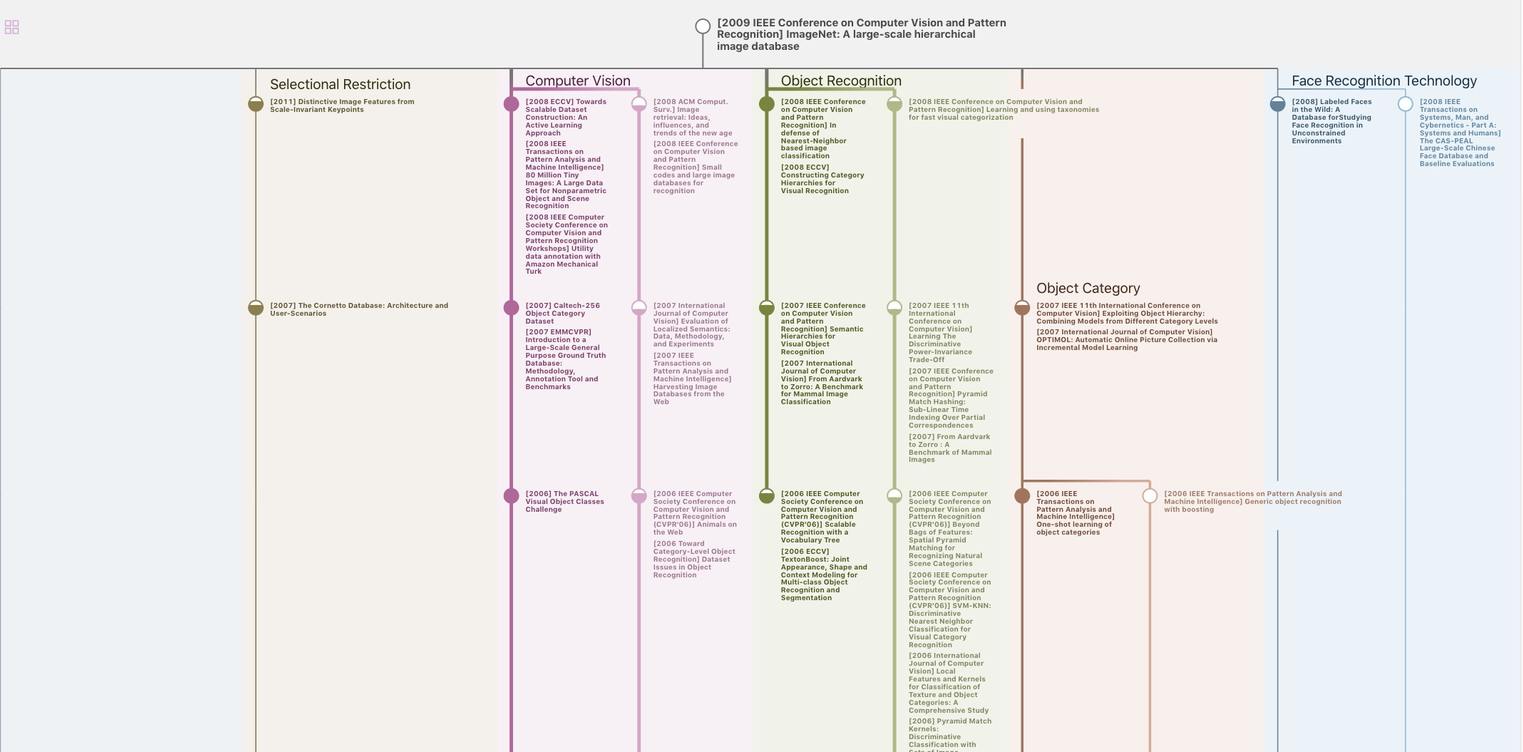
生成溯源树,研究论文发展脉络
Chat Paper
正在生成论文摘要