Invariant Extended Kalman Filtering for Tightly Coupled LiDAR-Inertial Odometry and Mapping
IEEE-ASME TRANSACTIONS ON MECHATRONICS(2023)
摘要
In this article, we extend the invariant extended Kalman filter (EKF) to light detection and ranging (LiDAR)-inertial odometry and mapping systems using invariant observer design and the theory of Lie groups for directly fusing LiDAR and inertial measurement unit (IMU) measurements. We consider this from two different aspects and implement two independent systems. Specifically, we propose a robo-centric invariant EKF LiDAR-inertial odometry termed Inv-LIO1. Its mapping module is an ordinary used one and two modules run in separate threads. A world-centric invariant EKF LiDAR-inertial odometry termed Inv-LIO2 is designed and implemented, which has an integrated odometry and mapping architecture. In Inv-LIO1, the output of the filter is the pose estimated by the scan-to-scan match method, which serves as the initial estimate of the mapping module that refines the odometry and constructs a 3-D map. The robo-centric formulation represents that the state in a local frame shifted at every LiDAR time to prevent filter divergence. Inv-LIO2 directly fuses LiDAR feature points and IMU data to obtain the map-refined odometry by scan-to-map match method, followed by global map update. To validate the effectiveness and robustness of the proposed method, we conduct extensive experiments in various indoor and outdoor environments. Overall, Inv-LIO1 offers pure odometry with higher accuracy than other state-of-the-art systems, improving the overall performance. Inv-LIO2 achieves superior accuracy over other state-of-the-art systems in the map-refined odometry comparison.
更多查看译文
关键词
Laser radar,Feature extraction,Simultaneous localization and mapping,Robustness,Point cloud compression,Optimization,Kalman filters,Invariant extended kalman filter (EKF),light detection and ranging (LiDAR)-inertial odometry,multisensor fusion localization,state estimation
AI 理解论文
溯源树
样例
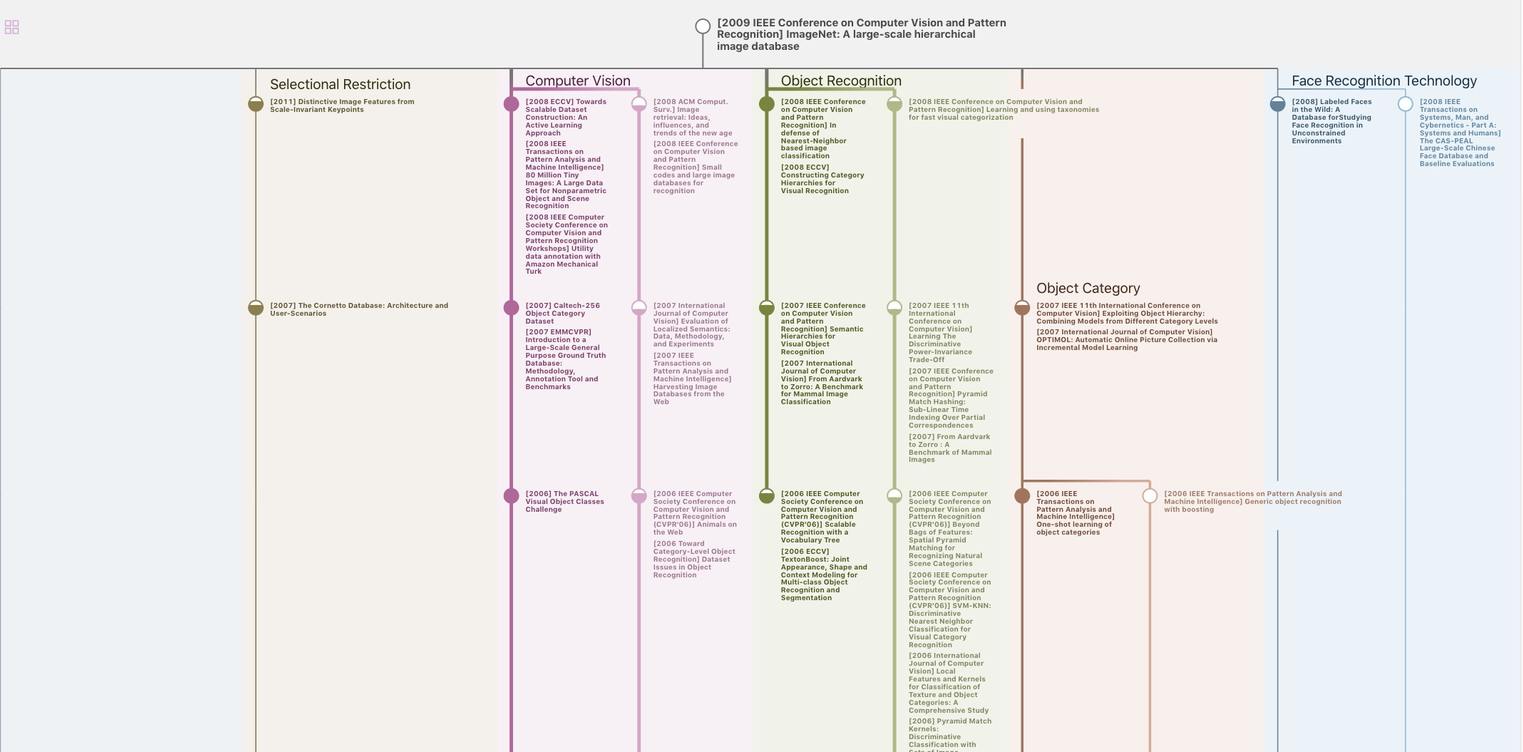
生成溯源树,研究论文发展脉络
Chat Paper
正在生成论文摘要