Dimensionality reduction using local-global standard hypergraph embedding for rotor fault diagnosis
MEASUREMENT SCIENCE AND TECHNOLOGY(2023)
摘要
The vibration signal contains a lot of state information, and its time domain, frequency domain, and time-frequency domain features are extracted to describe the rotor's operational state in detail. However, multi-domain and multi-angle feature extraction can lead to information redundancy, causing the 'dimensionality catastrophe' problem, which also makes fault classification more difficult. Dimensionality reduction (DR) is a technique that can help with this issue, but the majority of current DR algorithms are based on a simple intrinsic structure. To accurately characterize the intrinsic structure of high-dimensional fault dataset, this study proposed a novel DR algorithm that considers local information, global information, and the hypergraph model, named local-global standard hypergraph embedding (LGSHE). LGSHE redefines the hypergraph weight matrix's calculation formula and constructs the local intra-class standard hypergraph, local inter-class standard hypergraph, global intra-class standard hypergraph, and global inter-class standard hypergraph to characterize the fault data structure. LGSHE can accurately characterize the intrinsic structure of high-dimensional fault dataset and increase fault classification accuracy. The performance of LGSHE is validated on two different structures of double-span rotor experimental benches, and the results show that the algorithm can achieve good fault classification accuracy.
更多查看译文
关键词
fault diagnosis,dimensionality reduction (DR),standard hypergraph model,rotor system,local and global information
AI 理解论文
溯源树
样例
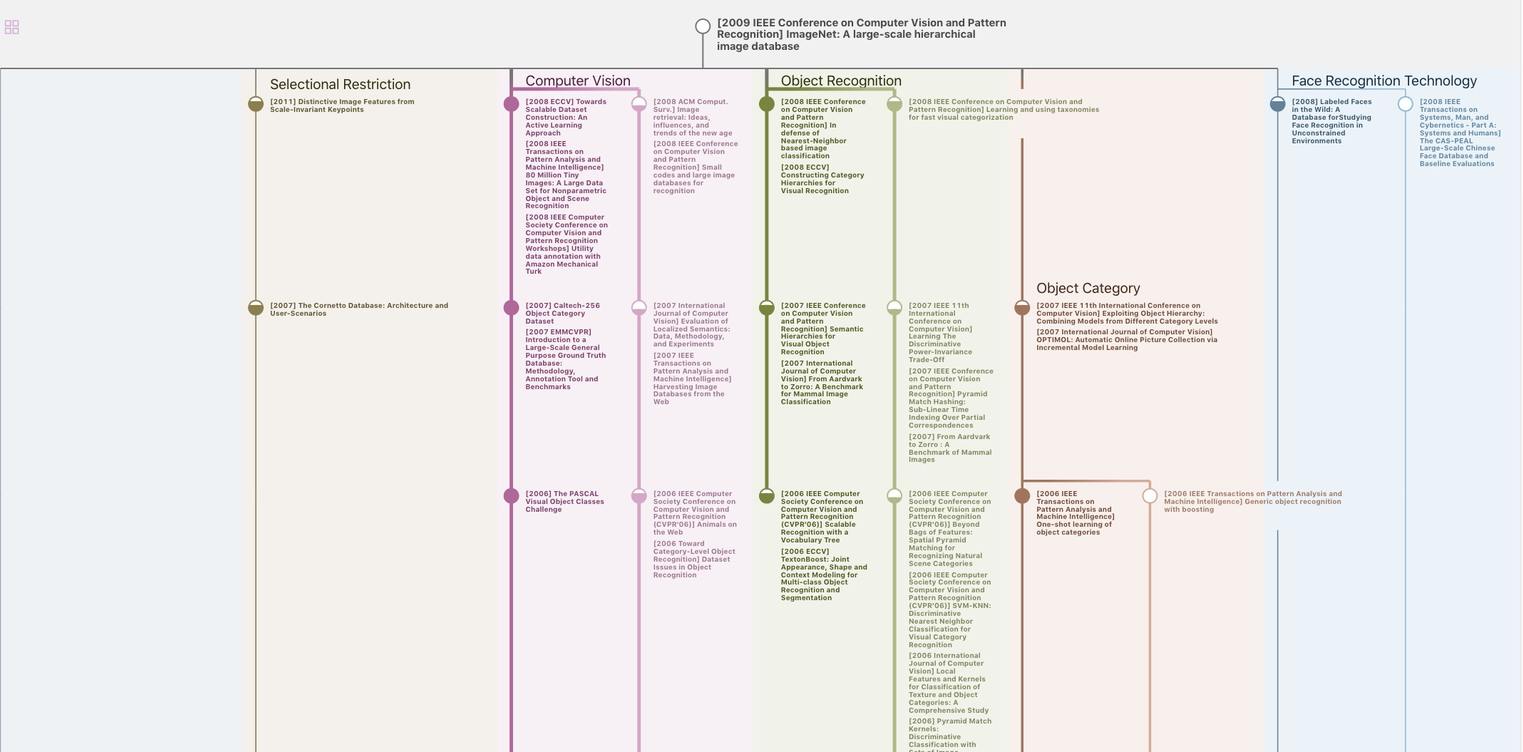
生成溯源树,研究论文发展脉络
Chat Paper
正在生成论文摘要